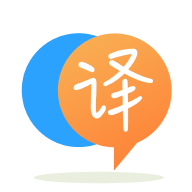
[英]Find all combinations among levels of a factor and output to list of data frames
[英]Sampling data frames maintaining all levels of factor variables
我需要對一個數據框進行采樣,以維護結果中的所有級別的因素。 然后我想得到這個樣本的補集——即那些不屬於樣本的行。 我的最終目標是為回歸分析創建訓練和測試樣本。 為了成功地做到這一點,我需要確保所有級別的因子變量都在訓練樣本上表示出來。
我嘗試過的方法(下面的示例代碼)是使用 dplyr::group_by 結合 dplyr::slice_sample 然后 dplyr::anti_join 來獲得測試。 由於某種原因,它不起作用。 要么我遺漏了有關這些功能應該如何工作的信息,要么它們的行為不符合預期。
我也嘗試過基於這個問題的方法。 他們沒有工作,因為(1)我需要保證所有級別的多個因素都得到了代表,(2)我想 select 觀察的一部分,而不是特定的數字。
> library(tidyverse)
>
> set.seed(72)
>
> data <- tibble(y = rnorm(100), x1 = rnorm(100),
+ x2 = sample(letters, 100, T), x3 = sample(LETTERS, 100, T))
> data
# A tibble: 100 x 4
y x1 x2 x3
<dbl> <dbl> <chr> <chr>
1 1.37 -0.737 c C
2 1.16 1.66 c T
3 0.0344 -0.319 q P
4 1.03 -0.963 k C
5 0.636 0.961 i H
6 0.319 0.761 g L
7 0.216 0.860 u M
8 1.31 0.887 g M
9 -0.594 2.70 m I
10 -0.542 0.517 u C
# … with 90 more rows
>
> train_data <- data %>%
+ group_by(x2, x3) %>%
+ slice_sample(prop = .7)
> train_data # clearly this is not what I want
# A tibble: 8 x 4
# Groups: x2, x3 [8]
y x1 x2 x3
<dbl> <dbl> <chr> <chr>
1 1.23 -0.297 c A
2 1.11 0.689 e O
3 0.559 0.353 e Z
4 -1.65 -1.71 l M
5 -0.777 1.31 l X
6 0.784 0.309 s E
7 0.755 -0.362 u X
8 -0.768 0.292 v H
>
> test_data <- data %>%
+ anti_join(train_data)
Joining, by = c("y", "x1", "x2", "x3")
> test_data # my goal was that the training data would have 70% and the test data would have around 30% of the full sample.
# A tibble: 92 x 4
y x1 x2 x3
<dbl> <dbl> <chr> <chr>
1 1.37 -0.737 c C
2 1.16 1.66 c T
3 0.0344 -0.319 q P
4 1.03 -0.963 k C
5 0.636 0.961 i H
6 0.319 0.761 g L
7 0.216 0.860 u M
8 1.31 0.887 g M
9 -0.594 2.70 m I
10 -0.542 0.517 u C
# … with 82 more rows
>
> reg <- lm(y ~ x1 + x2 + x3, train_data)
> predict(reg, newdata = test_data) # I obviously still have the same problem
Error in model.frame.default(Terms, newdata, na.action = na.action, xlev = object$xlevels) :
factor x2 has new levels a, b, d, f, g, h, i, j, k, m, n, o, p, q, r, t, w, x, y, z
>
>
我不得不將您的數據擴展到 10,000 行,以獲得每個分類變量組合的合理數量的觀察值。 然后,我使用了nest_by()
(版本 1.0.1)中的dplyr
() 並對每個子集進行了采樣。
library(dplyr)
set.seed(72)
data <- tibble(y = rnorm(10000), x1 = rnorm(10000),
x2 = sample(letters, 10000, T), x3 = sample(LETTERS, 10000, T))
train <- data %>%
nest_by(x2, x3, .key = "xy") %>%
mutate(sample = list(xy[sample(1:nrow(xy),
size = round(0.7*nrow(xy))),])) %>%
select(-xy) %>%
summarize(sample)
train
# A tibble: 6,975 x 4
# Groups: x2, x3 [676]
x2 x3 y x1
<chr> <chr> <dbl> <dbl>
1 a A -0.539 -1.22
2 a A -0.664 0.453
3 a A -1.32 -0.831
4 a A 0.765 0.258
5 a A -0.462 0.764
6 a A 1.86 -0.0400
7 a A -1.15 1.02
8 a A 0.244 -0.823
9 a A -0.277 -0.744
10 a A 0.221 -0.292
# ... with 6,965 more rows
test <- data%>%
anti_join(train)
test
# A tibble: 3,025 x 4
y x1 x2 x3
<dbl> <dbl> <chr> <chr>
1 0.636 1.71 b P
2 0.319 -0.851 b K
3 1.31 -1.61 r A
4 -1.03 0.436 a B
5 -0.672 -1.43 g O
6 -1.42 -0.637 l L
7 0.879 -1.78 t G
8 0.935 -1.44 g C
9 -2.21 -0.842 v F
10 -1.00 -2.40 i D
# ... with 3,015 more rows
我可以運行你的lm()
和predict()
而不會出錯。
如果您有舊版本的dplyr
,這里有一種稍微不同的方法來制作train
。
library(dplyr)
library(tidyr)
library(purrr)
train <-data %>%
nest(x2, x3) %>%
mutate(sample = map(data, function(df) {df[sample(1:nrow(df), round(0.7*nrow(df))),]}) %>%
select(-data) %>%
unnest(sample)
您的代碼/方法沒有任何問題。 你沒有足夠的觀察。 有很多組只有 1 行,當以 0.7 比例采樣時,會將其舍入為 0。如果將樣本更改為 1000 行,相同的代碼可以正常工作而不會出錯。
library(dplyr)
data <- tibble(y = rnorm(1000), x1 = rnorm(1000),
x2 = sample(letters, 1000, T), x3 = sample(LETTERS, 1000, T))
train_data <- data %>%
group_by(x2, x3) %>%
slice_sample(prop = 0.7)
test_data <- data %>% anti_join(train_data)
reg <- lm(y ~ x1 + x2 + x3, train_data)
predict(reg, newdata = test_data)
如果在您的真實數據中,您的組只有 1 行,您可以對它們進行采樣,使其選擇max
為 1 或(0.7 * 組中的行數)。
train_data <- data %>% group_by(x2, x3) %>% sample_n(max(0.7*n(), 1))
(這里使用了sample_n
,因為我不能在slice_sample
中使用n()
)。
聲明:本站的技術帖子網頁,遵循CC BY-SA 4.0協議,如果您需要轉載,請注明本站網址或者原文地址。任何問題請咨詢:yoyou2525@163.com.