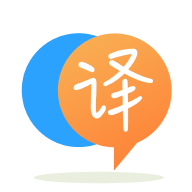
[英]TypeError: 'int' object is not iterable “ What am I doing wrong? ”
[英]"TypeError: 'type' object is not subscriptable" when doing multiprocessing. What am I doing wrong?
我嘗試“多”處理函數func
,但總是收到此錯誤:
File "c:\...programs\python\python37\lib\multiprocessing\pool.py", line 268, in map
return self._map_async(func, iterable, mapstar, chunksize).get()
File "c:\...\programs\python\python37\lib\multiprocessing\pool.py", line 657, in get
raise self._value
TypeError: 'type' object is not subscriptable
我究竟做錯了什么? 每個job
都是一個字典,包含func
所需的所有參數
最小可重復樣本:
import multiprocessing as mp,pandas as pd
def func(name, raw_df=pd.DataFrame, df={}, width=0):
# 3. do some column operations. (actually theres more than just this operation)
seriesF = raw_df[[name]].dropna()
afterDropping_indices = seriesF.index.copy(deep=True)
list_ = list(raw_df[name])[width:]
df[name]=pd.Series(list_.copy(), index=afterDropping_indices[width:])
def preprocess_columns(raw_df ):
# get all inputs.
df, width = {}, 137
args = {"raw_df":raw_df, "df":df, 'width': width }
column_names = raw_df.columns
# get input-dict for every single job.
jobs=[]
for i in range(len(column_names)):
job = {"name":column_names[i]}
job.update(args)
jobs.append(job)
# mutliprocessing
pool = mp.Pool(len(column_names))
pool.map(func, jobs)
# create df from dict and reindex
df=pd.concat(df,axis=1)
df=df.reindex(df.index[::-1])
return df
if __name__=='__main__':
raw_df = pd.DataFrame({"A":[ 1.1 ]*100000, "B":[ 2.2 ]*100000, "C":[ 3.3 ]*100000})
raw_df = preprocess_columns(raw_df )
編輯:僅傳遞列而不是 raw_df 的版本
import multiprocessing as mp,pandas as pd
def func(name, series, df, width):
# 3. do some column operations. (actually theres more than just this operation)
seriesF = series.dropna()
afterDropping_indices = seriesF.index.copy(deep=True)
list_ = list(series)[width:]
df[name]=pd.Series(list_.copy(), index=afterDropping_indices[width:])
def preprocess_columns(raw_df ):
df, width = {}, 137
args = {"df":df, 'width': width }
column_names = raw_df.columns
jobs=[]
for i in range(len(column_names)):
job = {"name":column_names[i], "series":raw_df[column_names[i]]}
job.update(args)
jobs.append(job)
pool = mp.Pool(len(column_names))
pool.map(func, jobs)
# create df from dict and reindex
df=pd.concat(df,axis=1)
df=df.reindex(df.index[::-1])
return df
if __name__=='__main__':
raw_df = pd.DataFrame({"A":[ 1.1 ]*100000, "B":[ 2.2 ]*100000, "C":[ 3.3 ]*100000})
raw_df = preprocess_columns(raw_df )
結果是:
TypeError: func() missing 3 required positional arguments: 'series', 'df', and 'width'
我找到了解決方案:總結:
注意:這只涉及多個線程。
import multiprocessing as mp,pandas as pd
def func(name, raw_df, df, width):
# 3. do some column operations. (actually theres more than just this operation)
seriesF = raw_df[name].dropna()
afterDropping_indices = seriesF.index.copy(deep=True)
list_ = list(raw_df[name])[width:]
df[name]=pd.Series(list_.copy(), index=afterDropping_indices[width:])
df[name].name = name
return df
def expandCall(kargs):
# Expand the arguments of a callback function, kargs[’func’]
func=kargs['func']
del kargs['func']
out=func(**kargs)
return out
def preprocess_columns(raw_df ):
df, width = pd.DataFrame(), 137
args = {"df":df, "raw_df":raw_df, 'width': width }
column_names = raw_df.columns
jobs=[]
for i in range(len(column_names)):
job = {"func":func,"name":column_names[i]}
job.update(args)
jobs.append(job)
pool = mp.Pool(len(column_names))
task=jobs[0]['func'].__name__
outputs= pool.imap_unordered(expandCall, jobs)
out = [];
for i,out_ in enumerate(outputs,1):
out.append(out_)
pool.close(); pool.join() # this is needed to prevent memory leaks return out
# create df from dict and reindex
df=pd.concat(out,axis=1)
df=df.reindex(df.index[::-1])
print(df)
return df
if __name__=='__main__':
raw_df = pd.DataFrame({"A":[ 1.1 ]*100000, "B":[ 2.2 ]*100000, "C":[ 3.3 ]*100000})
raw_df = preprocess_columns(raw_df )
聲明:本站的技術帖子網頁,遵循CC BY-SA 4.0協議,如果您需要轉載,請注明本站網址或者原文地址。任何問題請咨詢:yoyou2525@163.com.