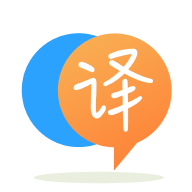
[英]Why i am getting "terminate called after throwing an instance of 'std::bad_alloc'' when my training data is large?
[英]Tensorflow MNIST: terminate called after throwing an instance of 'std::bad_alloc'
我正在嘗試使用默認的MNIST數據集在Tensorflow上實現卷積神經網絡。
from __future__ import print_function
import tensorflow as tf
from tensorflow.examples.tutorials.mnist import input_data
mnist = input_data.read_data_sets('MNIST_data', one_hot=True)
def compute_accuracy(v_xs, v_ys):
global prediction
y_pre = sess.run(prediction, feed_dict={xs: v_xs, keep_prob: 1})
correct_prediction = tf.equal(tf.argmax(y_pre,1), tf.argmax(v_ys,1))
accuracy = tf.reduce_mean(tf.cast(correct_prediction, tf.float32))
result = sess.run(accuracy, feed_dict={xs: v_xs, ys: v_ys, keep_prob: 1})
return result
def weight_variable(shape):
initial = tf.truncated_normal(shape, stddev=0.1)
return tf.Variable(initial)
def bias_variable(shape):
initial = tf.constant(0.1, shape=shape)
return tf.Variable(initial)
def conv2d(x, W):
# stride [1, x_movement, y_movement, 1]
# Must have strides[0] = strides[3] = 1
return tf.nn.conv2d(x, W, strides=[1, 1, 1, 1], padding='SAME')
def max_pool_2x2(x):
# stride [1, x_movement, y_movement, 1]
return tf.nn.max_pool(x, ksize=[1,2,2,1], strides=[1,2,2,1], padding='SAME')
# define placeholder for inputs to network
xs = tf.placeholder(tf.float32, [None, 784]) # 28x28
ys = tf.placeholder(tf.float32, [None, 10])
keep_prob = tf.placeholder(tf.float32)
x_image = tf.reshape(xs, [-1, 28, 28, 1])
# print(x_image.shape) # [n_samples, 28,28,1]
## conv1 layer ##
W_conv1 = weight_variable([5,5, 1,32]) # patch 5x5, in size 1, out size 32
b_conv1 = bias_variable([32])
h_conv1 = tf.nn.relu(conv2d(x_image, W_conv1) + b_conv1) # output size 28x28x32
h_pool1 = max_pool_2x2(h_conv1) # output size 14x14x32
## conv2 layer ##
W_conv2 = weight_variable([5,5, 32, 64]) # patch 5x5, in size 32, out size 64
b_conv2 = bias_variable([64])
h_conv2 = tf.nn.relu(conv2d(h_pool1, W_conv2) + b_conv2) # output size 14x14x64
h_pool2 = max_pool_2x2(h_conv2) # output size 7x7x64
## fc1 layer ##
W_fc1 = weight_variable([7*7*64, 1024])
b_fc1 = bias_variable([1024])
# [n_samples, 7, 7, 64] ->> [n_samples, 7*7*64]
h_pool2_flat = tf.reshape(h_pool2, [-1, 7*7*64])
h_fc1 = tf.nn.relu(tf.matmul(h_pool2_flat, W_fc1) + b_fc1)
h_fc1_drop = tf.nn.dropout(h_fc1, keep_prob)
## fc2 layer ##
W_fc2 = weight_variable([1024, 10])
b_fc2 = bias_variable([10])
prediction = tf.nn.softmax(tf.matmul(h_fc1_drop, W_fc2) + b_fc2)
# the error between prediction and real data
cross_entropy = tf.reduce_mean(-tf.reduce_sum(ys * tf.log(prediction),
reduction_indices=[1])) # loss
train_step = tf.train.AdamOptimizer(1e-4).minimize(cross_entropy)
sess = tf.Session()
sess.run(tf.global_variables_initializer())
for i in range(100):
batch_xs, batch_ys = mnist.train.next_batch(10)
sess.run(train_step, feed_dict={xs: batch_xs, ys: batch_ys, keep_prob: 0.5})
if i % 10 == 0:
print(compute_accuracy(
mnist.test.images, mnist.test.labels))
執行時,python崩潰並顯示以下消息:在拋出'std :: bad_alloc'的實例后調用terminate():std :: bad_alloc
我能夠指出,當我調用compute_accuracy
函數時,或者通常,當我加載整個mnist.test iamges和標簽時,會發生這種情況。 鑒於我希望使用這些數據,有關可以做什么的任何建議。 在不同的情況下,我已經能夠處理整個圖像。
我覺得你的內存不足了。 它在我的機器上運行正常(6GB顯卡)。 嘗試減小批量大小,或使用較小的完全連接層。
我遇到過同樣的問題。 我通過減少測試圖像的數量來解決它,以計算精度,例如我更換了
print(compute_accuracy(mnist.test.images, mnist.test.labels))
有類似的東西
batch_test = mnist.test.next_batch(5000)
print(compute_accuracy(batch_test[0], batch_test[1])
我希望這有幫助。
聲明:本站的技術帖子網頁,遵循CC BY-SA 4.0協議,如果您需要轉載,請注明本站網址或者原文地址。任何問題請咨詢:yoyou2525@163.com.