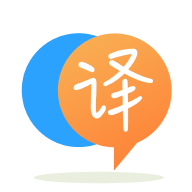
[英]How to add an array of list as a new column to a spark dataframe using pyspark
[英]How do I add a new column to a Spark DataFrame (using PySpark)?
我有一个 Spark DataFrame(使用 PySpark 1.5.1)并且想添加一个新列。
我尝试了以下方法但没有成功:
type(randomed_hours) # => list
# Create in Python and transform to RDD
new_col = pd.DataFrame(randomed_hours, columns=['new_col'])
spark_new_col = sqlContext.createDataFrame(new_col)
my_df_spark.withColumn("hours", spark_new_col["new_col"])
使用这个也有错误:
my_df_spark.withColumn("hours", sc.parallelize(randomed_hours))
那么如何使用 PySpark 向现有 DataFrame 添加新列(基于 Python 向量)?
您不能向 Spark 中的DataFrame
添加任意列。 新列只能通过使用文字创建(其他文字类型在如何在 Spark DataFrame 中添加常量列? )
from pyspark.sql.functions import lit
df = sqlContext.createDataFrame(
[(1, "a", 23.0), (3, "B", -23.0)], ("x1", "x2", "x3"))
df_with_x4 = df.withColumn("x4", lit(0))
df_with_x4.show()
## +---+---+-----+---+
## | x1| x2| x3| x4|
## +---+---+-----+---+
## | 1| a| 23.0| 0|
## | 3| B|-23.0| 0|
## +---+---+-----+---+
转换现有列:
from pyspark.sql.functions import exp
df_with_x5 = df_with_x4.withColumn("x5", exp("x3"))
df_with_x5.show()
## +---+---+-----+---+--------------------+
## | x1| x2| x3| x4| x5|
## +---+---+-----+---+--------------------+
## | 1| a| 23.0| 0| 9.744803446248903E9|
## | 3| B|-23.0| 0|1.026187963170189...|
## +---+---+-----+---+--------------------+
包括使用join
:
from pyspark.sql.functions import exp
lookup = sqlContext.createDataFrame([(1, "foo"), (2, "bar")], ("k", "v"))
df_with_x6 = (df_with_x5
.join(lookup, col("x1") == col("k"), "leftouter")
.drop("k")
.withColumnRenamed("v", "x6"))
## +---+---+-----+---+--------------------+----+
## | x1| x2| x3| x4| x5| x6|
## +---+---+-----+---+--------------------+----+
## | 1| a| 23.0| 0| 9.744803446248903E9| foo|
## | 3| B|-23.0| 0|1.026187963170189...|null|
## +---+---+-----+---+--------------------+----+
或使用函数 / udf 生成:
from pyspark.sql.functions import rand
df_with_x7 = df_with_x6.withColumn("x7", rand())
df_with_x7.show()
## +---+---+-----+---+--------------------+----+-------------------+
## | x1| x2| x3| x4| x5| x6| x7|
## +---+---+-----+---+--------------------+----+-------------------+
## | 1| a| 23.0| 0| 9.744803446248903E9| foo|0.41930610446846617|
## | 3| B|-23.0| 0|1.026187963170189...|null|0.37801881545497873|
## +---+---+-----+---+--------------------+----+-------------------+
映射到 Catalyst 表达式的性能方面的内置函数 ( pyspark.sql.functions
) 通常比 Python 用户定义函数更pyspark.sql.functions
。
如果要将任意 RDD 的内容添加为列,您可以
要使用 UDF 添加列:
df = sqlContext.createDataFrame(
[(1, "a", 23.0), (3, "B", -23.0)], ("x1", "x2", "x3"))
from pyspark.sql.functions import udf
from pyspark.sql.types import *
def valueToCategory(value):
if value == 1: return 'cat1'
elif value == 2: return 'cat2'
...
