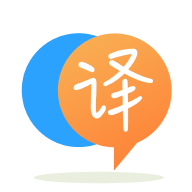
[英]Use Hamming Distance Loss Function with Tensorflow GradientTape: no gradients. Is it not differentiable?
[英]Differentiable Hamming Loss for TensorFlow
漢明損失計算我們的預測錯誤的標簽數量,對其進行歸一化。
作為度量標准的 HammingLoss 實現依賴於計算錯誤的預測,大致如下:(在 TF 上)
count_non_zero = tf.math.count_nonzero(actuals - predictions)
return tf.reduce_mean(count_non_zero / actuals.get_shape()[-1])
將漢明損失實現為實際損失需要它是可微的,由於tf.math.count_nonzero
,情況並非如此。 另一種(和近似的)方法是以這種方式計算非零標簽,但不幸的是,NN 似乎沒有改善。
def hamming_loss(y_true, y_pred):
y_true = tf.convert_to_tensor(y_true, name="y_true")
y_pred = tf.convert_to_tensor(y_pred, name="y_pred")
diff = tf.cast(tf.math.abs(y_true - y_pred), dtype=tf.float32)
#Counting non-zeros in a differentiable way
epsilon = K.epsilon()
nonzero = tf.reduce_mean(tf.math.abs( diff / (tf.math.abs(diff) + epsilon)))
return tf.reduce_mean(nonzero / K.int_shape(y_pred)[-1])
最后,TensorFlow 的漢明損失的正確實現是什么?
您的網絡沒有收斂,因為:
diff / (tf.math.abs(diff) + epsilon)
產生一個0, 1
向量,它會消除零和一上的梯度
聲明:本站的技術帖子網頁,遵循CC BY-SA 4.0協議,如果您需要轉載,請注明本站網址或者原文地址。任何問題請咨詢:yoyou2525@163.com.