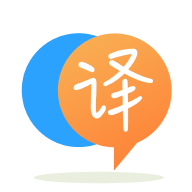
[英]Pandas: How to create a new column in a Dataframe and add values in it considering other existing columns
[英]How to join columns in a pandas dataframe, with empty values, considering priority?
我正在使用 pandas 数据框从事大学工作,如下所示:
import numpy as np
import pandas as pd
df = pd.DataFrame({'col1': [np.NaN, 11.2, 10, np.NaN, 1000],
'sensor_name': ['water', 'strain', 'fog', 'water', 'fog'],
'col2': [np.NaN, np.NaN, 30, 40, np.NaN],
'col3': [np.NaN, 2, 30, 44, np.NaN]
})
print(df)
col1 sensor_name col2 col3
NaN water NaN NaN
11.2 strain NaN 2.0
10.0 fog 30.0 30.0
NaN water 40.0 44.0
1000.0 fog NaN NaN
我想加入第 1、2 和 3 列,以避免 NaN 值。 优先级将是 col1 中的值(如果有)。 那么优先级将是“col2”,最后是“col3”。
我尝试执行以下代码:
df['new_column'] = df.ffill(axis=1)['col3']
输出是:
col1 sensor_name col2 col3 new_column
NaN water NaN NaN water
11.2 strain NaN 2.0 2.0
10.0 fog 30.0 30.0 30.0
NaN water 40.0 44.0 44.0
NaN fog NaN NaN fog
但是,所需的输出是:
col1 sensor_name col2 col3 new_column
NaN water NaN NaN NaN
11.2 strain NaN 2.0 11.2
10.0 fog 30.0 30.0 10.0
NaN water 40.0 44.0 40.0
1000.0 fog NaN NaN 1000.0
您可以实现的众多方法之一是使用pandas.apply
函数。
import numpy as np
import pandas as pd
df = pd.DataFrame({'col1': [np.NaN, 11.2, 10, np.NaN, 1000],
'sensor_name': ['water', 'strain', 'fog', 'water', 'fog'],
'col2': [np.NaN, np.NaN, 30, 40, np.NaN],
'col3': [np.NaN, 2, 30, 44, np.NaN]
})
print(df)
def apply_func(row):
if not pd.isna(row['col1']):
return row['col1']
elif not pd.isna(row['col2']):
return row['col2']
return row["col3"]
df["new_cols"]=df.apply(apply_func,axis=1)
print(df)
输出:
col1 sensor_name col2 col3 new_cols
0 NaN water NaN NaN NaN
1 11.2 strain NaN 2.0 11.2
2 10.0 fog 30.0 30.0 10.0
3 NaN water 40.0 44.0 40.0
4 1000.0 fog NaN NaN 1000.0
过滤列
df['new'] = df.filter(like='col').bfill(1)['col1']
df
Out[324]:
col1 sensor_name col2 col3 new
0 NaN water NaN NaN NaN
1 11.2 strain NaN 2.0 11.2
2 10.0 fog 30.0 30.0 10.0
3 NaN water 40.0 44.0 40.0
4 1000.0 fog NaN NaN 1000.0
尝试这个:
f = df.filter(regex='col\d')
res = df.assign(new_column=f.where(f.notnull().cumsum(axis=1).eq(1)).max(axis=1))
print(res)
>>>
col1 sensor_name col2 col3 new_column
0 NaN water NaN NaN NaN
1 11.2 strain NaN 2.0 11.2
2 10.0 fog 30.0 30.0 10.0
3 NaN water 40.0 44.0 40.0
4 1000.0 fog NaN NaN 1000.0
df['new_column'] = df['col1']
index = df[df['new_column'].isna()].index
df.iloc[index,4]= df.iloc[index,2]
index = df[df['new_column'].isna()].index
df.iloc[index,4]= df.iloc[index,3]
如果您有更多列要考虑和/或希望它更加自动化,您可以创建一个 for 循环。
声明:本站的技术帖子网页,遵循CC BY-SA 4.0协议,如果您需要转载,请注明本站网址或者原文地址。任何问题请咨询:yoyou2525@163.com.