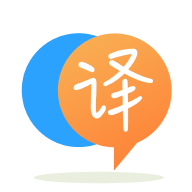
[英]Why my one-filter convolutional neural network is unable to learn a simple gaussian kernel?
[英]Why is a simple 2-layer Neural Network unable to learn 0,0 sequence?
在瀏覽一個小型 2 層神經網絡的例子時,我注意到了我無法解釋的結果。
想象一下,我們有以下帶有相應標簽的數據集:
[0,1] -> [0]
[0,1] -> [0]
[1,0] -> [1]
[1,0] -> [1]
讓我們創建一個很小的 2 層 NN,它將學習預測兩個數字序列的結果,其中每個數字可以是 0 或 1。我們將根據上面提到的數據集訓練這個 NN。
import numpy as np
# compute sigmoid nonlinearity
def sigmoid(x):
output = 1 / (1 + np.exp(-x))
return output
# convert output of sigmoid function to its derivative
def sigmoid_to_deriv(output):
return output * (1 - output)
def predict(inp, weigths):
print inp, sigmoid(np.dot(inp, weigths))
# input dataset
X = np.array([ [0,1],
[0,1],
[1,0],
[1,0]])
# output dataset
Y = np.array([[0,0,1,1]]).T
np.random.seed(1)
# init weights randomly with mean 0
weights0 = 2 * np.random.random((2,1)) - 1
for i in xrange(10000):
# forward propagation
layer0 = X
layer1 = sigmoid(np.dot(layer0, weights0))
# compute the error
layer1_error = layer1 - Y
# gradient descent
# calculate the slope at current x position
layer1_delta = layer1_error * sigmoid_to_deriv(layer1)
weights0_deriv = np.dot(layer0.T, layer1_delta)
# change x by the negative of the slope (x = x - slope)
weights0 -= weights0_deriv
print 'INPUT PREDICTION'
predict([0,1], weights0)
predict([1,0], weights0)
# test prediction of the unknown data
predict([1,1], weights0)
predict([0,0], weights0)
在我們訓練了這個神經網絡之后,我們測試它。
INPUT PREDICTION
[0, 1] [ 0.00881315]
[1, 0] [ 0.99990851]
[1, 1] [ 0.5]
[0, 0] [ 0.5]
好的, 0,1
和1,0
是我們所期望的。 0,0
和1,1
的預測也是可以解釋的,我們的神經網絡沒有這些情況的訓練數據,所以讓我們將其添加到我們的訓練數據集中:
[0,1] -> [0]
[0,1] -> [0]
[1,0] -> [1]
[1,0] -> [1]
[0,0] -> [0]
[1,1] -> [1]
重新訓練網絡並再次測試!
INPUT PREDICTION
[0, 1] [ 0.00881315]
[1, 0] [ 0.99990851]
[1, 1] [ 0.9898148]
[0, 0] [ 0.5]
這意味着 NN仍然不確定0,0
,與在我們訓練它之前不確定1,1
時相同。
分類也是對的。 您需要了解網絡能夠分離測試集。
現在您需要使用階躍函數對0
或1
之間的數據進行分類。
在您的情況下, 0.5
似乎是一個很好的threshold
編輯:
您需要在代碼中添加偏差。
# input dataset
X = np.array([ [0,0,1],
[0,0,1],
[0,1,0],
[0,1,0]])
# init weights randomly with mean 0
weights0 = 2 * np.random.random((3,1)) - 1
聲明:本站的技術帖子網頁,遵循CC BY-SA 4.0協議,如果您需要轉載,請注明本站網址或者原文地址。任何問題請咨詢:yoyou2525@163.com.