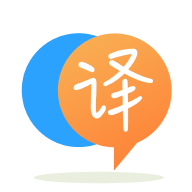
[英]How to loop multiple exposures and outcomes as well as different models with glm in R?
[英]How to loop multiple exposures and outcomes with glm in R?
我有一個循環,目前用於測試多次曝光,結果為 R。
下面的代碼測試結果 y 與 exp1、exp2 和 exp3 的關聯。
我的問題是,測試 y、y1、y2、y3、y4 的相同曝光關聯的最佳/有效方法是什么? 我正在嘗試運行 glm 以獲得多重曝光和多重結果。 而不是我為 5 個結果復制 5 次循環。
# Build data --------------------------------------------------------------
amino_df <- data.frame(y = rbinom(100, 1, 0.5), y2 = rbinom(100, 1, 0.3), y3 = rbinom(100, 1, 0.2), y4 = rbinom(100, 1, 0.22),
exp1 = rnorm(100), exp2 = rnorm(100), exp3 = rnorm(100))
# Observational estimates unadjusted -------------------------------------------------
exp <- c("exp1", "exp2", "exp3")
obs_results <- data.frame()
for (i in seq_along(exp))
{
mod <- as.formula(sprintf("y ~ %s", exp[i]))
glmmodel <- glm(formula = mod, family = binomial, data = amino_df)
obs_results[i,1] <- names(coef(glmmodel))[2]
obs_results[i,2] <- exp(glmmodel$coefficients[2])
obs_results[i,3] <- summary(glmmodel)$coefficients[2,2]
obs_results[i,4] <- summary(glmmodel)$coefficients[2,4]
obs_results[i,5] <- exp(confint.default(glmmodel)[2,1])
obs_results[i,6] <- exp(confint.default(glmmodel)[2,2])
colnames(obs_results) <- c("exposure","OR", "SE", "P_value", "95_CI_LOW","95_CI_HIGH")
}
與 Elena 所做的相同,但使用列表:
exp <- c("exp1", "exp2", "exp3")
y <- c("y","y2","y3")
obs_results <- replicate(length(y), data.frame())
for(j in seq_along(y)){
for (i in seq_along(exp)){
mod <- as.formula(paste(y[j], "~", exp[i]))
glmmodel <- glm(formula = mod, family = binomial, data = amino_df)
obs_results[[j]][i,1] <- names(coef(glmmodel))[2]
obs_results[[j]][i,2] <- exp(glmmodel$coefficients[2])
obs_results[[j]][i,3] <- summary(glmmodel)$coefficients[2,2]
obs_results[[j]][i,4] <- summary(glmmodel)$coefficients[2,4]
obs_results[[j]][i,5] <- exp(confint.default(glmmodel)[2,1])
obs_results[[j]][i,6] <- exp(confint.default(glmmodel)[2,2])
}
colnames(obs_results[[j]]) <- c("exposure","OR", "SE", "P_value", "95_CI_LOW","95_CI_HIGH")
}
names(obs_results) <- y
Output:
> obs_results
$y
exposure OR SE P_value 95_CI_LOW 95_CI_HIGH
1 exp1 0.992145 0.2023656 0.9689149 0.6673001 1.475126
2 exp2 1.064498 0.2107148 0.7667543 0.7043425 1.608812
3 exp3 0.704014 0.2143235 0.1015239 0.4625395 1.071553
$y2
exposure OR SE P_value 95_CI_LOW 95_CI_HIGH
1 exp1 0.9246032 0.2260353 0.7287363 0.5936818 1.439982
2 exp2 0.8905785 0.2347429 0.6215439 0.5621584 1.410866
3 exp3 1.2104091 0.2299170 0.4062258 0.7713056 1.899494
$y3
exposure OR SE P_value 95_CI_LOW 95_CI_HIGH
1 exp1 1.1224366 0.2425520 0.6339361 0.6977522 1.805604
2 exp2 0.9870573 0.2532694 0.9589780 0.6008403 1.621533
3 exp3 0.6854464 0.2582983 0.1436851 0.4131517 1.137201
您可以簡單地圍繞它包裝另一個循環:
exp <- c("exp1", "exp2", "exp3")
ys <- c("y2","y3","y4")
obs_results_total <- data.frame()
obs_results <- data.frame()
for (j in ys){
for (i in seq_along(exp))
{
mod <- as.formula(sprintf("%s ~ %s",j ,exp[i]))
glmmodel <- glm(formula = mod, family = binomial, data = amino_df)
obs_results[i,1] <- names(coef(glmmodel))[2]
obs_results[i,2] <- exp(glmmodel$coefficients[2])
obs_results[i,3] <- summary(glmmodel)$coefficients[2,2]
obs_results[i,4] <- summary(glmmodel)$coefficients[2,4]
obs_results[i,5] <- exp(confint.default(glmmodel)[2,1])
obs_results[i,6] <- exp(confint.default(glmmodel)[2,2])
obs_results[i,7] <- j
colnames(obs_results) <- c("exposure","OR", "SE", "P_value", "95_CI_LOW","95_CI_HIGH","y")
}
obs_results_total <- rbind(obs_results_total,obs_results)
}
聲明:本站的技術帖子網頁,遵循CC BY-SA 4.0協議,如果您需要轉載,請注明本站網址或者原文地址。任何問題請咨詢:yoyou2525@163.com.