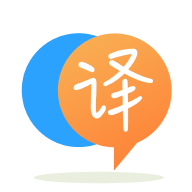
[英]Tensorflow 2 ModelCheckpoint callback with multiclass recall custom metric
[英]Tensorflow Callback as Custom Metric for CTC
為了在我的 model(在 TensorFlow 版本 2.1.0 中編寫)的訓練過程中產生更多指標,例如字符錯誤率 (CER) 和單詞錯誤率 (WER),我創建了一個回調以傳遞給合適的 function我的 model。它能夠在一個紀元結束時生成 CER 和 WER。
這是我的第二個選擇,因為我想為此創建一個自定義指標,但您只能將 keras 后端功能用於自定義指標。 有沒有人對如何將下面的回調轉換為自定義指標有任何建議(然后可以在驗證和/或培訓數據的培訓期間計算)?
我遇到的一些障礙是:
class Metrics(tf.keras.callbacks.Callback):
def __init__(self, valid_data, steps):
"""
valid_data is a TFRecordDataset with batches of 100 elements per batch, shuffled and repeated infinitely.
steps define the amount of batches per epoch
"""
super(Metrics, self).__init__()
self.valid_data = valid_data
self.steps = steps
def on_train_begin(self, logs={}):
self.cer = []
self.wer = []
def on_epoch_end(self, epoch, logs={}):
imgs = []
labels = []
for idx, (img, label) in enumerate(self.valid_data.as_numpy_iterator()):
if idx >= self.steps:
break
imgs.append(img)
labels.extend(label)
imgs = np.array(imgs)
labels = np.array(labels)
out = self.model.predict((batch for batch in imgs))
input_length = len(max(out, key=len))
out = np.asarray(out)
out_len = np.asarray([input_length for _ in range(len(out))])
decode, log = K.ctc_decode(out,
out_len,
greedy=True)
decode = [[[int(p) for p in x if p != -1] for x in y] for y in decode][0]
for (pred, lab) in zip(decode, labels):
dist = editdistance.eval(pred, lab)
self.cer.append(dist / (max(len(pred), len(lab))))
self.wer.append(not np.array_equal(pred, lab))
print("Mean CER: {}".format(np.mean([self.cer], axis=1)[0]))
print("Mean WER: {}".format(np.mean([self.wer], axis=1)[0]))
在 TF 2.3.1 中已解決,但也應適用於 2.x 的早期版本。
一些備注:
on_epoch_end
的顯式額外計算),或者我相信。 將其實現為tensorflow.keras.metrics.Metric
的子類似乎是正確的方法,並且在 epoch 進行時會產生結果(如果設置正確)。tf.edit_distance
(使用稀疏張量)很容易計算 CER 的編輯距離,隨后可以使用一些 tf 邏輯來計算 WER。self.model.compile(optimizer=opt, loss=loss, metrics=[CERMetric(), WERMetric()])
class CERMetric(tf.keras.metrics.Metric):
"""
A custom Keras metric to compute the Character Error Rate
"""
def __init__(self, name='CER_metric', **kwargs):
super(CERMetric, self).__init__(name=name, **kwargs)
self.cer_accumulator = self.add_weight(name="total_cer", initializer="zeros")
self.counter = self.add_weight(name="cer_count", initializer="zeros")
def update_state(self, y_true, y_pred, sample_weight=None):
input_shape = K.shape(y_pred)
input_length = tf.ones(shape=input_shape[0]) * K.cast(input_shape[1], 'float32')
decode, log = K.ctc_decode(y_pred,
input_length,
greedy=True)
decode = K.ctc_label_dense_to_sparse(decode[0], K.cast(input_length, 'int32'))
y_true_sparse = K.ctc_label_dense_to_sparse(y_true, K.cast(input_length, 'int32'))
decode = tf.sparse.retain(decode, tf.not_equal(decode.values, -1))
distance = tf.edit_distance(decode, y_true_sparse, normalize=True)
self.cer_accumulator.assign_add(tf.reduce_sum(distance))
self.counter.assign_add(len(y_true))
def result(self):
return tf.math.divide_no_nan(self.cer_accumulator, self.counter)
def reset_states(self):
