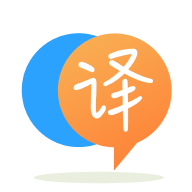
[英]python pandas new column categorization based on conditions in other columns
[英]Priority based categorization using pandas/python
我在下面的數據框和代碼列表中開具發票相關數據
df = pd.DataFrame({
'invoice':[1,1,2,2,2,3,3,3,4,4,4,5,5,6,6,6,7],
'code':[101,104,105,101,106,106,104,101,104,105,111,109,111,110,101,114,112],
'qty':[2,1,1,3,2,4,7,1,1,1,1,4,2,1,2,2,1]
})
+---------+------+-----+
| invoice | code | qty |
+---------+------+-----+
| 1 | 101 | 2 |
+---------+------+-----+
| 1 | 104 | 1 |
+---------+------+-----+
| 2 | 105 | 1 |
+---------+------+-----+
| 2 | 101 | 3 |
+---------+------+-----+
| 2 | 106 | 2 |
+---------+------+-----+
| 3 | 106 | 4 |
+---------+------+-----+
| 3 | 104 | 7 |
+---------+------+-----+
| 3 | 101 | 1 |
+---------+------+-----+
| 4 | 104 | 1 |
+---------+------+-----+
| 4 | 105 | 1 |
+---------+------+-----+
| 4 | 111 | 1 |
+---------+------+-----+
| 5 | 109 | 4 |
+---------+------+-----+
| 5 | 111 | 2 |
+---------+------+-----+
| 6 | 110 | 1 |
+---------+------+-----+
| 6 | 101 | 2 |
+---------+------+-----+
| 6 | 114 | 2 |
+---------+------+-----+
| 7 | 104 | 2 |
+---------+------+-----+
代碼列表是,
Soda = [101,102]
Hot = [103,109]
Juice = [104,105]
Milk = [106,107,108]
Dessert = [110,111]
我的任務是根據下面指定的Order of Priority
添加一個新的category
列。
優先級 1:如果任何發票的數量超過 10 個,則應歸類為Mega
。 例如: invoice 3 is 12
的qty
總和invoice 3 is 12
Priority No.2:來自rest of the invoice
的rest of the invoice
。 如果invoice
任何code
在Milk
列表中,則類別應為Healthy
。 例如: invoice 2
code 106
是Milk
。 因此,完整發票被歸類為Healthy
。 不管發票中是否存在其他項目( code 101 & 105
)。 由於優先級適用於full
發票。
重點三:從rest of the invoice
,如果有的話code
中的invoice
是在Juice
列表中,則這有2 parts
(3.1) 如果果汁數量的總和equal to 1
,則類別應為OneJuice
。 例如: invoice 1
有code 104
和qty 1
無論發票中是否存在其他項目( code 101
),此發票invoice 1
都將獲得OneJuice
。 由於優先級適用於full
發票。
(3.2) 如果果汁數量的總和greater than 1
,則類別應為ManyJuice
。 例如: invoice 4
code 104 & 105
, qty 1 + 1 = 2
。
優先級4:從rest of the invoice
的rest of the invoice
,如果rest of the invoice
任何code
在Hot
列表中,則應將其歸類為HotLovers
。 無論發票中是否存在其他項目。
優先級 5:從rest of the invoice
的rest of the invoice
,如果rest of the invoice
任何code
在Dessert
列表中,則應將其歸類為DessertLovers
。
最后,其余的發票應歸類為Others
。
我想要的輸出如下。
+---------+------+-----+---------------+
| invoice | code | qty | category |
+---------+------+-----+---------------+
| 1 | 101 | 2 | OneJuice |
+---------+------+-----+---------------+
| 1 | 104 | 1 | OneJuice |
+---------+------+-----+---------------+
| 2 | 105 | 1 | Healthy |
+---------+------+-----+---------------+
| 2 | 101 | 3 | Healthy |
+---------+------+-----+---------------+
| 2 | 106 | 2 | Healthy |
+---------+------+-----+---------------+
| 3 | 106 | 4 | Mega |
+---------+------+-----+---------------+
| 3 | 104 | 7 | Mega |
+---------+------+-----+---------------+
| 3 | 101 | 1 | Mega |
+---------+------+-----+---------------+
| 4 | 104 | 1 | ManyJuice |
+---------+------+-----+---------------+
| 4 | 105 | 1 | ManyJuice |
+---------+------+-----+---------------+
| 4 | 111 | 1 | ManyJuice |
+---------+------+-----+---------------+
| 5 | 109 | 4 | HotLovers |
+---------+------+-----+---------------+
| 5 | 111 | 2 | HotLovers |
+---------+------+-----+---------------+
| 6 | 110 | 1 | DessertLovers |
+---------+------+-----+---------------+
| 6 | 101 | 2 | DessertLovers |
+---------+------+-----+---------------+
| 6 | 114 | 2 | DessertLovers |
+---------+------+-----+---------------+
| 7 | 104 | 2 | ManyJuice |
+---------+------+-----+---------------+
到目前為止,我已經在下面嘗試過。 有用。 但非常天真,根本不是pythonic。 同樣,當我將其應用於原始數據集時,代碼非常非常慢。
