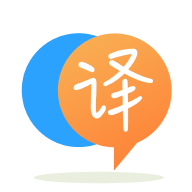
[英]Script Error from “Simple Digit Recognition OCR in OpenCV-Python”
[英]Simple Digit Recognition OCR in OpenCV-Python
我正在嘗試在 OpenCV-Python (cv2) 中實現“數字識別 OCR”。 它僅用於學習目的。 我想學習 OpenCV 中的 KNearest 和 SVM 功能。
我有每個數字的 100 個樣本(即圖像)。 我想和他們一起訓練。
OpenCV 示例附帶了一個示例letter_recog.py
。 但我仍然無法弄清楚如何使用它。 我不明白樣本、響應等是什么。此外,它首先加載了一個 txt 文件,我首先不明白。
后來稍微搜索了一下,我可以在 cpp 樣本中找到一個 letter_recognition.data 。 我使用它並在 letter_recog.py 的模型中為 cv2.KNearest 編寫了代碼(僅用於測試):
import numpy as np
import cv2
fn = 'letter-recognition.data'
a = np.loadtxt(fn, np.float32, delimiter=',', converters={ 0 : lambda ch : ord(ch)-ord('A') })
samples, responses = a[:,1:], a[:,0]
model = cv2.KNearest()
retval = model.train(samples,responses)
retval, results, neigh_resp, dists = model.find_nearest(samples, k = 10)
print results.ravel()
它給了我一個大小為 20000 的數組,我不明白它是什么。
問題:
1) 什么是 letter_recognition.data 文件? 如何從我自己的數據集中構建該文件?
2) results.reval()
表示什么?
3)我們如何使用 letter_recognition.data 文件(KNearest 或 SVM)編寫一個簡單的數字識別工具?
好吧,我決定在我的問題上鍛煉自己來解決上述問題。 我想要的是使用 OpenCV 中的 KNearest 或 SVM 功能實現一個簡單的 OCR。 下面是我做了什么以及如何做的。 (僅用於學習如何將 KNearest 用於簡單的 OCR 目的)。
1)我的第一個問題是關於 OpenCV 樣本附帶的letter_recognition.data
文件。 我想知道那個文件里面有什么。
它包含一個字母,以及該字母的 16 個特征。
而this SOF
幫助我找到了它。 這 16 個特征在論文Letter Recognition Using Holland-Style Adaptive Classifiers
中進行了解釋。 (雖然最后有些功能沒看懂)
2)因為我知道,如果不了解所有這些功能,很難做到這一點。 我嘗試了其他一些論文,但對於初學者來說都有點困難。
所以我決定把所有的像素值作為我的特征。 (我並不擔心准確性或性能,我只是希望它能夠工作,至少准確性最低)
我為我的訓練數據拍攝了下圖:
(我知道訓練數據量較少。但是,由於所有字母的字體和大小相同,我決定嘗試一下)。
為了准備訓練數據,我在 OpenCV 中編寫了一個小代碼。 它執行以下操作:
key press manually
。 這次我們自己按下與框中字母對應的數字鍵。.txt
文件中。 在數字的手動分類結束時,訓練數據( train.png
)中的所有數字都由我們自己手動標記,圖像如下所示:
下面是我用於上述目的的代碼(當然,不是那么干凈):
import sys
import numpy as np
import cv2
im = cv2.imread('pitrain.png')
im3 = im.copy()
gray = cv2.cvtColor(im,cv2.COLOR_BGR2GRAY)
blur = cv2.GaussianBlur(gray,(5,5),0)
thresh = cv2.adaptiveThreshold(blur,255,1,1,11,2)
################# Now finding Contours ###################
contours,hierarchy = cv2.findContours(thresh,cv2.RETR_LIST,cv2.CHAIN_APPROX_SIMPLE)
samples = np.empty((0,100))
responses = []
keys = [i for i in range(48,58)]
for cnt in contours:
if cv2.contourArea(cnt)>50:
[x,y,w,h] = cv2.boundingRect(cnt)
if h>28:
cv2.rectangle(im,(x,y),(x+w,y+h),(0,0,255),2)
roi = thresh[y:y+h,x:x+w]
roismall = cv2.resize(roi,(10,10))
cv2.imshow('norm',im)
key = cv2.waitKey(0)
if key == 27: # (escape to quit)
sys.exit()
elif key in keys:
responses.append(int(chr(key)))
sample = roismall.reshape((1,100))
samples = np.append(samples,sample,0)
responses = np.array(responses,np.float32)
responses = responses.reshape((responses.size,1))
print "training complete"
np.savetxt('generalsamples.data',samples)
np.savetxt('generalresponses.data',responses)
現在我們進入訓練和測試部分。
對於測試部分,我使用了下圖,它的字母類型與我在訓練階段使用的相同。
對於培訓,我們執行以下操作:
.txt
文件出於測試目的,我們執行以下操作:
我在下面的單個代碼中包含了最后兩個步驟(訓練和測試):
import cv2
import numpy as np
####### training part ###############
samples = np.loadtxt('generalsamples.data',np.float32)
responses = np.loadtxt('generalresponses.data',np.float32)
