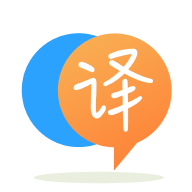
[英]Why passing a list as a parameter performs better than passing a generator?
[英]Python list performs better than numpy array?
我最近報名參加了一門科學的 python 課程,並在課堂上展示了 numpy 陣列在某些情況下的性能如何優於列表。 對於統計模擬,我嘗試了這兩種方法,令人驚訝的是,numpy 陣列需要更長的時間才能完成該過程。 有人可以幫我找到我的(可能的)錯誤嗎?
我的第一個想法是代碼的編寫方式可能有問題,但我不知道它是怎么出錯的。 該腳本計算一個人平均需要多少次嘗試才能完成一組已排序的貼紙:
我使用了 function 並且沒有外部模塊。
import random as rd
import statistics as st
def collectStickers(experiments, collectible):
obtained = []
attempts = 0
while(len(obtained) < collectible):
new_sticker = rd.randint(1, collectible)
if new_sticker not in obtained:
obtained.append(new_sticker)
attempts += 1
experiments.append(attempts)
experiments = []
collectible = 20
rep_experiment = 100000
for i in range(1, rep_experiment):
collectStickers(experiments, collectible)
print(st.mean(experiments))
對於像這樣的簡單實驗,處理時間似乎還可以,但對於更復雜的目的,13.8 秒太多了。
72.06983069830699
[Finished in 13.8s]
我無法使用任何 function,因為當我遵循與上述相同的邏輯時出現以下錯誤:
RuntimeWarning:空切片的平均值。
RuntimeWarning:在 double_scalars 中遇到無效值
所以我只是采取了幼稚的方式:
import random as rd
import numpy as np
experiments = np.array([])
rep_experiment = 100000
for i in range(1, rep_experiment):
obtained = np.array([])
attempts = 0
while(len(obtained) < 20):
new_sticker = rd.randint(1, 20)
if new_sticker not in obtained:
obtained = np.append(obtained, new_sticker)
attempts += 1
experiments = np.append(experiments, attempts)
print(np.mean(experiments))
幾乎慢了 4 倍!
使用function有區別嗎?
72.03112031120311
[Finished in 54.2s]
要真正考慮到 numpy arrays 的強大功能,您需要以 numpy 方式進行編程。 例如,嘗試像這樣對實驗進行矢量化:
def vectorized():
rep_experiment = 100000
collectible = 20
# array of falses
obtained = np.zeros(rep_experiment, dtype=bool)
attempts = np.zeros(rep_experiment, dtype=int)
targets = np.zeros((rep_experiment, collectible), dtype=bool)
x = np.arange(0,100000, step=1, dtype=int)
while False in targets:
r = np.random.randint(0, collectible, size=rep_experiment)
# add the new stickers to the collected target
targets[x,r] = True
# if collected all set obtained to True
obtained[np.sum(targets, axis=1)==collectible] = True
# increments the not obtained values
attempts[~obtained] += 1
print(attempts.mean(), attempts.std())
檢查速度,對我來說大約是 50 X
np.append
在追加之前復制數組。
您的程序可能大部分時間都在做那些不必要的副本
experiments = np.append(experiments, attempts)
正如預期的那樣,用預定義的數組替換二次型np.append()
使得包裝器 function 的速度大致相同。
用一組替換obtained
的貼紙列表會使事情變得更快。
然而,瓶頸是一個緩慢的隨機數生成器。 運行 cProfile 顯示 75% 的執行時間花費在randint()
中。
請參閱下面的代碼以獲取結果(在我的機器上)。
import random
import statistics
import timeit
import numpy as np
collectible = 20
rep_experiment = 10000
def original_collect_stickers():
obtained = []
attempts = 0
while len(obtained) < collectible:
new_sticker = random.randint(1, collectible)
if new_sticker not in obtained:
obtained.append(new_sticker)
attempts += 1
return attempts
def set_collect_stickers():
obtained = set()
attempts = 0
n = 0
while n < collectible:
new_sticker = random.randint(1, collectible)
if new_sticker not in obtained:
obtained.add(new_sticker)
n += 1
attempts += 1
return attempts
def repeat_with_list(fn):
experiments = []
for i in range(rep_experiment):
experiments.append(fn())
return statistics.mean(experiments)
def repeat_with_numpy(fn):
experiments = np.zeros(rep_experiment)
for i in range(rep_experiment):
experiments[i] = fn()
return np.mean(experiments)
def time_fn(name, fn, n=3):
time_taken = timeit.timeit(fn, number=n) / n
result = fn() # once more to get the result too
print(f"{name:15}: {time_taken:.6f}, result {result}")
for wrapper in (repeat_with_list, repeat_with_numpy):
for fn in (original_collect_stickers, set_collect_stickers):
time_fn(f"{wrapper.__name__} {fn.__name__}", lambda: wrapper(fn))
結果是
repeat_with_list original_collect_stickers: 0.747183, result 71.7912
repeat_with_list set_collect_stickers: 0.688952, result 72.1002
repeat_with_numpy original_collect_stickers: 0.752644, result 72.0978
repeat_with_numpy set_collect_stickers: 0.685355, result 71.7515
使用fastrand
庫的pcg32bounded()
生成器,即new_sticker = fastrand.pcg32bounded(collectible)
使事情變得非常快:
repeat_with_list original_collect_stickers: 0.761186, result 72.0185
repeat_with_list set_collect_stickers: 0.690244, result 71.878
repeat_with_list set_collect_stickers_fastrand: 0.116410, result 71.9323
repeat_with_numpy original_collect_stickers: 0.759154, result 71.8604
repeat_with_numpy set_collect_stickers: 0.696563, result 71.5482
repeat_with_numpy set_collect_stickers_fastrand: 0.114212, result 71.6369
聲明:本站的技術帖子網頁,遵循CC BY-SA 4.0協議,如果您需要轉載,請注明本站網址或者原文地址。任何問題請咨詢:yoyou2525@163.com.