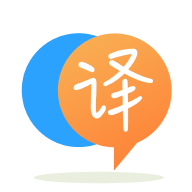
[英]Gensim 1.0.1 on Python 3.5 TypeError: object of type 'map' has no len()?
[英]python gensim word2vec gives typeerror TypeError: object of type 'generator' has no len() on custom dataclass
我試圖讓word2vec在python3中工作,但是由於我的數據集太大而無法輕松放入內存中,因此我正在通過迭代器(從zip文件)加載它。 但是,當我運行它時,我得到了錯誤
Traceback (most recent call last):
File "WordModel.py", line 85, in <module>
main()
File "WordModel.py", line 15, in main
word2vec = gensim.models.Word2Vec(data,workers=cpu_count())
File "/home/thijser/.local/lib/python3.7/site-packages/gensim/models/word2vec.py", line 783, in __init__
fast_version=FAST_VERSION)
File "/home/thijser/.local/lib/python3.7/site-packages/gensim/models/base_any2vec.py", line 759, in __init__
self.build_vocab(sentences=sentences, corpus_file=corpus_file, trim_rule=trim_rule)
File "/home/thijser/.local/lib/python3.7/site-packages/gensim/models/base_any2vec.py", line 936, in build_vocab
sentences=sentences, corpus_file=corpus_file, progress_per=progress_per, trim_rule=trim_rule)
File "/home/thijser/.local/lib/python3.7/site-packages/gensim/models/word2vec.py", line 1591, in scan_vocab
total_words, corpus_count = self._scan_vocab(sentences, progress_per, trim_rule)
File "/home/thijser/.local/lib/python3.7/site-packages/gensim/models/word2vec.py", line 1576, in _scan_vocab
total_words += len(sentence)
TypeError: object of type 'generator' has no len()
這是代碼:
import zipfile
import os
from ast import literal_eval
from lxml import etree
import io
import gensim
from multiprocessing import cpu_count
def main():
data = TrainingData("/media/thijser/Data/DataSets/uit2")
print(len(data))
word2vec = gensim.models.Word2Vec(data,workers=cpu_count())
word2vec.save('word2vec.save')
class TrainingData:
size=-1
def __init__(self, dirname):
self.data_location = dirname
def __len__(self):
if self.size<0:
for zipfile in self.get_zips_in_folder(self.data_location):
for text_file in self.get_files_names_from_zip(zipfile):
self.size=self.size+1
return self.size
def __iter__(self): #might not fit in memory otherwise
yield self.get_data()
def get_data(self):
for zipfile in self.get_zips_in_folder(self.data_location):
for text_file in self.get_files_names_from_zip(zipfile):
yield self.preproccess_text(text_file)
def stripXMLtags(self,text):
tree=etree.parse(text)
notags=etree.tostring(tree, encoding='utf8', method='text')
return notags.decode("utf-8")
def remove_newline(self,text):
text.replace("\\n"," ")
return text
def preproccess_text(self,text):
text=self.stripXMLtags(text)
text=self.remove_newline(text)
return text
def get_files_names_from_zip(self,zip_location):
files=[]
archive = zipfile.ZipFile(zip_location, 'r')
for info in archive.infolist():
files.append(archive.open(info.filename))
return files
def get_zips_in_folder(self,location):
zip_files = []
for root, dirs, files in os.walk(location):
for name in files:
if name.endswith((".zip")):
filepath=root+"/"+name
zip_files.append(filepath)
return zip_files
main()
for d in data:
for dd in d :
print(type(dd))
確實告訴我dd是字符串類型,並且包含正確的預處理字符串(每個字符串的長度在50到5000個單詞之間)。
討論后更新:
您的TrainingData
類__iter__()
函數沒有提供一個生成器,該生成器依次返回每個文本,而是提供了一個返回單個其他生成器的生成器。 ( yield
水平太多。)這不是Word2Vec
所期望的。
將您的__iter__()
方法的主體更改為簡單...
return self.get_data()
......使__iter__()
是你的代名詞get_data()
和剛剛返回相同的文字,通過文字發生器get_data()
不,應該有所幫助。
原始答案:
您沒有顯示在get_data()
引用的TrainingData.preproccess_text()
(sic)方法,該方法實際上是創建Word2Vec
正在處理的數據的Word2Vec
。 而且,正是這些數據產生了錯誤。
Word2Vec
要求其sentences
語料庫是一個可迭代的序列 (適用於生成器),其中每個單獨的項目都是一個字符串令牌列表 。
從該錯誤看來,您的TrainingData
序列中的各個項目本身可能是生成器,而不是具有可讀len()
列表。
(另外,如果您可能選擇在此處使用生成器,因為單個文本可能會非常長,請注意,gensim Word2Vec
和相關類僅針對長度不超過10000個單詞標記的單個文本進行訓練。第10000個字符將被靜默忽略。如果您對此有所擔心,則應將您的源文本預分解為10000個令牌或更少的單個文本。)
聲明:本站的技術帖子網頁,遵循CC BY-SA 4.0協議,如果您需要轉載,請注明本站網址或者原文地址。任何問題請咨詢:yoyou2525@163.com.