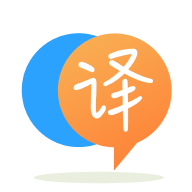
[英]python/pandas equivalent to dplyr 1.0.0 summarize(across())
[英]What is the pandas equivalent of dplyr summarize/aggregate by multiple functions?
我在從 R 過渡到dplyr
遇到了問題,其中dplyr
包可以輕松分組並執行多個匯總。
請幫助改進我現有的用於多個聚合的 Python pandas 代碼:
import pandas as pd
data = pd.DataFrame(
{'col1':[1,1,1,1,1,2,2,2,2,2],
'col2':[1,2,3,4,5,6,7,8,9,0],
'col3':[-1,-2,-3,-4,-5,-6,-7,-8,-9,0]
}
)
result = []
for k,v in data.groupby('col1'):
result.append([k, max(v['col2']), min(v['col3'])])
print pd.DataFrame(result, columns=['col1', 'col2_agg', 'col3_agg'])
問題:
for-loop groupby
實現重寫為groupby.agg
並且性能增強是巨大的)。在 R 中,等效的代碼是:
data %>% groupby(col1) %>% summarize(col2_agg=max(col2), col3_agg=min(col3))
更新:@ayhan 解決了我的問題,這是一個后續問題,我將在這里發布而不是作為評論:
Q2) groupby().summarize(newcolumn=max(col2 * col3))
的等價物是什么,即函數是 2+ 列的復合函數的聚合/匯總?
相當於
df %>% groupby(col1) %>% summarize(col2_agg=max(col2), col3_agg=min(col3))
是
df.groupby('col1').agg({'col2': 'max', 'col3': 'min'})
返回
col2 col3
col1
1 5 -5
2 9 -9
返回的對象是一個名為col1
的索引和名為col2
和col3
列的 pandas.DataFrame 。 默認情況下,當您對數據進行分組時,pandas 將分組列設置為索引以進行高效訪問和修改。 但是,如果您不想這樣,有兩種方法可以將col1
設置為列。
通過as_index=False
:
df.groupby('col1', as_index=False).agg({'col2': 'max', 'col3': 'min'})
調用reset_index
:
df.groupby('col1').agg({'col2': 'max', 'col3': 'min'}).reset_index()
兩者產量
col1 col2 col3
1 5 -5
2 9 -9
您還可以將多個函數傳遞給groupby.agg
。
agg_df = df.groupby('col1').agg({'col2': ['max', 'min', 'std'],
'col3': ['size', 'std', 'mean', 'max']})
這也返回一個 DataFrame,但現在它有一個列的 MultiIndex。
col2 col3
max min std size std mean max
col1
1 5 1 1.581139 5 1.581139 -3 -1
2 9 0 3.535534 5 3.535534 -6 0
MultiIndex 對於選擇和分組非常方便。 以下是一些示例:
agg_df['col2'] # select the second column
max min std
col1
1 5 1 1.581139
2 9 0 3.535534
agg_df[('col2', 'max')] # select the maximum of the second column
Out:
col1
1 5
2 9
Name: (col2, max), dtype: int64
agg_df.xs('max', axis=1, level=1) # select the maximum of all columns
Out:
col2 col3
col1
1 5 -1
2 9 0
早期( 版本 0.20.0之前)可以使用字典來重命名agg
調用中的列。 例如
df.groupby('col1')['col2'].agg({'max_col2': 'max'})
將第二列的最大值返回為max_col2
:
max_col2
col1
1 5
2 9
但是,它已被棄用,以支持重命名方法:
df.groupby('col1')['col2'].agg(['max']).rename(columns={'max': 'col2_max'})
col2_max
col1
1 5
2 9
對於像agg_df
定義的 agg_df 這樣的agg_df
它可能會變得冗長。 在這種情況下,您可以使用重命名函數來展平這些級別:
agg_df.columns = ['_'.join(col) for col in agg_df.columns]
col2_max col2_min col2_std col3_size col3_std col3_mean col3_max
col1
1 5 1 1.581139 5 1.581139 -3 -1
2 9 0 3.535534 5 3.535534 -6 0
對於像groupby().summarize(newcolumn=max(col2 * col3))
,您仍然可以通過首先添加一個帶有assign
的新列來使用 agg 。
df.assign(new_col=df.eval('col2 * col3')).groupby('col1').agg('max')
col2 col3 new_col
col1
1 5 -1 -1
2 9 0 0
這將為舊列和新列返回最大值,但您可以像往常一樣對其進行切片。
df.assign(new_col=df.eval('col2 * col3')).groupby('col1')['new_col'].agg('max')
col1
1 -1
2 0
Name: new_col, dtype: int64
使用groupby.apply
這會更短:
df.groupby('col1').apply(lambda x: (x.col2 * x.col3).max())
col1
1 -1
2 0
dtype: int64
但是, groupby.apply
將其視為自定義函數,因此不會對其進行矢量化。 到目前為止,我們傳遞給agg
的函數('min'、'max'、'min'、'size' 等)是矢量化的,這些是那些優化函數的別名。 您可以將df.groupby('col1').agg('min')
替換為df.groupby('col1').agg(min)
、 df.groupby('col1').agg(np.min)
或df.groupby('col1').min()
並且它們都將執行相同的函數。 當您使用自定義函數時,您不會看到同樣的效率。
最后,從 0.20 版本開始, agg
可以直接在 DataFrames 上使用,而不必先分組。 請參閱此處的示例。
在此處檢查 Pandas 文檔給出的並排比較: http : //pandas.pydata.org/pandas-docs/stable/comparison_with_r.html#grouping-and-summarizing
R的dplyr
gdf <- group_by(df, col1)
summarise(gdf, avg=mean(col1, na.rm=TRUE))
熊貓
gdf = df.groupby('col1')
df.groupby('col1').agg({'col1': 'mean'})
無需使用datar
學習datar
API,就可以很容易地將 R 代碼轉換為 python 代碼:
>>> from datar import f
>>> from datar.tibble import tibble
>>> from datar.dplyr import group_by, summarize
>>> from datar.base import min, max
>>> data = tibble(
... col1=[1,1,1,1,1,2,2,2,2,2],
... col2=[1,2,3,4,5,6,7,8,9,0],
... col3=[-1,-2,-3,-4,-5,-6,-7,-8,-9,0]
... )
>>> data >> group_by(f.col1) >> summarize(col2_agg=max(f.col2), col3_agg=min(f.col3))
col1 col2_agg col3_agg
0 1 5 -5
1 2 9 -9
我是包的作者。 如果您有任何問題,請隨時提交問題。
聲明:本站的技術帖子網頁,遵循CC BY-SA 4.0協議,如果您需要轉載,請注明本站網址或者原文地址。任何問題請咨詢:yoyou2525@163.com.