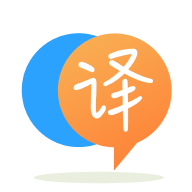
[英]rare misspelled words messes my fastText/Word-Embedding Classfiers
[英]Word-embedding does not provide expected relations between words
我正在嘗試將單詞嵌入訓練到只有主題發生變化的重復句子列表中。 我期望與主題相對應的生成向量在訓練后提供強相關性,正如詞嵌入所期望的那樣。 然而,主題向量之間的角度並不總是大於主題與隨機詞之間的角度。
Man is going to write a very long novel that no one can read.
Woman is going to write a very long novel that no one can read.
Boy is going to write a very long novel that no one can read.
代碼基於pytorch 教程:
import torch
from torch import nn
import torch.nn.functional as F
import numpy as np
class EmbedTrainer(nn.Module):
def __init__(self, d_vocab, d_embed, d_context):
super(EmbedTrainer, self).__init__()
self.embed = nn.Embedding(d_vocab, d_embed)
self.fc_1 = nn.Linear(d_embed * d_context, 128)
self.fc_2 = nn.Linear(128, d_vocab)
def forward(self, x):
x = self.embed(x).view((1, -1)) # flatten after embedding
x = self.fc_2(F.relu(self.fc_1(x)))
x = F.log_softmax(x, dim=1)
return x
text = " ".join(["{} is going to write a very long novel that no one can read.".format(x) for x in ["Man", "Woman", "Boy"]])
text_split = text.split()
trigrams = [([text_split[i], text_split[i+1]], text_split[i+2]) for i in range(len(text_split)-2)]
dic = list(set(text.split()))
tok_to_ids = {w:i for i, w in enumerate(dic)}
tokens_text = text.split(" ")
d_vocab, d_embed, d_context = len(dic), 10, 2
""" Train """
loss_func = nn.NLLLoss()
model = EmbedTrainer(d_vocab, d_embed, d_context)
print(model)
optimizer = torch.optim.SGD(model.parameters(), lr=0.001)
losses = []
epochs = 10
for epoch in range(epochs):
total_loss = 0
for input, target in trigrams:
tok_ids = torch.tensor([tok_to_ids[tok] for tok in input], dtype=torch.long)
target_id = torch.tensor([tok_to_ids[target]], dtype=torch.long)
model.zero_grad()
log_prob = model(tok_ids)
#if total_loss == 0: print("train ", log_prob, target_id)
loss = loss_func(log_prob, target_id)
total_loss += loss.item()
loss.backward()
optimizer.step()
print(total_loss)
losses.append(total_loss)
embed_map = {}
for word in ["Man", "Woman", "Boy", "novel"]:
embed_map[word] = model.embed.weight[tok_to_ids[word]]
print(word, embed_map[word])
def angle(a, b):
from numpy.linalg import norm
a, b = a.detach().numpy(), b.detach().numpy()
return np.dot(a, b) / norm(a) / norm(b)
print("man.woman", angle(embed_map["Man"], embed_map["Woman"]))
print("man.novel", angle(embed_map["Man"], embed_map["novel"]))
我期望與主題對應的生成向量在訓練后提供強相關性,正如詞嵌入所期望的那樣
我真的不認為你會用 3 個句子和 10 個 epoch 中的 40 次迭代(加上你的 40 次迭代中的大部分數據是重復的)來實現那種結果。
也許嘗試在那里下載幾個免費的數據集,或者嘗試使用經過驗證的 model (如 genism model)自己的數據。
我會給你訓練 gensim model 的代碼,這樣你就可以在另一個 model 上測試你的數據集,看看問題是來自你的數據還是來自你的 model。 我已經在包含數百萬個句子的數據集上測試了類似的 gensim 模型,它就像一個魅力,對於較小的數據集,您可能想要更改參數。
from gensim.models import Word2Vec
from multiprocessing import cpu_count
corpus_path = 'eachLineASentence.txt'
vecSize = 300
winSize = 5
numWorkers = cpu_count()-1
epochs = 20
minCount = 5
skipGram = False
modelName = f'mymodel.model'
model = Word2Vec(corpus_file=corpus_path,
size=vecSize,
window=winSize,
min_count=minCount,
workers=numWorkers,
iter=epochs,
sg=skipGram)
model.save(modelName)
PS 我認為在代碼中使用關鍵字輸入作為變量不是一個好主意。
這很可能是訓練規模。 訓練 128d 嵌入絕對是矯枉過正。 來自谷歌開發者博客的經驗法則:
為什么在我們的示例中嵌入向量大小為 3? 好吧,以下“公式”提供了關於嵌入維數的一般經驗法則:
embedding_dimensions = number_of_categories**0.25
也就是說,嵌入向量維度應該是類別數的第 4 根。 因為我們在這個例子中的詞匯量是 81,所以推薦的維數是 3:
3 = 81**0.25
聲明:本站的技術帖子網頁,遵循CC BY-SA 4.0協議,如果您需要轉載,請注明本站網址或者原文地址。任何問題請咨詢:yoyou2525@163.com.