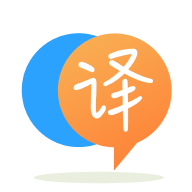
[英]Keras Custom loss function to pass arguments other than y_true and y_pred
[英]How do you gather the elements of y_pred that do not correspond to the true label in a Keras/tf2.0 custom loss function?
下面是我想做的 numpy 中的一個簡單示例:
import numpy as np
y_true = np.array([0,0,1])
y_pred = np.array([0.1,0.2,0.7])
yc = (1-y_true).astype('bool')
desired = y_pred[yc]
>>> desired
>>> array([0.1, 0.2])
所以ground truth對應的預測是0.7,我想對一個包含y_pred所有元素的數組進行操作,除了ground truth元素。
我不確定如何在 Keras 中進行這項工作。 這是損失函數中問題的一個工作示例。 現在 'desired' 沒有完成任何事情,但這就是我需要處理的:
# using tensorflow 2.0.0 and keras 2.3.1
import tensorflow.keras.backend as K
import tensorflow as tf
from tensorflow.keras.layers import Input,Dense,Flatten
from tensorflow.keras.models import Model
from keras.datasets import mnist
(x_train, y_train), (x_test, y_test) = mnist.load_data()
# Normalize data.
x_train = x_train.astype('float32') / 255
x_test = x_test.astype('float32') / 255
# Convert class vectors to binary class matrices.
y_train = tf.keras.utils.to_categorical(y_train, 10)
y_test = tf.keras.utils.to_categorical(y_test, 10)
input_shape = x_train.shape[1:]
x_in = Input((input_shape))
x = Flatten()(x_in)
x = Dense(256,'relu')(x)
x = Dense(256,'relu')(x)
x = Dense(256,'relu')(x)
out = Dense(10,'softmax')(x)
def loss(y_true,y_pred):
yc = tf.math.logical_not(kb.cast(y_true, 'bool'))
desired = tf.boolean_mask(y_pred,yc,axis = 1) #Remove and it runs
CE = tf.keras.losses.categorical_crossentropy(
y_true,
y_pred)
L = CE
return L
model = Model(x_in,out)
model.compile('adam',loss = loss,metrics = ['accuracy'])
model.fit(x_train,y_train)
我最終得到一個錯誤
ValueError: Shapes (10,) and (None, None) are incompatible
其中 10 是類別數。 最終目的是實現這一點:Keras 中的ComplementEntropy ,我的問題似乎是第 26-28 行。
您可以從Boolean_mask
刪除axis=1
並且它會運行。 坦率地說,我不明白為什么你需要axis=1。
def loss(y_true,y_pred):
yc = tf.math.logical_not(K.cast(y_true, 'bool'))
print(yc.shape)
desired = tf.boolean_mask(y_pred, yc) #Remove axis=1 and it runs
CE = tf.keras.losses.categorical_crossentropy(
y_true,
y_pred)
L = CE
return L
這大概就是會發生的事情。 你有y_pred
這是一個二維張量( N=2
)。 然后你有一個 2D 面具( K=2
)。 但是有這個條件K + axis <= N
。 如果您通過axis=1
這將失敗。
使用 sohv89 的答案,這里是我如何在參考論文中在 LeNet 上實現COT的完整代碼。 一個技巧是我實際上並沒有在兩個目標之間來回翻轉,而是只有一個隨機權重可以翻轉s
。
# using tensorflow 2.0.0 and keras 2.3.1
import tensorflow.keras.backend as kb
import tensorflow as tf
from tensorflow.keras.layers import Conv2D, Input, Dense,Flatten,AveragePooling2D,GlobalAveragePooling2D
from tensorflow.keras.models import Model
from keras.datasets import mnist
(x_train, y_train), (x_test, y_test) = mnist.load_data()
# Normalize data.
x_train = x_train.astype('float32') / 255
x_test = x_test.astype('float32') / 255
#exapnd dims to fit chn format
x_train = np.expand_dims(x_train,axis=3)
x_test = np.expand_dims(x_test,axis=3)
# Convert class vectors to binary class matrices.
y_train = tf.keras.utils.to_categorical(y_train, 10)
y_test = tf.keras.utils.to_categorical(y_test, 10)
input_shape = x_train.shape[1:]
x_in = Input((input_shape))
act = 'tanh'
x = Conv2D(32, (5, 5), activation=act, padding='same',strides = 1)(x_in)
x = AveragePooling2D((2, 2),strides = (2,2))(x)
x = Conv2D(16, (5, 5), activation=act)(x)
x = AveragePooling2D((2, 2),strides = (2,2))(x)
conv_out = Flatten()(x)
z = Dense(120,activation = act)(conv_out)#120
z = Dense(84,activation = act)(z)#84
last = Dense(10,activation = 'softmax')(z)
model = Model(x_in,last)
def loss(y_true,y_pred, axis=-1):
s = kb.round(tf.random.uniform( (1,), minval=0, maxval=1, dtype=tf.dtypes.float32))
s_ = 1 - s
y_pred = y_pred + 1e-8
yg = kb.max(y_pred,axis=1)
yc = tf.math.logical_not(kb.cast(y_true, 'bool'))
yp_c = tf.boolean_mask(y_pred, yc)
ygc_ = 1/(1-yg+1e-8)
ygc_ = kb.expand_dims(ygc_,axis=1)
Px = yp_c*ygc_ +1e-8
COT = kb.mean(Px*kb.log(Px),axis=1)
CE = -kb.mean(y_true*kb.log(y_pred),axis=1)
L = s*CE +s_*(1/(10-1))*COT
return L
model.compile(loss=loss,
optimizer='adam', metrics=['accuracy'])
model.fit(x_train,y_train,epochs=20,batch_size = 128,validation_data= (x_test,y_test))
pred = model.predict(x_test)
pred_label = np.argmax(pred,axis=1)
label = np.argmax(y_test,axis=1)
cor = (pred_label == label).sum()
acc = print('acc:',cor/label.shape[0])
聲明:本站的技術帖子網頁,遵循CC BY-SA 4.0協議,如果您需要轉載,請注明本站網址或者原文地址。任何問題請咨詢:yoyou2525@163.com.