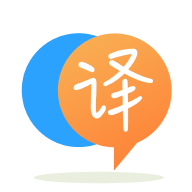
[英]Is there a way to find the average precision and recall of each class in the custom Tensorflow model?
[英]Why is the Average Precision of my TensorFlow model -1.000?
我一直在訓練的object_detection TensorFlow模型有一段時間了,但我注意到,在評估表始終顯示的-1.000一個值,而我看到其他人的輸出0和1之間顯示不同的值是否有我的TFRecords出問題了嗎? 我已經評估了輸出和后幾千步的模式肯定是提高了,所以我不知道為什么,這總是會發生的。 謝謝!
我的輸出:
Precision (AP) @[ IoU=0.50:0.95 | area= all | maxDets=100 ] = -1.000
Precision (AP) @[ IoU=0.50 | area= all | maxDets=100 ] = -1.000
Precision (AP) @[ IoU=0.75 | area= all | maxDets=100 ] = -1.000
Precision (AP) @[ IoU=0.50:0.95 | area= small | maxDets=100 ] = -1.000
Precision (AP) @[ IoU=0.50:0.95 | area=medium | maxDets=100 ] = -1.000
Precision (AP) @[ IoU=0.50:0.95 | area= large | maxDets=100 ] = -1.000
Recall (AR) @[ IoU=0.50:0.95 | area= all | maxDets= 1 ] = -1.000
Recall (AR) @[ IoU=0.50:0.95 | area= all | maxDets= 10 ] = -1.000
Recall (AR) @[ IoU=0.50:0.95 | area= all | maxDets=100 ] = -1.000
Recall (AR) @[ IoU=0.50:0.95 | area= small | maxDets=100 ] = -1.000
Recall (AR) @[ IoU=0.50:0.95 | area=medium | maxDets=100 ] = -1.000
Recall (AR) @[ IoU=0.50:0.95 | area= large | maxDets=100 ] = -1.000
我的pipeline.config文件:
model {
ssd {
num_classes: 2
image_resizer {
fixed_shape_resizer {
height: 300
width: 300
}
}
feature_extractor {
type: "ssd_mobilenet_v1"
depth_multiplier: 1.0
min_depth: 16
conv_hyperparams {
regularizer {
l2_regularizer {
weight: 3.99999989895e-05
}
}
initializer {
truncated_normal_initializer {
mean: 0.0
stddev: 0.0299999993294
}
}
activation: RELU_6
batch_norm {
decay: 0.999700009823
center: true
scale: true
epsilon: 0.0010000000475
train: true
}
}
}
box_coder {
faster_rcnn_box_coder {
y_scale: 10.0
x_scale: 10.0
height_scale: 5.0
width_scale: 5.0
}
}
matcher {
argmax_matcher {
matched_threshold: 0.5
unmatched_threshold: 0.5
ignore_thresholds: false
negatives_lower_than_unmatched: true
force_match_for_each_row: true
}
}
similarity_calculator {
iou_similarity {
}
}
box_predictor {
convolutional_box_predictor {
conv_hyperparams {
regularizer {
l2_regularizer {
weight: 3.99999989895e-05
}
}
initializer {
truncated_normal_initializer {
mean: 0.0
stddev: 0.0299999993294
}
}
activation: RELU_6
batch_norm {
decay: 0.999700009823
center: true
scale: true
epsilon: 0.0010000000475
train: true
}
}
min_depth: 0
max_depth: 0
num_layers_before_predictor: 0
use_dropout: false
dropout_keep_probability: 0.800000011921
kernel_size: 1
box_code_size: 4
apply_sigmoid_to_scores: false
}
}
anchor_generator {
ssd_anchor_generator {
num_layers: 6
min_scale: 0.20000000298
max_scale: 0.949999988079
aspect_ratios: 1.0
aspect_ratios: 2.0
aspect_ratios: 0.5
aspect_ratios: 3.0
aspect_ratios: 0.333299994469
}
}
post_processing {
batch_non_max_suppression {
score_threshold: 0.300000011921
iou_threshold: 0.600000023842
max_detections_per_class: 100
max_total_detections: 100
}
score_converter: SIGMOID
}
normalize_loss_by_num_matches: true
loss {
localization_loss {
weighted_smooth_l1 {
}
}
classification_loss {
weighted_sigmoid {
}
}
hard_example_miner {
num_hard_examples: 3000
iou_threshold: 0.990000009537
loss_type: CLASSIFICATION
max_negatives_per_positive: 3
min_negatives_per_image: 0
}
classification_weight: 1.0
localization_weight: 1.0
}
}
}
train_config {
batch_size: 24
data_augmentation_options {
random_horizontal_flip {
}
}
data_augmentation_options {
ssd_random_crop {
}
}
optimizer {
rms_prop_optimizer {
learning_rate {
exponential_decay_learning_rate {
initial_learning_rate: 0.00400000018999
decay_steps: 800720
decay_factor: 0.949999988079
}
}
momentum_optimizer_value: 0.899999976158
decay: 0.899999976158
epsilon: 1.0
}
}
fine_tune_checkpoint: "pretrained_model/model.ckpt"
from_detection_checkpoint: true
num_steps: 300
}
train_input_reader {
label_map_path: "./CMFCD/pascal_label_map.pbtxt"
tf_record_input_reader {
input_path: "./CMFCD/data/annotations/train.record"
}
}
eval_config {
num_examples: 47
max_evals: 10
use_moving_averages: false
}
eval_input_reader {
label_map_path: "./CMFCD/pascal_label_map.pbtxt"
shuffle: false
num_readers: 1
tf_record_input_reader {
input_path: "./CMFCD/data/annotations/test.record"
}
}
編輯-解決方案:這是因為我用來生成TFRecords的腳本已過時,並創建了沒有邊界框,只有圖像的空記錄。 我找到了更新腳本和使用的,現在工作得很好。
通常,這意味着檢測器不會產生任何有意義的置信度得分的檢測結果(所有檢測結果的置信度為零),因此在評估AP時沒有要評估的內容,並且COCO API評估代碼返回-1.0
聲明:本站的技術帖子網頁,遵循CC BY-SA 4.0協議,如果您需要轉載,請注明本站網址或者原文地址。任何問題請咨詢:yoyou2525@163.com.