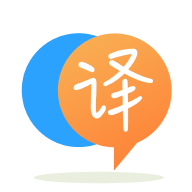
[英]AttributeError: module 'tensorflow.python.framework.ops' has no attribute '_TensorLike'
[英]I cannot understand this AttributeError : module 'tensorflow.python.framework.ops' has no attribute '_TensorLike'
我在 Google Colab 中使用 Tensorflow 並且出現這樣的錯誤。 我 100% 確定我的代碼在前一天工作,但是當我嘗試重新運行它時,我無法解決這個錯誤。
AttributeError: in user code:
/usr/local/lib/python3.6/dist-packages/tensorflow/python/keras/engine/training.py:806 train_function *
return step_function(self, iterator)
/usr/local/lib/python3.6/dist-packages/keras/backend/tensorflow_backend.py:81 symbolic_fn_wrapper *
return func(*args, **kwargs)
/usr/local/lib/python3.6/dist-packages/keras/metrics.py:80 __call__ *
update_op = self.update_state(*args, **kwargs)
/usr/local/lib/python3.6/dist-packages/keras/utils/metrics_utils.py:42 decorated *
update_op = update_state_fn(*args, **kwargs)
/usr/local/lib/python3.6/dist-packages/keras/metrics.py:1707 update_state *
metrics_utils.ConfusionMatrix.FALSE_NEGATIVES: self.false_negatives,
/usr/local/lib/python3.6/dist-packages/keras/utils/metrics_utils.py:274 update_confusion_matrix_variables *
thresh_tiled = K.tile(
/usr/local/lib/python3.6/dist-packages/keras/backend/tensorflow_backend.py:2682 tile *
if not is_tensor(n):
/usr/local/lib/python3.6/dist-packages/keras/backend/tensorflow_backend.py:703 is_tensor *
return isinstance(x, tf_ops._TensorLike) or tf_ops.is_dense_tensor_like(x)
AttributeError: module 'tensorflow.python.framework.ops' has no attribute '_TensorLike'
你能幫我解決這個問題嗎? 謝謝。
我的代碼直到錯誤是:
!pip uninstall tensorflow -y
!pip install tensorflow-gpu
from tensorflow.python.client import device_lib
print(device_lib.list_local_devices())
from IPython.core.interactiveshell import InteractiveShell
InteractiveShell.ast_node_interactivity = "all"
import matplotlib.pyplot as plt # Required to plot data
import numpy as np # Management of arrays
import os # System utils
from scipy.io import loadmat # Required to load .mat files
from scipy import signal # Required for signal processing
import tensorflow as tf
import keras
import random
from collections import Counter
from imblearn.over_sampling import SMOTE
from keras.utils import to_categorical
from sklearn.utils import shuffle
from sklearn.utils import class_weight
from keras.constraints import max_norm
SEED = 1234
tf.random.set_seed(SEED)
np.random.seed(SEED)
random.seed(SEED)
os.environ['PYTHONHASHSEED']=str(SEED)
from google.colab import drive
drive.mount('/content/drive')
save_models = True
x=15
y=3
train_set_path="drive/My Drive/Colab Notebooks/IFMBEproglearn/train_{0}".format(x)+"_{0}.mat".format(y)
test_set_path="drive/My Drive/Colab Notebooks/IFMBEproglearn/test_{0}".format(x)+"_{0}.mat".format(y)
train_events_path="drive/My Drive/Colab Notebooks/IFMBEproglearn/trainEvents_{0}".format(x)+"_{0}.txt".format(y)
train_labels_path="drive/My Drive/Colab Notebooks/IFMBEproglearn/trainLabels_{0}".format(x)+"_{0}.txt".format(y)
train_targets_path="drive/My Drive/Colab Notebooks/IFMBEproglearn/trainTargets_{0}".format(x)+"_{0}.txt".format(y)
test_events_path="drive/My Drive/Colab Notebooks/IFMBEproglearn/testEvents_{0}".format(x)+"_{0}.txt".format(y)
test_targets_path="drive/My Drive/Colab Notebooks/IFMBEproglearn/testTargets_{0}".format(x)+"_{0}.txt".format(y)
numRunsTest_path="drive/My Drive/Colab Notebooks/IFMBEproglearn/runs_per_block_{0}".format(x)+"_{0}.txt".format(y)
if not os.path.exists(train_set_path):
print("Missing file: {}", train_set_path)
else:
# Load the required data
training_set = loadmat(train_set_path)['newData']
if not os.path.exists(test_set_path):
print("Missing file: {}", test_set_path)
else:
# Load the required data
testing_set = loadmat(test_set_path)['newData']
if not os.path.exists(train_events_path):
print("Missing file: {}", train_events_path)
else:
# Load the required data
f = open(train_events_path, 'r+')
train_events = [line for line in f.readlines()]
f.close()
if not os.path.exists(train_labels_path):
print("Missing file: {}", train_labels_path)
else:
# Load the required data
f = open(train_labels_path, 'r+')
train_labels = [line for line in f.readlines()]
f.close()
if not os.path.exists(train_targets_path):
print("Missing file: {}", train_targets_path)
else:
# Load the required data
f = open(train_targets_path, 'r+')
train_targets = [line for line in f.readlines()]
f.close()
if not os.path.exists(test_events_path):
print("Missing file: {}", test_events_path)
else:
# Load the required data
f = open(test_events_path, 'r+')
test_events = [line for line in f.readlines()]