else: return 'n/a'
# NOTE: it seems that calls to udf() must be after SparkContext() is called
udfValueToCategory = udf(valueToCategory, StringType())
df_with_cat = df.withColumn("category", udfValueToCategory("x1"))
df_with_cat.show()
## +---+---+-----+---------+
## | x1| x2| x3| category|
## +---+---+-----+---------+
## | 1| a| 23.0| cat1|
## | 3| B|-23.0| n/a|
## +---+---+-----+---------+
# assumes schema has 'age' column
df.select('*', (df.age + 10).alias('agePlusTen'))
我们可以通过多种方式在 pySpark 中添加新列。
让我们首先创建一个简单的 DataFrame。
date = [27, 28, 29, None, 30, 31]
df = spark.createDataFrame(date, IntegerType())
现在让我们尝试将列值加倍并将其存储在新列中。 PFB 几种不同的方法实现相同。
# Approach - 1 : using withColumn function
df.withColumn("double", df.value * 2).show()
# Approach - 2 : using select with alias function.
df.select("*", (df.value * 2).alias("double")).show()
# Approach - 3 : using selectExpr function with as clause.
df.selectExpr("*", "value * 2 as double").show()
# Approach - 4 : Using as clause in SQL statement.
df.createTempView("temp")
spark.sql("select *, value * 2 as double from temp").show()
有关 spark DataFrame 函数的更多示例和说明,您可以访问我的博客。
我希望这有帮助。
您可以定义一个新的udf
添加时, column_name
:
u_f = F.udf(lambda :yourstring,StringType())
a.select(u_f().alias('column_name')
from pyspark.sql.functions import udf
from pyspark.sql.types import *
func_name = udf(
lambda val: val, # do sth to val
StringType()
)
df.withColumn('new_col', func_name(df.old_col))
我想为一个非常相似的用例提供一个通用示例:
用例:我有一个包含以下内容的 csv:
First|Third|Fifth
data|data|data
data|data|data
...billion more lines
我需要执行一些转换,最终的 csv 需要看起来像
First|Second|Third|Fourth|Fifth
data|null|data|null|data
data|null|data|null|data
...billion more lines
我需要这样做,因为这是由某个模型定义的模式,我需要让我的最终数据与 SQL 批量插入和诸如此类的东西互操作。
所以:
1)我使用 spark.read 读取原始 csv 并将其称为“df”。
2)我对数据做了一些事情。
3)我使用这个脚本添加空列:
outcols = []
for column in MY_COLUMN_LIST:
if column in df.columns:
outcols.append(column)
else:
outcols.append(lit(None).cast(StringType()).alias('{0}'.format(column)))
df = df.select(outcols)
通过这种方式,您可以在加载 csv 后构建您的架构(如果您必须对许多表执行此操作,也可以用于重新排序列)。
添加列的最简单方法是使用“withColumn”。 由于数据框是使用 sqlContext 创建的,因此您必须指定架构或默认情况下可以在数据集中使用。 如果指定了模式,每次更改时工作负载都会变得乏味。
以下是您可以考虑的示例:
from pyspark.sql import SQLContext
from pyspark.sql.types import *
sqlContext = SQLContext(sc) # SparkContext will be sc by default
# Read the dataset of your choice (Already loaded with schema)
Data = sqlContext.read.csv("/path", header = True/False, schema = "infer", sep = "delimiter")
# For instance the data has 30 columns from col1, col2, ... col30. If you want to add a 31st column, you can do so by the following:
Data = Data.withColumn("col31", "Code goes here")
# Check the change
Data.printSchema()
我们可以通过以下步骤直接向 DataFrame 添加额外的列:
from pyspark.sql.functions import when
df = spark.createDataFrame([["amit", 30], ["rohit", 45], ["sameer", 50]], ["name", "age"])
df = df.withColumn("profile", when(df.age >= 40, "Senior").otherwise("Executive"))
df.show()
添加具有一些自定义值或动态值计算的新列,这些计算将基于现有列进行填充。
例如
|ColumnA | ColumnB |
|--------|---------|
| 10 | 15 |
| 10 | 20 |
| 10 | 30 |
和新的 ColumnC 作为 ColumnA+ColumnB
|ColumnA | ColumnB | ColumnC|
|--------|---------|--------|
| 10 | 15 | 25 |
| 10 | 20 | 30 |
| 10 | 30 | 40 |
使用
#to add new column
def customColumnVal(row):
rd=row.asDict()
rd["ColumnC"]=row["ColumnA"] + row["ColumnB"]
new_row=Row(**rd)
return new_row
----------------------------
#convert DF to RDD
df_rdd= input_dataframe.rdd
#apply new fucntion to rdd
output_dataframe=df_rdd.map(customColumnVal).toDF()
input_dataframe
是将被修改的数据帧,并且customColumnVal
函数具有添加新列的代码。
声明:本站的技术帖子网页,遵循CC BY-SA 4.0协议,如果您需要转载,请注明本站网址或者原文地址。任何问题请咨询:yoyou2525@163.com.