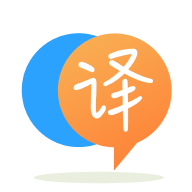
[英]Getting “InvalidArgumentError” in TensorFlow when working on MNIST data
[英]InvalidArgumentError error when evaluating new data in tensorflow
我正在嘗試使用張量流中的前饋DNN圖來預測新數據實例的分類。
這是該線程的延續, 在嘗試評估tensorflow中的新數據實例時已解決ValueError
代碼是:
import tensorflow as tf
import pandas as pd
dataframe = pd.read_csv("jfkspxstrain.csv") # Let's have Pandas load our dataset as a dataframe
dataframe = dataframe.drop(["Field6", "Field9", "rowid"], axis=1) # Remove columns we don't care about
dataframe.loc[:, ("y2")] = dataframe["y1"] == 0 # y2 is the negation of y1
dataframe.loc[:, ("y2")] = dataframe["y2"].astype(int) # Turn TRUE/FALSE values into 1/0
trainX = dataframe.loc[:, ['Field2', 'Field3', 'Field4', 'Field5', 'Field7', 'Field8', 'Field10']].as_matrix()
trainY = dataframe.loc[:, ["y1", 'y2']].as_matrix()
dataframe = pd.read_csv("jfkspxstest.csv") # Let's have Pandas load our dataset as a dataframe
dataframe = dataframe.drop(["Field6", "Field9", "rowid"], axis=1) # Remove columns we don't care about
dataframe.loc[:, ("y2")] = dataframe["y1"] == 0 # y2 is the negation of y1
dataframe.loc[:, ("y2")] = dataframe["y2"].astype(int) # Turn TRUE/FALSE values into 1/0
testX = dataframe.loc[:, ['Field2', 'Field3', 'Field4', 'Field5', 'Field7', 'Field8', 'Field10']].as_matrix()
testY = dataframe.loc[:, ["y1", 'y2']].as_matrix()
n_nodes_hl1 = 10
n_nodes_hl2 = 10
n_nodes_hl3 = 10
n_classes = 2
batch_size = 1
x = tf.placeholder('float',[None, 7])
y = tf.placeholder('float')
def neural_network_model(data):
hidden_1_layer = {'weights':tf.Variable(tf.random_normal([7, n_nodes_hl1])),
'biases':tf.Variable(tf.random_normal([n_nodes_hl1]))}
hidden_2_layer = {'weights':tf.Variable(tf.random_normal([n_nodes_hl1, n_nodes_hl2])),
'biases':tf.Variable(tf.random_normal([n_nodes_hl2]))}
hidden_3_layer = {'weights':tf.Variable(tf.random_normal([n_nodes_hl2, n_nodes_hl3])),
'biases':tf.Variable(tf.random_normal([n_nodes_hl3]))}
output_layer = {'weights':tf.Variable(tf.random_normal([n_nodes_hl1, n_classes])),
'biases':tf.Variable(tf.random_normal([n_classes]))}
l1 = tf.add(tf.matmul(data, hidden_1_layer['weights']), hidden_1_layer['biases'])
l1 = tf.nn.relu(l1)
l2 = tf.add(tf.matmul(l1, hidden_2_layer['weights']), hidden_2_layer['biases'])
l2 = tf.nn.relu(l2)
l3 = tf.add(tf.matmul(l2, hidden_3_layer['weights']), hidden_3_layer['biases'])
l3 = tf.nn.relu(l3)
output = tf.matmul(l3, output_layer['weights']) + output_layer['biases']
return output
def train_neural_network(x):
prediction = neural_network_model(x)
cost = tf.reduce_mean(tf.nn.softmax_cross_entropy_with_logits(prediction,y))
optimizer = tf.train.AdamOptimizer().minimize(cost)
hm_epochs = 5
with tf.Session() as sess:
sess.run(tf.initialize_all_variables())
for epoch in range(hm_epochs):
epoch_loss = .1
for _ in range(399):
_, c = sess.run([optimizer, cost], feed_dict = {x: trainX, y: trainY})
epoch_loss += c
print('Epoch', epoch, 'completed out of', hm_epochs, 'loss:', epoch_loss)
correct = tf.equal(tf.argmax(prediction,1), tf.argmax(y,1))
accuracy = tf.reduce_mean(tf.cast(correct, 'float'))
print('Accuracy:',accuracy.eval({x: testX, y: testY}))
classification = y.eval(feed_dict={x: [[51.0,10.0,71.0,65.0,5.0,70.0,30.06]]})
print (classification)
train_neural_network(x)
錯誤是:
InvalidArgumentError (see above for traceback): You must feed a value for placeholder tensor 'Placeholder_1' with dtype float
[[Node: Placeholder_1 = Placeholder[dtype=DT_FLOAT, shape=[], _device="/job:localhost/replica:0/task:0/cpu:0"]()]]
在這條線上
classification = y.eval(feed_dict={x: [[51.0,10.0,71.0,65.0,5.0,70.0,30.06]]})
代碼和數據在這里: https : //github.com/jhsmith12345/tensorflow
我看到這是在要求浮動值,我以為我已經喂了它。 任何幫助表示贊賞!
因此,假設y是您的目標數據,則在訓練期間,您需要為目標數據提供一個值,以便可以計算誤差。 線classification = y.eval
正在獲取預測,因此不應要求提供訓練數據-因此應classification = prediction.eval ...
classification = y.eval
classification = prediction.eval ...
def train_neural_network(x):
prediction = neural_network_model(x)
cost =tf.reduce_mean(tf.nn.softmax_cross_entropy_with_logits(prediction,y))
optimizer = tf.train.AdamOptimizer().minimize(cost)
hm_epochs = 5
with tf.Session() as sess:
sess.run(tf.initialize_all_variables())
for epoch in range(hm_epochs):
epoch_loss = .1
for _ in range(399):
_, c = sess.run([optimizer, cost], feed_dict = {x: trainX, y: trainY})
epoch_loss += c
print('Epoch', epoch, 'completed out of', hm_epochs, 'loss:', epoch_loss)
correct = tf.equal(tf.argmax(prediction,1), tf.argmax(y,1))
accuracy = tf.reduce_mean(tf.cast(correct, 'float'))
print('Accuracy:',accuracy.eval({x: testX, y: testY}))
classification = prediction.eval(feed_dict={x: [[51.0,10.0,71.0,65.0,5.0,70.0,30.06]]})
print (classification)
train_neural_network(x)
聲明:本站的技術帖子網頁,遵循CC BY-SA 4.0協議,如果您需要轉載,請注明本站網址或者原文地址。任何問題請咨詢:yoyou2525@163.com.