# Calculating Priority No.1
L = df.groupby(['invoice'])['qty'].transform('sum') >= 10
df_Large = df[L]['invoice'].to_frame()
df_Large['category'] = 'Mega'
df_Large.drop_duplicates(['invoice'], inplace=True)
# Calculating Priority No.2
df_1 = df[~L] # removing Priority No.1 calculated above
M = (df_1['code'].isin(Milk)
.groupby(df_1['invoice'])
.transform('any'))
df_Milk = df_1[M]['invoice'].to_frame()
df_Milk['category'] = 'Healthy'
df_Milk.drop_duplicates(['invoice'], inplace=True)
# Calculating Priority No.3
# 3.a Part -1
df_2 = df[~L & ~M] # removing Priority No.1 & 2 calculated above
J_1 = (df_2['code'].isin(Juice)
.groupby(df_2['invoice'])
.transform('sum') == 1)
df_SM = df_2[J_1]['invoice'].to_frame()
df_SM['category'] = 'OneJuice'
df_SM.drop_duplicates(['invoice'], inplace=True)
# 3.b Part -2
J_2 = (df_2['code'].isin(Juice)
.groupby(df_2['invoice'])
.transform('sum') > 1)
df_MM = df_2[J_2]['invoice'].to_frame()
df_MM['category'] = 'ManyJuice'
df_MM.drop_duplicates(['invoice'], inplace=True)
# Calculating Priority No.4
df_3 = df[~L & ~M & ~J_1 & ~J_2] # removing Priority No.1, 2 & 3 (a & b) calculated above
H = (df_3['code'].isin(Hot)
.groupby(df_3['invoice'])
.transform('any'))
df_Hot = df_3[H]['invoice'].to_frame()
df_Hot['category'] = 'HotLovers'
df_Hot.drop_duplicates(['invoice'], inplace=True)
# Calculating Priority No.5
df_4 = df[~L & ~M & ~J_1 & ~J_2 & ~H ] # removing Priority No.1, 2, 3 (a & b) and 4 calculated above
D = (df_4['code'].isin(Dessert)
.groupby(df_4['invoice'])
.transform('any'))
df_Dessert = df_4[D]['invoice'].to_frame()
df_Dessert['category'] = 'DessertLovers'
df_Dessert.drop_duplicates(['invoice'], inplace=True)
# merge all dfs
category = pd.concat([df_Large,df_Milk,df_SM,df_MM,df_Hot,df_Dessert], axis=0,sort=False, ignore_index=True)
# Final merge to the original dataset
df = df.merge(category,on='invoice', how='left').fillna(value='Others')
因此需要幫助來清理此代碼以提高速度/效率和 pythonic 方式。
你可以嘗試使用np.select
df['category'] = np.select([
df.groupby('invoice')['qty'].transform('sum') >= 10,
df['code'].isin(Milk).groupby(df.invoice).transform('any'),
(df['qty']*df['code'].isin(Juice)).groupby(df.invoice).transform('sum') == 1,
(df['qty']*df['code'].isin(Juice)).groupby(df.invoice).transform('sum') > 1,
df['code'].isin(Hot).groupby(df.invoice).transform('any'),
df['code'].isin(Dessert).groupby(df.invoice).transform('any')
],
['Mega','Healthy','OneJuice','ManyJuice','HotLovers','DessertLovers'],
'Other'
)
print(df)
輸出
invoice code qty category
0 1 101 2 OneJuice
1 1 104 1 OneJuice
2 2 105 1 Healthy
3 2 101 3 Healthy
4 2 106 2 Healthy
5 3 106 4 Mega
6 3 104 7 Mega
7 3 101 1 Mega
8 4 104 1 ManyJuice
9 4 105 1 ManyJuice
10 4 111 1 ManyJuice
11 5 109 4 HotLovers
12 5 111 2 HotLovers
13 6 110 1 DessertLovers
14 6 101 2 DessertLovers
15 6 114 2 DessertLovers
16 7 104 2 ManyJuice
pd.show_versions()
commit : None
python : 3.7.5.final.0
python-bits : 64
OS : Linux
OS-release : 4.4.0-18362-Microsoft
machine : x86_64
processor : x86_64
byteorder : little
LC_ALL : None
LANG : C.UTF-8
LOCALE : en_US.UTF-8
pandas : 0.25.3
numpy : 1.17.4
數據是用
def make_data(n):
return pd.DataFrame({
'invoice':np.arange(n)//3,
'code':np.random.choice(np.arange(101,112),n),
'qty':np.random.choice(np.arange(1,8), n, p=[10/25,10/25,1/25,1/25,1/25,1/25,1/25])
})
結果
perfplot.show(
setup=make_data,
kernels=[get_category, get_with_np_select],
n_range=[2**k for k in range(8, 20)],
logx=True,
logy=True,
equality_check=False,
xlabel='len(df)')
聲明:本站的技術帖子網頁,遵循CC BY-SA 4.0協議,如果您需要轉載,請注明本站網址或者原文地址。任何問題請咨詢:yoyou2525@163.com.