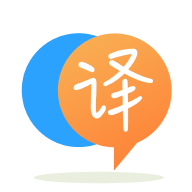
[英]why my model does not train over all the data (Tensorflow 2)
[英]Why does my keras model not train at all?
我的代碼是:
from keras.models import Sequential
from keras.layers import Dense, Dropout, Masking
import numpy as np
import pandas as pd
dataset = pd.read_csv("data/train.csv", header=0)
dataset = dataset.fillna(0)
X = dataset.drop(columns=['YearRemodAdd', "Id", "SalePrice"], axis=1)
Y = dataset[['SalePrice']]
X = pd.get_dummies(X, columns=["MSSubClass", "MSZoning",
"Street", "Alley", "LotShape",
"LandContour", "Utilities", "LotConfig",
"LandSlope", "Neighborhood", "Condition1",
"Condition2", "BldgType", "HouseStyle",
"YearBuilt", "RoofStyle", "RoofMatl",
"Exterior1st", "Exterior2nd", "MasVnrType",
"ExterQual", "ExterCond", "Foundation",
"BsmtQual", "BsmtCond", "BsmtExposure",
"BsmtFinType1", "BsmtFinType2", "Heating",
"HeatingQC", "CentralAir", "Electrical",
"KitchenQual", "Functional", "FireplaceQu",
"GarageType", "GarageFinish", "GarageQual",
"GarageCond", "PavedDrive", "PoolQC",
"Fence", "MiscFeature", "MoSold",
"YrSold", "SaleType", "SaleCondition"])
Ymax = Y['SalePrice'].max()
Y = Y['SalePrice'].apply(lambda x: float(x) / Ymax)
input_units = X.shape[1]
print(X)
print(Y)
model = Sequential()
model.add(Dense(input_units, input_dim=input_units, activation='relu'))
model.add(Dense(input_units, activation='relu'))
model.add(Dense(input_units, activation='relu'))
model.add(Dense(1, activation='sigmoid'))
model.compile(loss='binary_crossentropy',
optimizer='adam', metrics=['mse'])
model.fit(X, Y, epochs=250, batch_size=50,
shuffle=True, validation_split=0.05, verbose=2)
scores = model.evaluate(X, Y)
print("\n%s: %.2f%%" % (model.metrics_names[1], scores[1]*100))
我的數據像:
Id,MSSubClass,MSZoning,LotFrontage,LotArea,Street,Alley,LotShape,LandContour,Utilities,LotConfig,LandSlope,Neighborhood,Condition1,Condition2,BldgType,HouseStyle,OverallQual,OverallCond,YearBuilt,YearRemodAdd,RoofStyle,RoofMatl,Exterior1st,Exterior2nd,MasVnrType,MasVnrArea,ExterQual,ExterCond,Foundation,BsmtQual,BsmtCond,BsmtExposure,BsmtFinType1,BsmtFinSF1,BsmtFinType2,BsmtFinSF2,BsmtUnfSF,TotalBsmtSF,Heating,HeatingQC,CentralAir,Electrical,1stFlrSF,2ndFlrSF,LowQualFinSF,GrLivArea,BsmtFullBath,BsmtHalfBath,FullBath,HalfBath,BedroomAbvGr,KitchenAbvGr,KitchenQual,TotRmsAbvGrd,Functional,Fireplaces,FireplaceQu,GarageType,GarageYrBlt,GarageFinish,GarageCars,GarageArea,GarageQual,GarageCond,PavedDrive,WoodDeckSF,OpenPorchSF,EnclosedPorch,3SsnPorch,ScreenPorch,PoolArea,PoolQC,Fence,MiscFeature,MiscVal,MoSold,YrSold,SaleType,SaleCondition,SalePrice
1,60,RL,65,8450,Pave,NA,Reg,Lvl,AllPub,Inside,Gtl,CollgCr,Norm,Norm,1Fam,2Story,7,5,2003,2003,Gable,CompShg,VinylSd,VinylSd,BrkFace,196,Gd,TA,PConc,Gd,TA,No,GLQ,706,Unf,0,150,856,GasA,Ex,Y,SBrkr,856,854,0,1710,1,0,2,1,3,1,Gd,8,Typ,0,NA,Attchd,2003,RFn,2,548,TA,TA,Y,0,61,0,0,0,0,NA,NA,NA,0,2,2008,WD,Normal,208500
2,20,RL,80,9600,Pave,NA,Reg,Lvl,AllPub,FR2,Gtl,Veenker,Feedr,Norm,1Fam,1Story,6,8,1976,1976,Gable,CompShg,MetalSd,MetalSd,None,0,TA,TA,CBlock,Gd,TA,Gd,ALQ,978,Unf,0,284,1262,GasA,Ex,Y,SBrkr,1262,0,0,1262,0,1,2,0,3,1,TA,6,Typ,1,TA,Attchd,1976,RFn,2,460,TA,TA,Y,298,0,0,0,0,0,NA,NA,NA,0,5,2007,WD,Normal,181500
我的結果是:
Epoch 123/250
- 0s - loss: 3.8653 - mean_squared_error: 0.0687 - val_loss: 3.8064 - val_mean_squared_error: 0.0639
Epoch 124/250
像2個紀元之后就卡在那里了。 我該怎么做才能防止它卡得這么快?
看來您正在研究回歸問題(即預測連續值)。 至少需要考慮兩件事:
正如@Mitiku在注釋部分中提到的,數據中有一些NA
(即丟失)值。 這是使損失變得nan
的原因之一。 刪除具有NA
值的行,或者將NA
值替換為特定值(例如0)。有關處理缺失數據的更多信息,請參見此答案 。
使用accuracy
作為回歸問題的度量標准沒有意義,因為它僅對分類任務有效。 而是使用諸如mse
(即均方誤差)或mae
(即平均絕對誤差)之類的回歸度量。
請在代碼中應用以上兩點,然后報告培訓的進行情況,我將根據需要更新此答案。
聲明:本站的技術帖子網頁,遵循CC BY-SA 4.0協議,如果您需要轉載,請注明本站網址或者原文地址。任何問題請咨詢:yoyou2525@163.com.