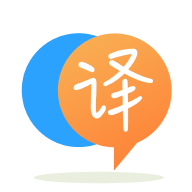
[英]pandas:calculate jaccard similarity for every row based on the value in multiple columns
[英]pandas:calculate jaccard similarity for every row based on the value in another column
我有一個 dataframe 如下,只有更多的行:
import pandas as pd
data = {'First': ['First value', 'Second value','Third value'],
'Second': [['old','new','gold','door'], ['old','view','bold','door'],['new','view','world','window']]}
df = pd.DataFrame (data, columns = ['First','Second'])
為了計算 Jaccard 相似度,我在網上找到了這篇文章(不是我的解決方案):
def lexical_overlap(doc1, doc2):
words_doc1 = set(doc1)
words_doc2 = set(doc2)
intersection = words_doc1.intersection(words_doc2)
union = words_doc1.union(words_doc2)
return float(len(intersection)) / len(union) * 100
因此,我想要得到的結果是度量將第二列的每一行作為 doc,並迭代地比較每一對並輸出具有第一列中行名稱的度量,如下所示:
First value and Second value = 80
First value and Third value = 95
Second value and Third value = 90
由於您的數據不大,您可以嘗試使用稍微不同的方法進行廣播:
# dummy for each rows
s = pd.get_dummies(df.Second.explode()).sum(level=0).values
# pair-wise jaccard
(s@s.T)/(s|s[:,None,:]).sum(-1) * 100
Output:
array([[100. , 33.33333333, 14.28571429],
[ 33.33333333, 100. , 14.28571429],
[ 14.28571429, 14.28571429, 100. ]])
好吧,我會這樣做:
from itertools import combinations
for val in list(combinations(range(len(df)), 2)):
firstlist = df.iloc[val[0],1]
secondlist = df.iloc[val[1],1]
value = round(lexical_overlap(firstlist,secondlist),2)
print(f"{df.iloc[val[0],0]} and {df.iloc[val[1],0]}'s value is: {value}")
Output:
First value and Second value's value is: 33.33
First value and Third value's value is: 14.29
Second value and Third value's value is: 14.29
聲明:本站的技術帖子網頁,遵循CC BY-SA 4.0協議,如果您需要轉載,請注明本站網址或者原文地址。任何問題請咨詢:yoyou2525@163.com.