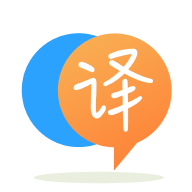
[英]tidymodel error, when calling predict function is asking for target variable
[英]Why does deploying a tidymodel with vetiver throw a error when there's a variable with role as ID?
當模型在配方中包含一個角色為 ID 的變量時,我無法使用香根草部署 tidymodel 並獲得預測。 在圖像中看到以下錯誤:
{“錯誤”:“500 - 內部服務器錯誤”,“消息”:“錯誤:缺少以下必需列:'Fake_ID'。\n”}
虛擬示例的代碼如下。 我是否需要從模型和配方中刪除 ID 變量才能使 Plumber API 工作?
#Load libraries
library(recipes)
library(parsnip)
library(workflows)
library(pins)
library(plumber)
library(stringi)
#Upload data
data(Sacramento, package = "modeldata")
#Create fake IDs for testing
Sacramento$Fake_ID <- stri_rand_strings(nrow(Sacramento), 10)
# Train model
Sacramento_recipe <- recipe(formula = price ~ type + sqft + beds + baths + zip + Fake_ID, data = Sacramento) %>%
update_role(Fake_ID, new_role = "ID") %>%
step_zv(all_predictors())
rf_spec <- rand_forest(mode = "regression") %>% set_engine("ranger")
rf_fit <-
workflow() %>%
add_model(rf_spec) %>%
add_recipe(Sacramento_recipe) %>%
fit(Sacramento)
# Create vetiver object
v <- vetiver::vetiver_model(rf_fit, "sacramento_rf")
v
# Allow for model versioning and sharing
model_board <- board_temp()
model_board %>% vetiver_pin_write(v)
# Deploying model
pr() %>%
vetiver_api(v) %>%
pr_run(port = 8088)
到今天為止,香根草尋找“模具” workflows::extract_mold(rf_fit)
並且只取出預測器來創建 ptype。 但是,當您從工作流進行預測時,它確實需要所有變量,包括非預測變量。 如果您已經用非預測器訓練了一個模型,那么從今天開始,您可以通過傳入自定義ptype
來使 API 工作:
library(recipes)
#> Loading required package: dplyr
#>
#> Attaching package: 'dplyr'
#> The following objects are masked from 'package:stats':
#>
#> filter, lag
#> The following objects are masked from 'package:base':
#>
#> intersect, setdiff, setequal, union
#>
#> Attaching package: 'recipes'
#> The following object is masked from 'package:stats':
#>
#> step
library(parsnip)
library(workflows)
library(pins)
library(plumber)
library(stringi)
data(Sacramento, package = "modeldata")
Sacramento$Fake_ID <- stri_rand_strings(nrow(Sacramento), 10)
Sacramento_recipe <-
recipe(formula = price ~ type + sqft + beds + baths + zip + Fake_ID,
data = Sacramento) %>%
update_role(Fake_ID, new_role = "ID") %>%
step_zv(all_predictors())
rf_spec <- rand_forest(mode = "regression") %>% set_engine("ranger")
rf_fit <-
workflow() %>%
add_model(rf_spec) %>%
add_recipe(Sacramento_recipe) %>%
fit(Sacramento)
library(vetiver)
## this is probably easiest because this model uses a simple formula
## if there is more complex preprocessing, select the variables
## from `Sacramento` via dplyr or similar
sac_ptype <- extract_recipe(rf_fit) %>%
bake(new_data = Sacramento, -all_outcomes()) %>%
vctrs::vec_ptype()
v <- vetiver_model(rf_fit, "sacramento_rf", save_ptype = sac_ptype)
v
#>
#> ── sacramento_rf ─ <butchered_workflow> model for deployment
#> A ranger regression modeling workflow using 6 features
pr() %>%
vetiver_api(v)
#> # Plumber router with 2 endpoints, 4 filters, and 0 sub-routers.
#> # Use `pr_run()` on this object to start the API.
#> ├──[queryString]
#> ├──[body]
#> ├──[cookieParser]
#> ├──[sharedSecret]
#> ├──/ping (GET)
#> └──/predict (POST)
由reprex 包於 2022-03-10 創建 (v2.0.1)
您是否正在使用非預測變量訓練生產模型? 您介意在 GitHub 上打開一個問題來進一步解釋您的用例嗎?
聲明:本站的技術帖子網頁,遵循CC BY-SA 4.0協議,如果您需要轉載,請注明本站網址或者原文地址。任何問題請咨詢:yoyou2525@163.com.