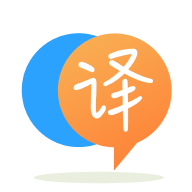
[英]InvalidArgumentError: Incompatible shapes with Keras LSTM Net
[英]Incompatible Shapes: Tensorflow/Keras Sequential LSTM with Autoencoder
我正在嘗試為時間序列數據設置 LSTM 自動編碼器/解碼器,並在嘗試訓練 model 時不斷出現Incompatible shapes
錯誤。按照步驟並使用此示例中的玩具數據。 請參閱下面的代碼和結果。 注意 Tensorflow 版本 2.3.0。
創建數據。 將數據放入序列中,以(樣本、時間戳、特征)的形式對 LSTM 進行時間化。
timeseries = np.array([[0.1, 0.2, 0.3, 0.4, 0.5, 0.6, 0.7, 0.8, 0.9],
[0.1**3, 0.2**3, 0.3**3, 0.4**3, 0.5**3, 0.6**3, 0.7**3, 0.8**3, 0.9**3]]).transpose()
timeseries_df = pd.DataFrame(timeseries)
def create_sequenced_dataset(X, time_steps=10):
Xs, ys = [], [] # start empty list
for i in range(len(X) - time_steps): # loop within range of data frame minus the time steps
v = X.iloc[i:(i + time_steps)].values # data from i to end of the time step
Xs.append(v)
ys.append(X.iloc[i + time_steps].values)
return np.array(Xs), np.array(ys) # convert lists into numpy arrays and return
X, y = create_sequenced_dataset(timeseries_df, time_steps=3)
timesteps = X.shape[1]
n_features = X.shape[2]
使用重復向量提供的自動編碼器/解碼器創建 LSTM model,並嘗試訓練 model。
model = Sequential()
model.add(LSTM(128, input_shape=(timesteps, n_features), return_sequences=False))
model.add(RepeatVector(timesteps))
model.add(LSTM(128, return_sequences=True))
model.add(TimeDistributed(Dense(n_features)))
model.compile(optimizer='adam', loss='mse')
model.summary()
model.fit(X, y, epochs=10, batch_size=4)
一直報錯:
tensorflow.python.framework.errors_impl.InvalidArgumentError: Incompatible shapes: [4,3,2] vs. [4,2]
[[node gradient_tape/mean_squared_error/BroadcastGradientArgs (defined at <ipython-input-9-56896428cea9>:1) ]] [Op:__inference_train_function_10833]
X 看起來像:
array([[[0.1 , 0.001],
[0.2 , 0.008],
[0.3 , 0.027]],
[[0.2 , 0.008],
[0.3 , 0.027],
[0.4 , 0.064]],
[[0.3 , 0.027],
[0.4 , 0.064],
[0.5 , 0.125]],
[[0.4 , 0.064],
[0.5 , 0.125],
[0.6 , 0.216]],
[[0.5 , 0.125],
[0.6 , 0.216],
[0.7 , 0.343]],
[[0.6 , 0.216],
[0.7 , 0.343],
[0.8 , 0.512]]])
你看起來像:
array([[0.4 , 0.064],
[0.5 , 0.125],
[0.6 , 0.216],
[0.7 , 0.343],
[0.8 , 0.512],
[0.9 , 0.729]])
希望譯者正確翻譯我的想法。 我一開始也不明白問題是什么,但后來我又看了一遍自動編碼器的定義。 由於這是一個自動編碼器,我們將 X 應用於輸入和 output(y 不以任何方式參與 model,因為我們試圖確定數據 X 中的依賴關系,然后重新創建它們)。 有些人有關於這個主題的代碼(y = x.copy()),而在這里它適用(model.fit(X,X,epochs = 300,batch_size = 5,verbose = 0))。
正如消息明確指出的那樣,這是您要傳遞給 model 的形狀問題。
根據您給出的上述數據,X 具有(6, 3, 2)
的形狀,而 Y 具有(6, 2)
的形狀,這是不兼容的。
下面是修改后的代碼,其輸入與您使用形狀為(6,3,2)
的X
和Y
所采用的示例相同。
model = Sequential()
model.add(LSTM(128, input_shape=(timesteps, n_features), return_sequences=False))
model.add(RepeatVector(timesteps))
model.add(LSTM(128, return_sequences=True))
model.add(TimeDistributed(Dense(n_features)))
model.compile(optimizer='adam', loss='mse')
model.summary()
model.fit(X,Y, epochs=10, batch_size=4)
結果:
Epoch 1/10
2/2 [==============================] - 0s 5ms/step - loss: 0.0069
Epoch 2/10
2/2 [==============================] - 0s 4ms/step - loss: 0.0065
Epoch 3/10
2/2 [==============================] - 0s 4ms/step - loss: 0.0065
Epoch 4/10
2/2 [==============================] - 0s 4ms/step - loss: 0.0062
Epoch 5/10
2/2 [==============================] - 0s 4ms/step - loss: 0.0059
Epoch 6/10
2/2 [==============================] - 0s 4ms/step - loss: 0.0053
Epoch 7/10
2/2 [==============================] - 0s 5ms/step - loss: 0.0048
Epoch 8/10
2/2 [==============================] - 0s 5ms/step - loss: 0.0046
Epoch 9/10
2/2 [==============================] - 0s 5ms/step - loss: 0.0044
Epoch 10/10
2/2 [==============================] - 0s 6ms/step - loss: 0.0043
<tensorflow.python.keras.callbacks.History at 0x7ff352f9ccf8>
聲明:本站的技術帖子網頁,遵循CC BY-SA 4.0協議,如果您需要轉載,請注明本站網址或者原文地址。任何問題請咨詢:yoyou2525@163.com.