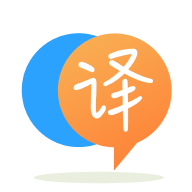
[英]Reset all weights and biases of the model in Keras (restore the model after training)
[英]reinitializing keras model weights after each training pass
我在Stack-overflow中注意到很少有與此類似的問題,但是沒有一個答案。
我有一個簡單的Keras模型:
def create_model(x_train, y_train, x_val, y_val):
# building the model
# compile
# fit
# return the score using model.predict
我正在應用交叉驗證(Kfold分層),如下所示:
skf = StratifiedKFold(y, n_folds=5, shuffle=True, random_state=0)
scores = []
for train_index, val_index in skf:
X_train, X_val = df[train_index], df[val_index]
y_train, y_val = y[train_index], y[val_index]
scores.append(create_model(X_train, y_train, X_val, y_val))
# point A
在每次訓練通過后(點A),我是否必須重新初始化模型權重,還是Keras庫管理此過程?
如果沒有,那么建議可以改善處理時間(如果可能,刷新內存嗎?..)。
我問這個問題是因為我正在將這個過程與Hyperopt庫一起用於Hyperparameter優化,並且我注意到,經過多次試驗,該模型開始比開始花費了更多時間。
編輯:作為示例,您可以注意到以下Hyperopt評估的處理時間,其中每遍均采用5折方法:
Hyperopt evals: 3%|▎ | 5/150 [16:09<7:54:20, 196.28s/it]
Hyperopt evals: 4%|▍ | 6/150 [22:33<10:06:20, 252.64s/it]
Hyperopt evals: 5%|▍ | 7/150 [26:20<9:43:55, 245.01s/it]
Hyperopt evals: 5%|▌ | 8/150 [33:33<11:53:16, 301.38s/it]
Hyperopt evals: 6%|▌ | 9/150 [41:56<14:10:16, 361.82s/it]
Hyperopt evals: 7%|▋ | 10/150 [45:56<12:38:50, 325.22s/it]
Hyperopt evals: 7%|▋ | 11/150 [48:19<10:26:55, 270.61s/it]
Hyperopt evals: 8%|▊ | 12/150 [54:11<11:18:28, 294.99s/it]
Hyperopt evals: 9%|▊ | 13/150 [58:45<10:58:57, 288.59s/it]
Hyperopt evals: 9%|▉ | 14/150 [1:05:57<12:31:47, 331.68s/it]
Hyperopt evals: 10%|█ | 15/150 [1:13:38<13:53:30, 370.45s/it]
Hyperopt evals: 11%|█ | 16/150 [1:17:36<12:18:28, 330.66s/it]
Hyperopt evals: 11%|█▏ | 17/150 [1:25:56<14:06:13, 381.75s/it]
Hyperopt evals: 12%|█▏ | 18/150 [1:31:54<13:43:38, 374.39s/it]
Hyperopt evals: 13%|█▎ | 19/150 [1:36:11<12:20:55, 339.35s/it]
Hyperopt evals: 13%|█▎ | 20/150 [1:45:06<14:22:20, 398.01s/it]
Hyperopt evals: 14%|█▍ | 21/150 [1:49:14<12:38:51, 352.95s/it]
Hyperopt evals: 15%|█▍ | 22/150 [1:54:45<12:18:47, 346.31s/it]
Hyperopt evals: 15%|█▌ | 23/150 [1:59:04<11:17:24, 320.04s/it]
Hyperopt evals: 16%|█▌ | 24/150 [2:04:05<11:00:29, 314.52s/it]
Hyperopt evals: 17%|█▋ | 25/150 [2:07:47<9:57:11, 286.65s/it]
Hyperopt evals: 17%|█▋ | 26/150 [2:12:47<10:00:37, 290.62s/it]
Hyperopt evals: 18%|█▊ | 27/150 [2:17:08<9:37:55, 281.91s/it]
Hyperopt evals: 19%|█▊ | 28/150 [2:22:46<10:07:15, 298.65s/it]
Hyperopt evals: 19%|█▉ | 29/150 [2:28:56<10:45:29, 320.08s/it]
Hyperopt evals: 20%|██ | 30/150 [2:34:55<11:03:44, 331.87s/it]
Hyperopt evals: 21%|██ | 31/150 [2:40:20<10:53:43, 329.61s/it]
Hyperopt evals: 21%|██▏ | 32/150 [2:46:19<11:05:42, 338.50s/it]
Hyperopt evals: 22%|██▏ | 33/150 [2:51:47<10:53:54, 335.34s/it]
