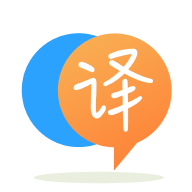
[英]Migrate tf.contrib.layers.batch_norm to Tensorflow 2.0
[英]Tensorflow - batch_normalizaiton layers
我嘗試建立一些神經網絡,我想在激活函數之前使用batch_normalization,但是我有一些問題。 我不確定是否正確使用了這些圖層。
graph = tf.Graph()
with graph.as_default():
x = tf.placeholder(tf.float32, shape=(batch_size, image_width, image_height, image_depth), name='x')
y = tf.placeholder(tf.float32, shape=(batch_size, num_categories), name='y')
keep_prob = tf.placeholder(tf.float32, name='keep_prob')
phase = tf.placeholder(tf.bool, name='phase')
layer1_weights = tf.Variable(tf.truncated_normal(shape=(filter_size, filter_size, image_depth, num_filters), stddev=0.01))
layer1_biases = tf.Variable(tf.ones(shape=(num_filters)))
layer2_weights = tf.Variable(tf.truncated_normal(shape=(filter_size, filter_size, num_filters, num_filters), stddev=0.01))
layer2_biases = tf.Variable(tf.ones(shape=(num_filters)))
layer3_weights = tf.Variable(tf.truncated_normal(shape=(filter_size, filter_size, num_filters, num_filters*2), stddev=0.01))
layer3_biases = tf.Variable(tf.ones(shape=(num_filters*2)))
layer4_weights = tf.Variable(tf.truncated_normal(shape=(filter_size, filter_size, num_filters*2, num_categories), stddev=0.01))
layer4_biases = tf.Variable(tf.ones(shape=(num_categories)))
x = batch_normalization(x, training=phase)
conv = tf.nn.conv2d(x, layer1_weights, [1, 1, 1, 1], padding='SAME') + layer1_biases
conv = batch_normalization(conv, training=phase)
conv = tf.nn.elu(conv)
conv = tf.nn.max_pool(conv, ksize=[1, 2, 2, 1], strides=[1, 2, 2, 1], padding='SAME')
conv = tf.nn.conv2d(conv, layer2_weights, [1, 1, 1, 1], padding='SAME') + layer2_biases
conv = batch_normalization(conv, training=phase)
conv = tf.nn.elu(conv)
conv = tf.nn.max_pool(conv, ksize=[1, 2, 2, 1], strides=[1, 2, 2, 1], padding='SAME')
conv = tf.nn.conv2d(conv, layer3_weights, [1, 1, 1, 1], padding='SAME') + layer3_biases
conv = batch_normalization(conv, training=phase)
conv = tf.nn.elu(conv)
conv = tf.nn.max_pool(conv, ksize=[1, 2, 2, 1], strides=[1, 2, 2, 1], padding='SAME')
conv = tf.nn.conv2d(conv, layer4_weights, [1, 1, 1, 1], padding='SAME') + layer4_biases
conv = batch_normalization(conv, training=phase)
conv = tf.nn.elu(conv)
conv = tf.layers.average_pooling2d(conv, [4, 4], [4, 4])
shape = conv.get_shape().as_list()
size = shape[1] * shape[2] * shape[3]
conv = tf.reshape(conv, shape=[-1, size])
y_ = tf.nn.softmax(conv)
# Loss function
loss = tf.reduce_mean(tf.nn.softmax_cross_entropy_with_logits(logits=conv, labels=y))
optimizer = tf.train.AdamOptimizer(learning_rate=0.0001)
extra_update_ops = tf.get_collection(tf.GraphKeys.UPDATE_OPS)
with tf.control_dependencies(extra_update_ops):
train_step = optimizer.minimize(loss)
# Accuracy
accuracy = tf.reduce_mean(tf.cast(tf.equal(tf.argmax(y_, axis=1),
tf.argmax(y, axis=1)),
tf.float32))
epochs = 1
dropout = 0.5
with tf.Session(graph=graph) as sess:
sess.run(tf.global_variables_initializer())
losses = []
acc = []
for e in range(epochs):
print('\nEpoch {}'.format(e+1))
for b in range(0, len(X_train), batch_size):
be = min(len(X_train), b + batch_size)
x_batch = X_train[b: be]
y_batch = y_train[b: be]
extra_update_ops = tf.get_collection(tf.GraphKeys.UPDATE_OPS)
l, a, _ = sess.run([loss, accuracy, train_step, extra_update_ops],
feed_dict={x: x_batch, y: y_batch, keep_prob: dropout, phase: True})
losses += [l]
acc += [a]
print('\r[{:5d}/{:5d}] loss = {}'.format(be, len(X_train), l), end='')
validation_accuracy = 0
for b in range(0, len(y_test), batch_size):
be = min(len(y_test), b + batch_size)
a = sess.run(accuracy, feed_dict={x: X_test[b: be], y: y_test[b: be], keep_prob: 1, phase: False})
validation_accuracy += a * (be - b)
validation_accuracy /= len(y_test)
training_accuracy = 0
for b in range(0, len(y_train), batch_size):
be = min(len(y_train), b + batch_size)
a = sess.run(accuracy, feed_dict={x: X_train[b: be], y: y_train[b: be], keep_prob: 1, phase: False})
training_accuracy += a * (be - b)
training_accuracy /= len(y_train)
plt.plot(losses)
plt.plot(acc)
plt.show()
print('Validation accuracy: {}'.format(validation_accuracy))
print()
print('Training accuracy: {}'.format(training_accuracy))
錯誤:我不知道為什么要說我沒有喂張量x?
InvalidArgumentError: You must feed a value for placeholder tensor 'x' with dtype float and shape [16,32,32,3]
[[Node: x = Placeholder[dtype=DT_FLOAT, shape=[16,32,32,3], _device="/job:localhost/replica:0/task:0/gpu:0"]()]]
在一行中,您將x
定義為占位符
x = tf.placeholder(tf.float32, shape=(batch_size, image_width, image_height, image_depth), name='x')
接下來的一行,您使用batch_normalization
函數調用的結果覆蓋x
變量
x = batch_normalization(x, training=phase)
x
不再是tf.placeholder
,因此,當您在feed_dict
使用它時,您不會覆蓋tf.placeholder
值,而是覆蓋tf.Tensor' generated by the
batch_normalization` op tf.Tensor' generated by the
的tf.Tensor' generated by the
。
要解決,你換線
x = batch_normalization(x, training=phase)
同
x_bn = batch_normalization(x, training=phase)
然后在x_bn
的行x_bn
x
替換為x_bn
。
這樣,占位符變量x
不會被覆蓋,您的代碼應該可以正常運行。
聲明:本站的技術帖子網頁,遵循CC BY-SA 4.0協議,如果您需要轉載,請注明本站網址或者原文地址。任何問題請咨詢:yoyou2525@163.com.