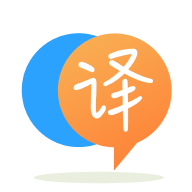
[英]How to preserve dict keys in tf.data.Dataset.from_generator?
[英]How to speed up tf.data.Dataset.from_generator()
在 tensorflow2.0 中,我想訓練一個具有 nce 損失的 skip-gram 模型。 tf.data.Dataset.from_tensor_slices() 不適合,因為輸入文件真的很大。 所以我寫了一個這樣的數據集生成器類:
class DataSet:
""""""
def __init__(self, args, vocab):
self.args = args
self.vocab = vocab
def generator(self):
"""a generator function, it will return skip-gram sample or cbow sample"""
with open(self.args.input) as f_input:
for line in tqdm.tqdm(f_input.readlines()):
tokens = line.strip().split()
tokens_indices = self.vocab.indices(tokens)
for index, target_word in enumerate(tokens_indices):
context_words = list()
begin = index - self.args.window_size if index - self.args.window_size > 0 else 0
end = index + 1 + self.args.window_size if index + self.args.window_size + 1 < len(tokens_indices) else len(
tokens_indices)
context_words.extend(tokens_indices[begin:index])
context_words.extend(tokens_indices[index + 1:end])
if self.args.cbow > 0:
yield context_words, target_word
else:
for i in range(len(context_words)):
yield target_word, context_words[i]
def dataset(self):
"""Using tf.data.Dataset.from_generator() to return sample"""
if self.args.cbow:
dataset = tf.data.Dataset.from_generator(
self.generator,
(tf.int32, tf.int32),
(tf.TensorShape([None]), tf.TensorShape([]))
)
else:
dataset = tf.data.Dataset.from_generator(
self.generator,
(tf.int32, tf.int32),
(tf.TensorShape([]), tf.TensorShape([]))
)
return dataset
然后我用以下方法測試我的代碼:
dataset = DataSet(args, vocab).dataset()
iterator = dataset.make_one_shot_iterator()
for batch, (x,y) in enumerate(dataset.batch(128)):
pass
print(batch, x.shape, y.shape)
但是迭代所有行需要花費大量時間(在 MacBook pro 2012 中大約 10 分鍾/15000 行)。 有沒有什么方法可以加速代碼?
如果您正在處理大型數據集,那么 TFRecord 是合適的選擇。 它使用二進制文件格式來存儲您的數據,並且會對導入管道的性能產生重大影響,從而對模型的訓練時間產生重大影響。 二進制數據占用更少的磁盤空間,復制所需的時間更少,並且可以更有效地從磁盤讀取。 如果您的數據存儲在旋轉磁盤上,則尤其如此,因為與 SSD 相比讀/寫性能要低得多。
然而,純粹的性能並不是 TFRecord 文件格式的唯一優勢。 它以多種方式針對 Tensorflow 進行了優化。 首先,它可以輕松組合多個數據集,並與庫提供的數據導入和預處理功能無縫集成。 特別是對於太大而無法完全存儲在內存中的數據集,這是一個優勢,因為只有當時需要的數據(例如批處理)從磁盤加載然后進行處理。 TFRecords 的另一個主要優點是可以存儲序列數據——例如,時間序列或單詞編碼——以允許非常有效和(從編碼角度)方便地導入此類數據的方式。
建議通過此官方鏈接查看TFRecord 。 您也可以通過此鏈接了解如何構建 TFRecord 管道。
下面是一個簡單的例子,使用TFRecordWriter
寫入序列化記錄,然后將其加載到TFRecordDatset
%tensorflow_version 2.x
import tensorflow as tf
print(tf.__version__)
def write_date_tfrecord():
#writes 10 dummy values to replicate the issue
Output = [20191221 + x for x in range(0,10)]
print("Writing Output - ", Output)
example = tf.train.Example(
features = tf.train.Features(
feature = {
'Output':tf.train.Feature(float_list=tf.train.FloatList(value=Output))
}
))
writer = tf.io.TFRecordWriter("Output.tf_record")
writer.write(example.SerializeToString())
def parse_function(serialized_example):
features = {
'Output': tf.io.FixedLenSequenceFeature([], tf.float32,allow_missing=True)
}
features = tf.io.parse_single_example(serialized=serialized_example, features=features)
Output = features['Output']
return Output
def dataset_generator():
trRecordDataset = tf.data.TFRecordDataset("Output.tf_record")
trRecordDataset = trRecordDataset.map(parse_function, num_parallel_calls = tf.data.experimental.AUTOTUNE)
return trRecordDataset
if __name__ == '__main__':
write_date_tfrecord()
generator = dataset_generator()
for Output in generator:
print(Output)
輸出 -
2.2.0
Writing Output - [20191221, 20191222, 20191223, 20191224, 20191225, 20191226, 20191227, 20191228, 20191229, 20191230]
tf.Tensor(
[20191220. 20191222. 20191224. 20191224. 20191224. 20191226. 20191228.
20191228. 20191228. 20191230.], shape=(10,), dtype=float32)
希望這能回答你的問題。 快樂學習。
聲明:本站的技術帖子網頁,遵循CC BY-SA 4.0協議,如果您需要轉載,請注明本站網址或者原文地址。任何問題請咨詢:yoyou2525@163.com.