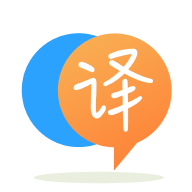
[英]Getting an error with a multi-input Keras model related to the input dimension
[英]Is it possible to extract part of a multi-input model in Keras/TensorFlow?
我有這個多輸入網絡:
def build_model():
inputRGB = tf.keras.Input(shape=(128,128,3), name='train_ds')
inputFixed = tf.keras.Input(shape=(128,128,3), name='fixed_ds')
inputDinamic = tf.keras.Input(shape=(128,128,3), name='dinamic_ds')
# rete per Immagini RGB
rgb = models.Sequential()
rgb = layers.Conv2D(32, (5, 5), padding='SAME')(inputRGB)
rgb = layers.PReLU()(rgb)
rgb = layers.MaxPooling2D((2, 2))(rgb)
rgb = layers.BatchNormalization()(rgb)
rgb = layers.Conv2D(64, (3, 3))(rgb)
rgb = layers.PReLU()(rgb)
rgb = layers.Conv2D(64, (3, 3))(rgb)
rgb = layers.PReLU()(rgb)
rgb = layers.Conv2D(64, (3, 3))(rgb)
rgb = layers.PReLU()(rgb)
rgb = layers.Dropout(0.5)(rgb)
rgb = layers.GlobalAvgPool2D()(rgb)
rgb = Model(inputs = inputRGB, outputs=rgb)
# rete per Density Map con "Pallini"
fixed = models.Sequential()
fixed = layers.Conv2D(32, (5, 5), padding='SAME')(inputFixed)
fixed = layers.PReLU()(fixed)
fixed = layers.MaxPooling2D((2, 2))(fixed)
fixed = layers.BatchNormalization()(fixed)
fixed = layers.Conv2D(64, (3, 3))(fixed)
fixed = layers.PReLU()(fixed)
fixed = layers.Conv2D(64, (3, 3))(fixed)
fixed = layers.PReLU()(fixed)
fixed = layers.Conv2D(64, (3, 3))(fixed)
fixed = layers.PReLU()(fixed)
fixed = layers.Dropout(0.5)(fixed)
fixed = layers.GlobalAvgPool2D()(fixed)
fixed = Model(inputs = inputFixed, outputs=fixed)
# rete per Density map per "assembramenti"
dinamic = models.Sequential()
dinamic = layers.Conv2D(32, (5, 5), padding='SAME')(inputDinamic)
dinamic = layers.PReLU()(dinamic)
dinamic = layers.MaxPooling2D((2, 2))(dinamic)
dinamic = layers.BatchNormalization()(dinamic)
dinamic = layers.Conv2D(64, (3, 3))(dinamic)
dinamic = layers.PReLU()(dinamic)
dinamic = layers.Conv2D(64, (3, 3))(dinamic)
dinamic = layers.PReLU()(dinamic)
dinamic = layers.Conv2D(64, (3, 3))(dinamic)
dinamic = layers.PReLU()(dinamic)
dinamic = layers.Dropout(0.5)(dinamic)
dinamic = layers.GlobalAvgPool2D()(dinamic)
dinamic = Model(inputs = inputDinamic, outputs=dinamic)
concat = layers.concatenate([rgb.output, fixed.output, dinamic.output]) # merge the outputs of the two models
k = layers.Dense(1)(concat)
modelFinal = Model(inputs={'train_ds':inputRGB, 'fixed_ds':inputFixed, 'dinamic_ds':inputDinamic}, outputs=[k])
opt = tf.keras.optimizers.Adam(learning_rate=0.001, amsgrad=False)
modelFinal.compile(optimizer=opt , loss='mae', metrics=['mae'])
return modelFinal
我想從最好的 model 中提取,我已經使用以下代碼行保存並重新加載:
best_model = tf.keras.models.load_model(checkpoint_path + 'regression_count_128.30-1.11.hdf5')
只是前面展示的多輸入神經網絡的第一部分。 具體來說,我想提取將 RGB 圖像作為輸入的部分,以便僅在 RGB 測試圖像上測試 model(使用 3 種不同類型的圖像進行訓練)。
使用輸入層的名稱(即'train_ds'
)和 RGB 部分的 output 層的名稱(您尚未命名,但可以使用best_model.summary()
找到它;它以'global_average_pooling2d'
開頭)像這樣構造一個新的 model :
rgb_model = Model(
best_model.get_layer('train_ds').output,
best_model.get_layer(name_of_rgb_output_layer).output
)
旁注:行... = model.Sequential()
是多余的,可以刪除,因為您正在使用 keras 的功能 API 來定義您的模型。
聲明:本站的技術帖子網頁,遵循CC BY-SA 4.0協議,如果您需要轉載,請注明本站網址或者原文地址。任何問題請咨詢:yoyou2525@163.com.