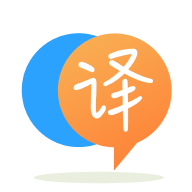
[英]FastText: AttributeError: type object 'FastText' has no attribute 'reduce_model'
[英]Problem retraining a FastText model from .bin file from Fasttext using Gensim. 'FastTextTrainables' object has no attribute 'syn1neg'
我正在尝试使用 gensim 包装器对 FastText 预训练模型进行微调以解决我的问题,但我遇到了问题。 我从 .bin 文件成功加载模型嵌入,如下所示:
from gensim.models.fasttext import FastText
model=FastText.load_fasttext_format(r_bin)
然而,当我想使用这 3 行代码重新训练模型时,我很挣扎:
sent = [['i', 'am ', 'interested', 'on', 'SPGB'], ['SPGB' 'is', 'a', 'good', 'choice']]
model.build_vocab(sent, update=True)
model.train(sentences=sent, total_examples = len(sent), epochs=5)
无论我更改什么,我都会一遍又一遍地收到此错误:
---------------------------------------------------------------------------
AttributeError Traceback (most recent call last)
<ipython-input-91-6456730b1919> in <module>
1 sent = [['i', 'am', 'interested', 'on', 'SPGB'], ['SPGB' 'is', 'a', 'good', 'choice']]
----> 2 model.build_vocab(sent, update=True)
3 model.train(sentences=sent, total_examples = len(sent), epochs=5)
/opt/.../fasttext.py in build_vocab(self, sentences, update, progress_per, keep_raw_vocab, trim_rule, **kwargs)
380 return super(FastText, self).build_vocab(
381 sentences, update=update, progress_per=progress_per,
--> 382 keep_raw_vocab=keep_raw_vocab, trim_rule=trim_rule, **kwargs)
383
384 def _set_train_params(self, **kwargs):
/opt/.../base_any2vec.py in build_vocab(self, sentences, update, progress_per, keep_raw_vocab, trim_rule, **kwargs)
484 trim_rule=trim_rule, **kwargs)
485 report_values['memory'] = self.estimate_memory(vocab_size=report_values['num_retained_words'])
--> 486 self.trainables.prepare_weights(self.hs, self.negative, self.wv, update=update, vocabulary=self.vocabulary)
487
488 def build_vocab_from_freq(self, word_freq, keep_raw_vocab=False, corpus_count=None, trim_rule=None, update=False):
/opt/.../fasttext.py in prepare_weights(self, hs, negative, wv, update, vocabulary)
752
753 def prepare_weights(self, hs, negative, wv, update=False, vocabulary=None):
--> 754 super(FastTextTrainables, self).prepare_weights(hs, negative, wv, update=update, vocabulary=vocabulary)
755 self.init_ngrams_weights(wv, update=update, vocabulary=vocabulary)
756
/opt/.../word2vec.py in prepare_weights(self, hs, negative, wv, update, vocabulary)
1402 self.reset_weights(hs, negative, wv)
1403 else:
-> 1404 self.update_weights(hs, negative, wv)
1405
1406 def seeded_vector(self, seed_string, vector_size):
/opt/.../word2vec.py in update_weights(self, hs, negative, wv)
1452 self.syn1 = vstack([self.syn1, zeros((gained_vocab, self.layer1_size), dtype=REAL)])
1453 if negative:
-> 1454 self.syn1neg = vstack([self.syn1neg, zeros((gained_vocab, self.layer1_size), dtype=REAL)])
1455 wv.vectors_norm = None
1456
AttributeError: 'FastTextTrainables' object has no attribute 'syn1neg'
提前感谢您的帮助
感谢您提供详细的代码,显示您尝试过的内容以及遇到的错误。
您确定您使用的是最新的 Gensim 版本gensim-3.8.3
吗? 我无法使用您的代码和 Gensim 重现错误。
另外:在gensim-3.8.3
您会看到以下效果的警告:
DeprecationWarning: Call to deprecated 'load_fasttext_format' (use load_facebook_vectors (to use pretrained embeddings) or load_facebook_model (to continue training with the loaded full model, more RAM) instead).
(不推荐使用的方法只会为您调用load_facebook_model()
,因此使用旧方法不会单独导致您的问题 - 但您的环境应该使用最新的 Gensim,并且您的代码应该更新以调用首选方法。)
进一步注意:
由于您的小测试文本中没有新词,因此build_vocab(..., update=True)
不是绝对必要的,也不是做任何相关的事情。 模型的已知词汇在前后是相同的。 (当然,如果使用带有新单词的实际新句子,那就不同了——但你的小例子还没有真正测试词汇扩展。)
并进一步:
这种将一些新数据或少量新词训练到现有模型中的方式充满了艰难的权衡。
特别是,如果您的新数据仅包括您的新词和原始模型词的某些子集,则只有这些新数据词将根据其新用法接收训练更新。 这会逐渐将新训练数据中的所有单词拉到新位置。 这些新位置可能会成为新文本的最佳位置,但可能与它们的旧位置相去甚远——也许很远——它们最初是在早期模型中训练的。
因此,您的新词和旧词都不会与新数据中没有的任何旧词保持固有的可比性。 本质上,只有一起训练的单词才必须移动到有用的对比位置。
因此,如果您的新数据足够大且变化多端,足以涵盖您的应用程序所需的单词,那么训练一个全新的模型可能既简单又好。 另一方面,如果您的新数据很薄,那么将一小部分单词/示例训练到旧模型中仍然有可能将这些单词从有用的“对齐”中拉出与旧单词的“对齐”。
声明:本站的技术帖子网页,遵循CC BY-SA 4.0协议,如果您需要转载,请注明本站网址或者原文地址。任何问题请咨询:yoyou2525@163.com.