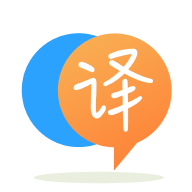
[英]How to split images into test and train set using my own data in TensorFlow
[英]how to split data into test and train using tensorflow
我對張量流很陌生。 我參加了一個在線課程,但我仍然有很多與數據預處理相關的問題。 如果有人能幫助我,我將不勝感激!!
我的目標是訓練一個模型,根據內部結構將葡萄牙語名詞分為兩個性別類別(女性和男性)。 因此,為此,我有一個包含大約 4300 個名詞及其類別(F 和 M 標簽)的文件。
第一個問題:我打開了名詞文件,我首先標記了單詞,然后填充了它們。 我有一個把標簽放在一個單獨的文件中。 標簽文件是一個 txt 列表,其中包含標簽“f”和“m”。 我已將它們轉換為 0 和 1 整數,然后將它們轉換為一個 numpy 數組。 我還將填充的名詞轉換為 numpy 數組。 那是對的嗎?
奇怪的是我把epoch的數量設置為100,但是程序一直在訓練……
第二個問題:
如何將我的火車和標簽分成 test 和 test_labels?
到目前為止,我的代碼如下:
from collections import defaultdict
import nltk
from nltk.tokenize import word_tokenize
from nltk.tokenize import sent_tokenize,wordpunct_tokenize
import re
import os
import sys
from pathlib import Path
import numpy as np
import numpy
import tensorflow as tf
while True:
try:
file_to_open =Path(input("Please, insert your file path: "))
with open(file_to_open,'r', encoding="utf-8") as f:
words = f.read()
break
except FileNotFoundError:
print("\nFile not found. Better try again")
except IsADirectoryError:
print("\nIncorrect Directory path.Try again")
corpus=words.split('\n')
labels = []
new_labels=[]
nouns = []
for i in corpus:
if i == '0':
labels.append(i)
elif i== '1':
labels.append(i)
else:
nouns.append(i)
for x in labels:
new_labels.append(int(x))
training_labels= numpy.array(new_labels)
training_nouns=[]
for w in nouns:
a=list(w)
b=' '.join([str(elem) for elem in a]) + ',' + ' '
training_nouns.append(b)
vocab_size = 10000
embedding_dim = 16
max_length = 120
trunc_type='post'
oov_tok = "<OOV>"
from tensorflow.keras.preprocessing.text import Tokenizer
from tensorflow.keras.preprocessing.sequence import pad_sequences
tokenizer = Tokenizer(num_words = vocab_size, oov_token=oov_tok)
tokenizer.fit_on_texts(training_nouns)
word_index = tokenizer.word_index
nouns_sequences = tokenizer.texts_to_sequences(training_nouns)
padded = pad_sequences(nouns_sequences,maxlen=max_length)
model = tf.keras.Sequential([
tf.keras.layers.Embedding(vocab_size, embedding_dim,
input_length=max_length),
tf.keras.layers.GlobalAveragePooling1D(),
tf.keras.layers.Dense(36, activation='relu'),
tf.keras.layers.Dense(1, activation='sigmoid')
])
model.compile(loss='binary_crossentropy',optimizer='adam',metrics=.
['accuracy'])
model.summary()
training_padded = np.array(padded)
num_epochs = 150
model.fit(training_padded, training_labels, epochs=num_epochs)
如果你不應該使用 Tensorflow。 您可以像這樣使用train_test_split
scikit-learn
函數(您可以繼續使用 tensorflow):
from sklearn.model_selection import train_test_split
train_data,train_labels,test_data,test_labels=train_test_split(YOUR DATA,YOUR LABELS)
請參閱此處了解更多信息。
聲明:本站的技術帖子網頁,遵循CC BY-SA 4.0協議,如果您需要轉載,請注明本站網址或者原文地址。任何問題請咨詢:yoyou2525@163.com.