self.cer_accumulator.assign(0.0)
self.counter.assign(0.0)
class WERMetric(tf.keras.metrics.Metric):
"""
A custom Keras metric to compute the Word Error Rate
"""
def __init__(self, name='WER_metric', **kwargs):
super(WERMetric, self).__init__(name=name, **kwargs)
self.wer_accumulator = self.add_weight(name="total_wer", initializer="zeros")
self.counter = self.add_weight(name="wer_count", initializer="zeros")
def update_state(self, y_true, y_pred, sample_weight=None):
input_shape = K.shape(y_pred)
input_length = tf.ones(shape=input_shape[0]) * K.cast(input_shape[1], 'float32')
decode, log = K.ctc_decode(y_pred,
input_length,
greedy=True)
decode = K.ctc_label_dense_to_sparse(decode[0], K.cast(input_length, 'int32'))
y_true_sparse = K.ctc_label_dense_to_sparse(y_true, K.cast(input_length, 'int32'))
decode = tf.sparse.retain(decode, tf.not_equal(decode.values, -1))
distance = tf.edit_distance(decode, y_true_sparse, normalize=True)
correct_words_amount = tf.reduce_sum(tf.cast(tf.not_equal(distance, 0), tf.float32))
self.wer_accumulator.assign_add(correct_words_amount)
self.counter.assign_add(len(y_true))
def result(self):
return tf.math.divide_no_nan(self.wer_accumulator, self.counter)
def reset_states(self):
self.wer_accumulator.assign(0.0)
self.counter.assign(0.0)
las,我還沒有找到如何在一個指標中同時實現 CER 和 WER(因為它有很多重復代碼),如果有人知道如何這樣做,請與我聯系。
嘿,這個解決方案真的幫了我很多。 截至目前,有 TensorFlow 2.10 版本,因此對於這個版本,我編寫了 WER 和 CER 指標的組合,這是最終的工作代碼:
import tensorflow as tf
class CWERMetric(tf.keras.metrics.Metric):
""" A custom TensorFlow metric to compute the Character Error Rate
"""
def __init__(self, name='CWER', **kwargs):
super(CWERMetric, self).__init__(name=name, **kwargs)
self.cer_accumulator = tf.Variable(0.0, name="cer_accumulator", dtype=tf.float32)
self.wer_accumulator = tf.Variable(0.0, name="wer_accumulator", dtype=tf.float32)
self.counter = tf.Variable(0, name="counter", dtype=tf.int32)
def update_state(self, y_true, y_pred, sample_weight=None):
input_shape = tf.keras.backend.shape(y_pred)
input_length = tf.ones(shape=input_shape[0], dtype='int32') * tf.cast(input_shape[1], 'int32')
decode, log = tf.keras.backend.ctc_decode(y_pred, input_length, greedy=True)
decode = tf.keras.backend.ctc_label_dense_to_sparse(decode[0], input_length)
y_true_sparse = tf.cast(tf.keras.backend.ctc_label_dense_to_sparse(y_true, input_length), "int64")
decode = tf.sparse.retain(decode, tf.not_equal(decode.values, -1))
distance = tf.edit_distance(decode, y_true_sparse, normalize=True)
correct_words_amount = tf.reduce_sum(tf.cast(tf.not_equal(distance, 0), tf.float32))
self.wer_accumulator.assign_add(correct_words_amount)
self.cer_accumulator.assign_add(tf.reduce_sum(distance))
self.counter.assign_add(len(y_true))
def result(self):
return {
"CER": tf.math.divide_no_nan(self.cer_accumulator, tf.cast(self.counter, tf.float32)),
"WER": tf.math.divide_no_nan(self.wer_accumulator, tf.cast(self.counter, tf.float32))
}
我仍然需要檢查它是否正確計算了 CER 和 WER,我會發現缺少某些東西,我會更新它。
聲明:本站的技術帖子網頁,遵循CC BY-SA 4.0協議,如果您需要轉載,請注明本站網址或者原文地址。任何問題請咨詢:yoyou2525@163.com.