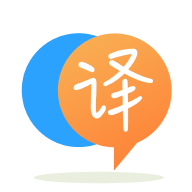
[英]How to sort one column based on another column in Pandas Dataframe?
[英]Interpolate a DataFrame column and sort based on another column in PySpark or Pandas
給定以下DataFrame,我們需要對示例中的my_column
值進行插值, my_column
其用作單獨的列,然后按int_column
對屬於每個some_id
列的int_column
值進行排序。 這個例子:
+--------------------+-----------+------------------+
| some_id | my_column | int_column |
+--------------------+-----------+------------------+
|xx1 |id_1 | 3 |
|xx1 |id_2 | 4 |
|xx1 |id_3 | 5 |
|xx2 |id_1 | 6 |
|xx2 |id_2 | 1 |
|xx2 |id_3 | 3 |
|xx3 |id_1 | 4 |
|xx3 |id_2 | 8 |
|xx3 |id_3 | 9 |
|xx4 |id_1 | 1 |
+--------------------+-----------+------------------+
預期產量:
+--------------------+-----------+------------------+
| id_1 | id_2 | id_3 |
+--------------------+-----------+------------------+
| [xx4, 1] |[xx2, 1] |[xx2, 3] |
| [xx1, 3] |[xx1, 4] |[xx1, 5] |
| [xx3, 4] |[xx3, 8] |[xx3, 9] |
| [xx2, 6] |null |null |
+--------------------+-----------+------------------+
如您所見,對於id_1
, id_1
的最小數字在int_column
是1,它屬於some_id
列中的xx4
,下一個值是3、4和6,每個值分別屬於xx1,xx3和xx2。
關於如何解決這個問題的任何指示? 可以使用PySpark或Pandas。
再現輸入數據幀的代碼:
import pandas as pd
data = {'some_id': ['xx1', 'xx1', 'xx1', 'xx2', 'xx2', 'xx2', 'xx3', 'xx3', 'xx3', 'xx4'], \
'my_column' : ['id_1', 'id_2', 'id_3', 'id_1', 'id_2', 'id_3', 'id_1', 'id_2', 'id_3', 'id_1'],\
'int_column' : [3, 4, 5, 6 , 1, 3, 4, 8, 9, 1]}
df = pd.DataFrame.from_dict(data)
我們需要一個幫助鍵,通過使用cumcount
創建,然后使用groupby
+ apply
(這部分和pivot
crosstab
一樣,或者您可以使用數據pivot_table
crosstab
或crosstab
)
df=df.assign(key=df.groupby('my_column').cumcount())
df.groupby(['key','my_column']).apply(lambda x : list(zip(x['some_id'],x['int_column']))[0]).unstack()
Out[378]:
my_column id_1 id_2 id_3
key
0 (xx1, 3) (xx1, 4) (xx1, 5)
1 (xx2, 6) (xx2, 1) (xx2, 3)
2 (xx3, 4) (xx3, 8) (xx3, 9)
3 (xx4, 1) None None
如果使用pivot
+ sort_values
df=df.sort_values('int_column').assign(key=df.groupby('my_column').cumcount())
df['Value']=list(zip(df['some_id'],df['int_column']))
s=df.pivot(index='key',columns='my_column',values='Value')
s
Out[397]:
my_column id_1 id_2 id_3
key
0 (xx4, 1) (xx2, 1) (xx2, 3)
1 (xx1, 3) (xx1, 4) (xx1, 5)
2 (xx3, 4) (xx3, 8) (xx3, 9)
3 (xx2, 6) None None
這是pyspark中的解決方案。
首先定義一個Window
,按my_column
進行分區, my_column
進行int_column
。 我們將在該分區上使用pyspark.sql.functions.row_number()
定義順序。
from pyspark.sql import Window
import pyspark.sql.functions as f
w = Window.partitionBy("my_column").orderBy("int_column")
df.withColumn("order", f.row_number().over(w)).sort("order").show()
#+-------+---------+----------+-----+
#|some_id|my_column|int_column|order|
#+-------+---------+----------+-----+
#| xx4| id_1| 1| 1|
#| xx2| id_2| 1| 1|
#| xx2| id_3| 3| 1|
#| xx1| id_2| 4| 2|
#| xx1| id_1| 3| 2|
#| xx1| id_3| 5| 2|
#| xx3| id_2| 8| 3|
#| xx3| id_3| 9| 3|
#| xx3| id_1| 4| 3|
#| xx2| id_1| 6| 4|
#+-------+---------+----------+-----+
請注意,按照您的說明, (xx4, 1)
在按order
排序后的第一行中。
現在,您可以按order
分組並在my_column
上pivot
數據my_column
。 這需要一個聚合函數,因此我將使用pyspark.sql.functions.first()
因為我假設每個order
只有一對(some_id, int_column)
對。 然后只需按order
並放下該列即可獲得所需的輸出:
df.withColumn("order", f.row_number().over(w))\
.groupBy("order")\
.pivot("my_column")\
.agg(f.first(f.array([f.col("some_id"), f.col("int_column")])))\
.sort("order")\
.drop("order")\
.show(truncate=False)
#+--------+--------+--------+
#|id_1 |id_2 |id_3 |
#+--------+--------+--------+
#|[xx4, 1]|[xx2, 1]|[xx2, 3]|
#|[xx1, 3]|[xx1, 4]|[xx1, 5]|
#|[xx3, 4]|[xx3, 8]|[xx3, 9]|
#|[xx2, 6]|null |null |
#+--------+--------+--------+
聲明:本站的技術帖子網頁,遵循CC BY-SA 4.0協議,如果您需要轉載,請注明本站網址或者原文地址。任何問題請咨詢:yoyou2525@163.com.