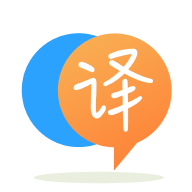
[英]Calculate cumulative sum in a group_by() on two different sets of columns in dplyr
[英]dplyr group_by over elements of two columns
我的數據集的簡化版本可以通過以下方式復制:
df <- data.frame(buyer = c("A","C","B"),
seller = c("B","D","E"),
amount = c(1,2,3))
我正在尋找一個優選的dplyr解決方案來實現以下目標。
buyer seller amount
A B 1
C D 2
B E 3
應該為每個代理(A,B,C,D,E)生成分組摘要
output
agent total_amount
A 1
B 4 #(=1+3)
C 2
D 2
我可以group_by買家和賣家,然后添加結果,但這不優雅,有點麻煩。
library(dplyr)
res_b <- df %>%
group_by(buyer) %>%
summarise(total_amount=sum(amount))
res_s <- df %>%
group_by(seller) %>%
summarise(total_amount=sum(amount))
任何幫助表示贊賞。 其他解決方案(不是整齊的)顯然也是受歡迎的。
編輯:應該說我的原始數據集大約為6000萬觀察。
我們可以先轉換為長格式並進行簡單的聚合,即
library(tidyverse)
df %>%
gather(var, agent, -amount) %>%
group_by(agent) %>%
summarise(total_amount = sum(amount))
這使,
# A tibble: 5 x 2 agent total_amount <chr> <dbl> 1 A 1 2 B 4 3 C 2 4 D 2 5 E 3
您可以嘗試使用data.table
以提高效率。 這是上面的tidyverse
代碼的直接翻譯,
library(data.table)
dt1 <- setDT(df)
melt(dt1, measure.vars = c('buyer', 'seller'), id.vars = 'amount', value.name = "agent"
)[, .(total_amount = sum(amount)), by = agent][]
# agent total_amount
#1: A 1
#2: C 2
#3: B 4
#4: D 2
#5: E 3
標桿
library(bench)
bnch <-
press(
n = 10^c(5, 6, 7, 8),{
set.seed(1);df_big <- data.frame(buyer = sample(LETTERS, n, replace = TRUE), seller = sample(LETTERS, n, replace = TRUE), amount = sample(1:10, n, replace = TRUE))
set.seed(1);dt_big <- data.table(buyer = sample(LETTERS, n, replace = TRUE), seller = sample(LETTERS, n, replace = TRUE), amount = sample(1:10, n, replace = TRUE))
mark(
dplyr = {
df_big %>%
gather(var, agent, -amount) %>%
group_by(agent) %>%
summarise(total_amount = sum(amount))},
dt_melt = {
melt(dt_big, measure.vars = c('buyer', 'seller'), id.vars = 'amount')[
, .(total_amount = sum(amount)), by = .(agent = value) ][order(agent), ]},
dt_rbind = {
rbind(dt_big[ , .(x = sum(amount)), by = .(agent = buyer) ],
dt_big[ , .(x = sum(amount)), by = .(agent = seller) ])[
order(agent), .(total_amount = sum(x)), by = agent]}
)})
bnch
# # A tibble: 12 x 15
# expression n min mean median max `itr/sec` mem_alloc n_gc n_itr
# <chr> <dbl> <bch:tm> <bch:tm> <bch:tm> <bch:tm> <dbl> <bch:byt> <dbl> <int>
# 1 dplyr 1.00e5 15.75ms 16.4ms 15.85ms 22.7ms 61.0 6.88MB 0 31
# 2 dt_melt 1.00e5 6.34ms 8.39ms 8.48ms 9.2ms 119. 7.01MB 1 53
# 3 dt_rbind 1.00e5 7.45ms 7.82ms 7.75ms 8.9ms 128. 4.06MB 0 64
# 4 dplyr 1.00e6 149.07ms 159.32ms 160.07ms 168.06ms 6.28 68.68MB 0 4
# 5 dt_melt 1.00e6 49.85ms 58.88ms 60.52ms 62.58ms 17.0 69.34MB 1 7
# 6 dt_rbind 1.00e6 35.73ms 38.05ms 38.61ms 40.01ms 26.3 39.09MB 1 12
# 7 dplyr 1.00e7 1.78s 1.78s 1.78s 1.78s 0.560 686.66MB 2 1
# 8 dt_melt 1.00e7 648.77ms 648.77ms 648.77ms 648.77ms 1.54 692.61MB 1 1
# 9 dt_rbind 1.00e7 389.32ms 390.37ms 390.37ms 391.41ms 2.56 387.54MB 3 2
# 10 dplyr 1.00e8 18.73s 18.73s 18.73s 18.73s 0.0534 6.71GB 3 1
# 11 dt_melt 1.00e8 8.18s 8.18s 8.18s 8.18s 0.122 6.76GB 2 1
# 12 dt_rbind 1.00e8 4.15s 4.15s 4.15s 4.15s 0.241 3.78GB 1 1
ggplot2::autoplot(bnch)
正如你提到的"60 million observations"
,這是使用data.table
, rbind而不是melt的另一個解決方案:
library(data.table)
setDT(df)
rbind(df[ , .(x = sum(amount)), by = .(agent = buyer) ],
df[ , .(x = sum(amount)), by = .(agent = seller) ])[
, .(total_amount = sum(x)), by = agent]
# agent total_amount
# 1: A 1
# 2: C 2
# 3: B 4
# 4: D 2
# 5: E 3
訪問行兩次並按c(buyer, seller)
分組c(buyer, seller)
:
# setup
library(data.table)
setDT(df)
df[, c("buyer", "seller") := .(as.character(buyer), as.character(seller))]
# aggregate
df[rep(1:.N, 2), .(total = sum(amount)), by=.(agent = c(df$buyer, df$seller))]
agent total
1: A 1
2: C 2
3: B 4
4: D 2
5: E 3
我認為,由於積極的NSE解析,需要df$
stuff。 我不確定by=
或keyby=
在這里應該更快。
基准測試 :我用zx8的數據嘗試了這個,發現它的重量是rbind
兩倍,如果我重新制定...
dt_big[, data.table(agent = c(buyer,seller), v = amount)][, sum(v), by=agent]
# 7.4 seconds vs 4.0 for dt_rbind with n = 10^8
最后,還有一個快速但又冗長的選項:
groupingsets(dt_big,
by=c("buyer", "seller"),
sets = list("buyer", "seller"),
j = sum(amount))[is.na(buyer), buyer := seller][, sum(V1), by=buyer])
# 4.2 seconds
聲明:本站的技術帖子網頁,遵循CC BY-SA 4.0協議,如果您需要轉載,請注明本站網址或者原文地址。任何問題請咨詢:yoyou2525@163.com.