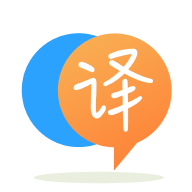
[英]pandas - Update only specific DataFrame column values based on a 'key' value
[英]Update cell values of specific columns based on condition of column value pandas
考虑以下df...。如果['Catalogue']=='Equity'
,我想将所有数据复制到['Catalogue','Display','Shelves','Price','触发条件的['周']中的“机械师”]。
在下面的示例中,['Catalogue'] =='Equity'出现在['Week'] =='1'中,因此在这种情况下,我想复制[[Catalogue],'Display', 'Shelves','Price','Mechanic'] which happens to be 'Equity','Tactical,0.0,NaN,0.5 for all rows where ['Week']== '1'
) which happens to be 'Equity','Tactical,0.0,NaN,0.5 for all rows where ['Week']== '1'
。
然后,我想在['Mechanic']*['RRP']
['Price']
中进行计算
示例数据集如下
Product Name Year Customer Week RRP Catalogue Display Shelves Price Mechanic
0 product1 2016 Customer1 1 6.99 EQUITY Tactical 0.0 NaN 0.5
1 product2 2016 Customer1 1 3.49 NaN NaN NaN NaN NaN
2 product3 2016 Customer1 1 3.49 NaN NaN NaN NaN NaN
3 product1 2016 Customer1 2 6.99 NaN NaN NaN NaN NaN
4 product2 2016 Customer1 2 3.49 NaN NaN NaN NaN NaN
5 product3 2016 Customer1 2 3.49 NaN NaN NaN NaN NaN
6 product1 2016 Customer1 3 6.99 NaN Shelf NaN 2.44 3 for 2
7 product2 2016 Customer1 3 3.49 NaN Shelf NaN 3.28 3 for 2
8 product3 2016 Customer1 3 3.49 NaN Shelf NaN 1.97 3 for 2
9 product1 2016 Customer1 4 6.99 NaN Shelf NaN 2.44 3 for 2
10 product2 2016 Customer1 4 3.49 NaN Shelf NaN 3.28 3 for 2
11 product3 2016 Customer1 4 3.49 NaN Shelf NaN 1.97 3 for 2
12 product1 2016 Customer1 5 6.99 NaN NaN NaN NaN NaN
13 product2 2016 Customer1 5 3.49 NaN NaN NaN NaN NaN
14 product3 2016 Customer1 5 3.49 NaN NaN NaN NaN NaN
我想要的输出如下...
Product Name Year Customer Week RRP Catalogue Display Shelves Price Mechanic
0 product1 2016 Customer1 1 6.99 EQUITY Tactical 0.0 3.50 0.5
1 product2 2016 Customer1 1 3.49 EQUITY Tactical 0.0 1.74 0.5
2 product3 2016 Customer1 1 3.49 EQUITY Tactical 0.0 1.74 0.5
3 product1 2016 Customer1 2 6.99 NaN NaN NaN NaN NaN
4 product2 2016 Customer1 2 3.49 NaN NaN NaN NaN NaN
5 product3 2016 Customer1 2 3.49 NaN NaN NaN NaN NaN
6 product1 2016 Customer1 3 6.99 NaN Shelf NaN 2.44 3 for 2
7 product2 2016 Customer1 3 3.49 NaN Shelf NaN 3.28 3 for 2
8 product3 2016 Customer1 3 3.49 NaN Shelf NaN 1.97 3 for 2
9 product1 2016 Customer1 4 6.99 NaN Shelf NaN 2.44 3 for 2
10 product2 2016 Customer1 4 3.49 NaN Shelf NaN 3.28 3 for 2
11 product3 2016 Customer1 4 3.49 NaN Shelf NaN 1.97 3 for 2
12 product1 2016 Customer1 5 6.99 NaN NaN NaN NaN NaN
13 product2 2016 Customer1 5 3.49 NaN NaN NaN NaN NaN
14 product3 2016 Customer1 5 3.49 NaN NaN NaN NaN NaN
有人可以帮忙吗?
采用:
cols = ['Catalogue','Display','Shelves','Price','Mechanic']
m1 = df['Catalogue']=='EQUITY'
#boolean mask for all Weeks contains at least one EQUITY in column Catalogue
m2 = df['Week'].isin(df.loc[m1, 'Week'])
#filter df and for week forward and then back filling missing values
df[m2] = df[m2].groupby('Week').ffill().groupby('Week').bfill()
#convert column to numeric
mech = pd.to_numeric(df.loc[m2, 'Mechanic'], errors='coerce')
#multiple filtered columns
df.loc[m2, 'Price'] = (df.loc[m2, 'RRP'] * mech).round(2)
#for temporary display for disable representation of dataframes to stretch across pages
with pd.option_context('display.expand_frame_repr', False):
print (df)
Product Name Year Customer Week RRP Catalogue Display Shelves Price Mechanic
0 product1 2016 Customer1 1 6.99 EQUITY Tactical 0.0 3.50 0.5
1 product2 2016 Customer1 1 3.49 EQUITY Tactical 0.0 1.74 0.5
2 product3 2016 Customer1 1 3.49 EQUITY Tactical 0.0 1.74 0.5
3 product1 2016 Customer1 2 6.99 NaN NaN NaN NaN NaN
4 product2 2016 Customer1 2 3.49 NaN NaN NaN NaN NaN
5 product3 2016 Customer1 2 3.49 NaN NaN NaN NaN NaN
6 product1 2016 Customer1 3 6.99 NaN Shelf NaN 2.44 3 for 2
7 product2 2016 Customer1 3 3.49 NaN Shelf NaN 3.28 3 for 2
8 product3 2016 Customer1 3 3.49 NaN Shelf NaN 1.97 3 for 2
9 product1 2016 Customer1 4 6.99 NaN Shelf NaN 2.44 3 for 2
10 product2 2016 Customer1 4 3.49 NaN Shelf NaN 3.28 3 for 2
11 product3 2016 Customer1 4 3.49 NaN Shelf NaN 1.97 3 for 2
12 product1 2016 Customer1 5 6.99 NaN NaN NaN NaN NaN
13 product2 2016 Customer1 5 3.49 NaN NaN NaN NaN NaN
14 product3 2016 Customer1 5 3.49 NaN NaN NaN NaN NaN
声明:本站的技术帖子网页,遵循CC BY-SA 4.0协议,如果您需要转载,请注明本站网址或者原文地址。任何问题请咨询:yoyou2525@163.com.