Hyperopt evals: 23%|██▎ | 34/150 [2:58:14<11:18:06, 350.75s/it]
Hyperopt evals: 23%|██▎ | 35/150 [3:04:10<11:15:41, 352.53s/it]
Hyperopt evals: 24%|██▍ | 36/150 [3:13:59<13:24:26, 423.39s/it]
Hyperopt evals: 25%|██▍ | 37/150 [3:20:13<12:49:38, 408.66s/it]
Hyperopt evals: 25%|██▌ | 38/150 [3:25:55<12:05:23, 388.61s/it]
Hyperopt evals: 26%|██▌ | 39/150 [3:35:53<13:54:59, 451.35s/it]
Hyperopt evals: 27%|██▋ | 40/150 [3:44:26<14:21:12, 469.75s/it]
Hyperopt evals: 27%|██▋ | 41/150 [3:50:42<13:22:33, 441.77s/it]
Hyperopt evals: 28%|██▊ | 42/150 [3:58:03<13:14:29, 441.39s/it]
Hyperopt evals: 29%|██▊ | 43/150 [4:11:11<16:12:35, 545.38s/it]
Hyperopt evals: 29%|██▉ | 44/150 [4:19:18<15:32:40, 527.93s/it]
Hyperopt evals: 30%|███ | 45/150 [4:26:03<14:19:21, 491.06s/it]
Hyperopt evals: 31%|███ | 46/150 [4:34:32<14:20:31, 496.46s/it]
Hyperopt evals: 31%|███▏ | 47/150 [4:45:01<15:20:25, 536.17s/it]
Hyperopt evals: 32%|███▏ | 48/150 [4:54:11<15:18:45, 540.45s/it]
Hyperopt evals: 33%|███▎ | 49/150 [4:58:42<12:53:19, 459.40s/it]
Hyperopt evals: 33%|███▎ | 50/150 [5:04:07<11:38:30, 419.11s/it]
Hyperopt evals: 34%|███▍ | 51/150 [5:12:48<12:22:14, 449.85s/it]
Hyperopt evals: 35%|███▍ | 52/150 [5:20:37<12:23:57, 455.49s/it]
Hyperopt evals: 35%|███▌ | 53/150 [5:28:18<12:19:19, 457.31s/it]
Hyperopt evals: 36%|███▌ | 54/150 [5:37:02<12:43:26, 477.15s/it]
Hyperopt evals: 37%|███▋ | 55/150 [5:45:21<12:46:00, 483.80s/it]
Hyperopt evals: 37%|███▋ | 56/150 [5:51:07<11:33:16, 442.51s/it]
Hyperopt evals: 38%|███▊ | 57/150 [5:59:38<11:57:39, 463.00s/it]
Hyperopt evals: 39%|███▊ | 58/150 [6:11:19<13:39:13, 534.27s/it]
Hyperopt evals: 39%|███▉ | 59/150 [6:28:06<17:05:39, 676.26s/it]
Hyperopt evals: 40%|████ | 60/150 [6:37:29<16:03:23, 642.27s/it]
Hyperopt evals: 41%|████ | 61/150 [6:43:38<13:51:06, 560.30s/it]
Hyperopt evals: 41%|████▏ | 62/150 [6:52:41<13:33:52, 554.92s/it]
Hyperopt evals: 42%|████▏ | 63/150 [7:00:05<12:36:40, 521.84s/it]
Hyperopt evals: 43%|████▎ | 64/150 [7:12:13<13:56:21, 583.50s/it]
Hyperopt evals: 43%|████▎ | 65/150 [7:20:03<12:58:38, 549.62s/it]
Hyperopt evals: 44%|████▍ | 66/150 [7:31:56<13:58:08, 598.68s/it]
Hyperopt evals: 45%|████▍ | 67/150 [7:44:48<15:00:05, 650.67s/it]
Hyperopt evals: 45%|████▌ | 68/150 [7:57:32<15:35:45, 684.70s/it]
在每次訓練通過后(點A),我是否必須重新初始化模型權重,還是Keras庫管理此過程?
在檢查了文檔和手動實驗之后:在我看來,Keras負責重新初始化權重,因此不需要。
如果沒有,那么建議可以改善處理時間(如果可能,刷新內存嗎?..)。
在我的情況下,處理時間在增加,原因是:
1- Hyperopt使用貝葉斯優化技術,因此每次選擇下一個參數集時都會嘗試,以根據先驗概率選擇更好的方法
2-我正在盡早停止。
因此,在每個下一個評估中,hyperopt庫開始選擇更好的參數集,其中模型也開始比以前更好地收斂。這意味着,減少了早期停止的使用,並增加了處理時間(以完成整個時期)。
聲明:本站的技術帖子網頁,遵循CC BY-SA 4.0協議,如果您需要轉載,請注明本站網址或者原文地址。任何問題請咨詢:yoyou2525@163.com.