f.close()
if not os.path.exists(test_targets_path):
print("Missing file: {}", test_targets_path)
else:
# Load the required data
f = open(test_targets_path, 'r+')
test_targets = [line for line in f.readlines()]
f.close()
if not os.path.exists(numRunsTest_path):
print("Missing file: {}", numRunsTest_path)
else:
# Load the required data
f = open(numRunsTest_path, 'r+')
test_numRuns = [line for line in f.readlines()]
f.close()
training_array=np.asarray(training_set)
training_array=np.moveaxis(training_array, -1, 0)
testing_array=np.asarray(testing_set)
testing_array=np.moveaxis(testing_array, -1, 0)
shaped_testing_array=np.expand_dims(testing_array,-1)
shaped_testing_array.shape
events_array=np.asarray(train_events)
shaped_events=np.expand_dims(events_array,-1)
shaped_events.shape
labels_array=np.asarray(train_labels)
shaped_labels=np.expand_dims(labels_array,-1)
shaped_labels.shape
targets_array=np.asarray(train_targets)
test_events_array=np.asarray(test_events)
shaped_test_events=np.expand_dims(test_events_array,-1)
shaped_test_events.shape
test_targets_array=np.asarray(test_targets)
shaped_test_targets=np.expand_dims(test_targets_array,-1)
shaped_test_targets.shape
test_numRuns_array=np.asarray(test_numRuns)
shaped_test_numRuns=np.expand_dims(test_numRuns_array,-1)
shaped_test_numRuns.shape
training_array.shape
shaped_training_array=np.expand_dims(training_array,-1)
shaped_training_array.shape
shaped_targets=np.expand_dims(targets_array,-1)
shaped_targets.shape
shaped_targets_cat = to_categorical(shaped_targets)
shaped_targets_cat.shape
numChannels=8
numSamples=150
numClasses=2
SHAPE=(numChannels,numSamples,1)
model=tf.keras.Sequential([tf.keras.layers.Input(shape=SHAPE),
tf.keras.layers.ZeroPadding2D(input_shape=(numChannels,numSamples,1),padding=(0,32)),
tf.keras.layers.Conv2D(filters=16,kernel_size=(1,65),strides=(1,1),padding='valid',data_format='channels_last',use_bias=False),
tf.keras.layers.BatchNormalization(axis=-1,momentum=0.99,epsilon=0.001,center=False,scale=False),
tf.keras.layers.DepthwiseConv2D(kernel_size=(8,1),strides=(1, 1),padding='valid',depth_multiplier=2,data_format='channels_last',kernel_constraint=max_norm(1.),use_bias=False),
tf.keras.layers.BatchNormalization(axis=-1, momentum=0.99, epsilon=0.001,center=False,scale=False),
tf.keras.layers.ELU(),
tf.keras.layers.AveragePooling2D(pool_size=(1, 4),strides=None,padding='valid',data_format=None),
tf.keras.layers.Dropout(rate=0.50,noise_shape=None,seed=None),
tf.keras.layers.ZeroPadding2D(padding=(0,8)),
tf.keras.layers.SeparableConvolution2D(filters=16,kernel_size=(1,17),strides=(1,1),padding='valid',use_bias=False),
tf.keras.layers.BatchNormalization(axis=-1,momentum=0.99,epsilon=0.001,center=False,scale=False),
tf.keras.layers.ELU(),
tf.keras.layers.AveragePooling2D(pool_size=(1,8),strides=None,padding='valid',data_format=None),
tf.keras.layers.Dropout(rate=0.50,noise_shape=None,seed=None),
tf.keras.layers.Flatten(),
tf.keras.layers.Dense(numClasses,activation='softmax')])
model.summary()
model.compile(
optimizer = tf.keras.optimizers.Adam(learning_rate=0.005, beta_1=0.9, beta_2=0.999, epsilon=1e-07, amsgrad=False, name='Adam'),
loss = 'categorical_crossentropy',
metrics = ['accuracy',keras.metrics.AUC(name='auc')]
)
class_weights = class_weight.compute_class_weight('balanced',
np.unique(targets_array),
targets_array)
class_weights = dict(enumerate(class_weights))
class_weights
callbacks = []
es_callback = tf.keras.callbacks.EarlyStopping(monitor='val_loss', patience=5, mode='min', restore_best_weights=True)
callbacks.append(es_callback)
#class_weights={0:1 , 1:1}
在我自己檢查數據集之前,一切都很完美。但是,使用此代碼片段,我收到錯誤:
history = model.fit(shaped_training_array, shaped_targets_cat, batch_size=128, epochs=1000, validation_split=0.15, callbacks=callbacks, class_weight=class_weights, shuffle=True)
希望這會奏效。 https://github.com/tensorflow/tensorflow/issues/38589#issuecomment-665930503
from tensorflow.python.framework import tensor_util
def is_tensor(x):
return tensor_util.is_tensor(x)
像這樣導入:
from tensorflow.keras.utils import to_categorical
from tensorflow.keras.constraints import max_norm
代替:
from keras.utils import to_categorical
from keras.constraints import max_norm
keras
和tensorflow.keras
之間存在一些兼容性問題
您也可以嘗試重新安裝keras
和tensorflow
感謝您提供可能的解決方案。 我通過轉換行解決了這個問題:
!pip uninstall tensorflow -y
!pip install tensorflow-gpu
進入:
!pip uninstall tensorflow -y
!pip install tensorflow==2.2.0
我已經為 Tensorflow 2.2.0 編寫了代碼,但它已經更新到 2.3.0。 這就是問題所在。 再次感謝!
聲明:本站的技術帖子網頁,遵循CC BY-SA 4.0協議,如果您需要轉載,請注明本站網址或者原文地址。任何問題請咨詢:yoyou2525@163.com.