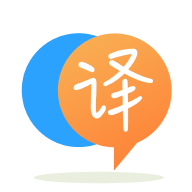
[英]How do i create a function that scans multiple dataframe columns for a value. if any of those values are found the new column returns a given figure
[英]How can I restructure a dataframe to create new column labels based on Column[se] values and then populate those new columns with Column[value] Values
原始數據幀
index Date Device Element Sub_Element Value 179593 2017-11-28 16:39:00 x y eth_txload 9 179594 2017-11-28 16:39:00 x y eth_rxload 30 179595 2017-11-28 16:39:00 x y eth_ip_addr x.x.x.x 179596 2017-11-28 16:39:00 x y description string
期望的數據幀
Date Device Element description eth_txload eth_rxload eth_ip_addr 2017-11-28 16:39:00 x y string 9 30 x.x.x.x
最好的方法是什么?
為每個Sub_Element創建數據幀並合並= ['Date','Device','Element']?
或者使用一些df.iloc魔法來創建一個布爾掩碼並將值應用於新列?
或者也許有一種更好/更有效的方式我會失蹤?
IIUC,給出:
print(df)
index Date Device Element Sub_Element Value
0 179593 2017-11-28 16:39:00 x y eth_txload 9
1 179594 2017-11-28 16:39:00 x y eth_rxload 30
2 179595 2017-11-28 16:39:00 x y eth_ip_addr x.x.x.x
3 179596 2017-11-28 16:39:00 x y description string
然后:
df_out = df.set_index(['Date','Device','Element','Sub_Element'])\
.drop('index',1).unstack()['Value'].reset_index()
print(df_out)
輸出:
Sub_Element Date Device Element description eth_ip_addr eth_rxload eth_txload
0 2017-11-28 16:39:00 x y string x.x.x.x 30 9
我就是這樣做的。 我的解決方案並不像斯科特那樣“花哨”,但我打破了邏輯中的步驟。 對於即插即用場景,他的解決方案可能更好:
#reading in dataframe from your text
df1 = pd.read_clipboard()
# creating an untouched copy of df1 for minpulation
df2 = df1.copy()
# dropping the duplicates of index and Date to get one row
df1 = df1.drop_duplicates(subset=['index', 'Date'])
# creating a dictionary of key, value pairs for each column and value
kv = dict(zip(df2.Sub_Element, df2.Value))
# creating a datframe out of the above dictionary
new_df = pd.DataFrame(kv, index=[0])
# creating temp values to merge on
df1['tmp'] = 1
new_df['tmp'] = 1
# merging on the tmp values
output_df = df1.merge(new_df, on='tmp')
# cleaning up for the output
del output_df['Sub_Element']
del output_df['Value']
del output_df['tmp]
#output
index Date Device Element description eth_ip_addr eth_rxload eth_txload
0 2017-11-28 16:39:00 x y string x.x.x.x 30 9
一個公認的更像SQL的解決方案,但避免處理索引:
# read in the dataframe
df = pd.read_clipboard()
# set up what we will be joining to
anchor = df[['Date','Device','Element']].drop_duplicates()
# loop through the values we want to pivot out
for element in df['Sub_Element'].unique():
# filter the original dataframe for the value for Sub_Element
# using the copy method avoids SettingWithCopyWarning
temp = df[df['Sub_Element']==element].copy()
temp.rename(columns={'Value':element},inplace=True) #rename the header
# left join the new dataframe to the anchor in case of NaNs
anchor = anchor.merge(temp[['Date','Device','Element',element]],
on=['Date','Device','Element'],how='left')
print(anchor)
輸出:
Date Device Element eth_txload eth_rxload eth_ip_addr description
0 2017-11-28 16:39:00 x y 9 30 x.x.x.x string
聲明:本站的技術帖子網頁,遵循CC BY-SA 4.0協議,如果您需要轉載,請注明本站網址或者原文地址。任何問題請咨詢:yoyou2525@163.com.