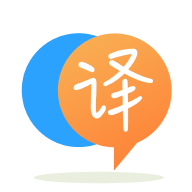
[英]how to assign the topics retried via LDA in R using "textmineR" package to the specific documents
[英]How do i measure perplexity scores on a LDA model made with the textmineR package in R?
我在 R 中制作了一個 LDA 主題模型,使用 textmineR 包,如下所示。
## get textmineR dtm
dtm2 <- CreateDtm(doc_vec = dat2$fulltext, # character vector of documents
ngram_window = c(1, 2),
doc_names = dat2$names,
stopword_vec = c(stopwords::stopwords("da"), custom_stopwords),
lower = T, # lowercase - this is the default value
remove_punctuation = T, # punctuation - this is the default
remove_numbers = T, # numbers - this is the default
verbose = T,
cpus = 4)
dtm2 <- dtm2[, colSums(dtm2) > 2]
dtm2 <- dtm2[, str_length(colnames(dtm2)) > 2]
############################################################
## RUN & EXAMINE TOPIC MODEL
############################################################
# Draw quasi-random sample from the pc
set.seed(34838)
model2 <- FitLdaModel(dtm = dtm2,
k = 8,
iterations = 500,
burnin = 200,
alpha = 0.1,
beta = 0.05,
optimize_alpha = TRUE,
calc_likelihood = TRUE,
calc_coherence = TRUE,
calc_r2 = TRUE,
cpus = 4)
那么問題是: 1. 我應該應用哪個函數來獲得 textmineR 包中的困惑度分數? 我似乎找不到一個。
2. 我如何衡量不同數量主題(k)的復雜度分數?
正如所問:除非您自己明確編程,否則無法使用textmineR
計算困惑textmineR
。 TBH,我從未見過您無法通過可能性和連貫性獲得的困惑的價值,所以我沒有實現它。
但是, text2vec
包確實有一個實現。 請參閱以下示例:
library(textmineR)
# model ships with textmineR as example
m <- nih_sample_topic_model
# dtm ships with textmineR as example
d <- nih_sample_dtm
# get perplexity
p <- text2vec::perplexity(X = d,
topic_word_distribution = m$phi,
doc_topic_distribution = m$theta)
聲明:本站的技術帖子網頁,遵循CC BY-SA 4.0協議,如果您需要轉載,請注明本站網址或者原文地址。任何問題請咨詢:yoyou2525@163.com.