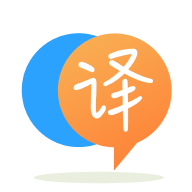
[英]boxplot on groupby timegrouper without subplots using pandas
[英]Pandas timeseries groupby using TimeGrouper
我有一個像這樣的時間序列
Time Demand
Date
2014-01-01 0:00 2899.0
2014-01-01 0:15 2869.0
2014-01-01 0:30 2827.0
2014-01-01 0:45 2787.0
2014-01-01 1:00 2724.0
2014-01-01 1:15 2687.0
2014-01-01 1:30 2596.0
2014-01-01 1:45 2543.0
2014-01-01 2:00 2483.0
它以15分鍾為增量。 我想要每天每一小時的平均值。所以我嘗試了類似df.groupby(pd.TimeGrouper(freq='H')).mean()
。 它的結果不太正確,因為它主要返回了NaNs
。
現在我的數據集具有全年這樣的數據,我想計算所有月份的所有小時的平均值,這樣我可以獲得24點,但是平均值是一年中所有小時的平均值,例如第一個小時得到平均值所有月份的第一個小時。 預期的輸出將是
2014 00:00:00 2884.0
2014 01:00:00 2807.0
2014 02:00:00 2705.5
2014 03:00:00 2569.5
..........
2014 23:00:00 2557.5
我該如何實現?
我認為您需要首先將Time
列添加到index
:
df.index = df.index + pd.to_timedelta(df.Time + ':00')
print (df)
Time Demand
2014-01-01 00:00:00 0:00 2899.0
2014-01-01 00:15:00 0:15 2869.0
2014-01-01 00:30:00 0:30 2827.0
2014-01-01 00:45:00 0:45 2787.0
2014-01-01 01:00:00 1:00 2724.0
2014-01-01 01:15:00 1:15 2687.0
2014-01-01 01:30:00 1:30 2596.0
2014-01-01 01:45:00 1:45 2543.0
2014-01-01 02:00:00 2:00 2483.0
print (df.groupby(pd.Grouper(freq='H')).mean())
#same as
#print (df.groupby(pd.TimeGrouper(freq='H')).mean())
Demand
2014-01-01 00:00:00 2845.5
2014-01-01 01:00:00 2637.5
2014-01-01 02:00:00 2483.0
感謝pansen對另一個想法resample
:
print (df.resample("H").mean())
Demand
2014-01-01 00:00:00 2845.5
2014-01-01 01:00:00 2637.5
2014-01-01 02:00:00 2483.0
編輯:
print (df)
Time Demand
Date
2014-01-01 0:00 1.0
2014-01-01 0:15 2.0
2014-01-01 0:30 4.0
2014-01-01 0:45 5.0
2014-01-01 1:00 1.0
2014-01-01 1:15 0.0
2015-01-01 1:30 1.0
2015-01-01 1:45 2.0
2015-01-01 2:00 3.0
df.index = df.index + pd.to_timedelta(df.Time + ':00')
print (df)
Time Demand
2014-01-01 00:00:00 0:00 1.0
2014-01-01 00:15:00 0:15 2.0
2014-01-01 00:30:00 0:30 4.0
2014-01-01 00:45:00 0:45 5.0
2014-01-01 01:00:00 1:00 1.0
2014-01-01 01:15:00 1:15 0.0
2015-01-01 01:30:00 1:30 1.0
2015-01-01 01:45:00 1:45 2.0
2015-01-01 02:00:00 2:00 3.0
df1 = df.groupby([df.index.year, df.index.hour]).mean().reset_index()
df1.columns = ['year','hour','Demand']
print (df1)
year hour Demand
0 2014 0 3.0
1 2014 1 0.5
2 2015 1 1.5
3 2015 2 3.0
對於DatetimeIndex
使用:
df1 = df.groupby([df.index.year, df.index.hour]).mean()
df1.index = pd.to_datetime(df1.index.get_level_values(0).astype(str) +
df1.index.get_level_values(1).astype(str), format='%Y%H')
print (df1)
Demand
2014-01-01 00:00:00 3.0
2014-01-01 01:00:00 0.5
2015-01-01 01:00:00 1.5
2015-01-01 02:00:00 3.0
聲明:本站的技術帖子網頁,遵循CC BY-SA 4.0協議,如果您需要轉載,請注明本站網址或者原文地址。任何問題請咨詢:yoyou2525@163.com.