responses = responses.reshape((responses.size,1))
model = cv2.KNearest()
model.train(samples,responses)
############################# testing part #########################
im = cv2.imread('pi.png')
out = np.zeros(im.shape,np.uint8)
gray = cv2.cvtColor(im,cv2.COLOR_BGR2GRAY)
thresh = cv2.adaptiveThreshold(gray,255,1,1,11,2)
contours,hierarchy = cv2.findContours(thresh,cv2.RETR_LIST,cv2.CHAIN_APPROX_SIMPLE)
for cnt in contours:
if cv2.contourArea(cnt)>50:
[x,y,w,h] = cv2.boundingRect(cnt)
if h>28:
cv2.rectangle(im,(x,y),(x+w,y+h),(0,255,0),2)
roi = thresh[y:y+h,x:x+w]
roismall = cv2.resize(roi,(10,10))
roismall = roismall.reshape((1,100))
roismall = np.float32(roismall)
retval, results, neigh_resp, dists = model.find_nearest(roismall, k = 1)
string = str(int((results[0][0])))
cv2.putText(out,string,(x,y+h),0,1,(0,255,0))
cv2.imshow('im',im)
cv2.imshow('out',out)
cv2.waitKey(0)
它奏效了,下面是我得到的結果:
在這里,它以 100% 的准確率工作。 我認為這是因為所有數字都是相同類型和相同大小的。
但無論如何,這對於初學者來說是一個好的開始(我希望如此)。
對 C++ 代碼感興趣的可以參考下面的代碼。 感謝Abid Rahman的精彩解釋。
過程與上述相同,但輪廓查找僅使用第一層級輪廓,因此該算法僅使用每個數字的外輪廓。
//Process image to extract contour
Mat thr,gray,con;
Mat src=imread("digit.png",1);
cvtColor(src,gray,CV_BGR2GRAY);
threshold(gray,thr,200,255,THRESH_BINARY_INV); //Threshold to find contour
thr.copyTo(con);
// Create sample and label data
vector< vector <Point> > contours; // Vector for storing contour
vector< Vec4i > hierarchy;
Mat sample;
Mat response_array;
findContours( con, contours, hierarchy,CV_RETR_CCOMP, CV_CHAIN_APPROX_SIMPLE ); //Find contour
for( int i = 0; i< contours.size(); i=hierarchy[i][0] ) // iterate through first hierarchy level contours
{
Rect r= boundingRect(contours[i]); //Find bounding rect for each contour
rectangle(src,Point(r.x,r.y), Point(r.x+r.width,r.y+r.height), Scalar(0,0,255),2,8,0);
Mat ROI = thr(r); //Crop the image
Mat tmp1, tmp2;
resize(ROI,tmp1, Size(10,10), 0,0,INTER_LINEAR ); //resize to 10X10
tmp1.convertTo(tmp2,CV_32FC1); //convert to float
sample.push_back(tmp2.reshape(1,1)); // Store sample data
imshow("src",src);
int c=waitKey(0); // Read corresponding label for contour from keyoard
c-=0x30; // Convert ascii to intiger value
response_array.push_back(c); // Store label to a mat
rectangle(src,Point(r.x,r.y), Point(r.x+r.width,r.y+r.height), Scalar(0,255,0),2,8,0);
}
// Store the data to file
Mat response,tmp;
tmp=response_array.reshape(1,1); //make continuous
tmp.convertTo(response,CV_32FC1); // Convert to float
FileStorage Data("TrainingData.yml",FileStorage::WRITE); // Store the sample data in a file
Data << "data" << sample;
Data.release();
FileStorage Label("LabelData.yml",FileStorage::WRITE); // Store the label data in a file
Label << "label" << response;
Label.release();
cout<<"Training and Label data created successfully....!! "<<endl;
imshow("src",src);
waitKey();
Mat thr,gray,con;
Mat src=imread("dig.png",1);
cvtColor(src,gray,CV_BGR2GRAY);
threshold(gray,thr,200,255,THRESH_BINARY_INV); // Threshold to create input
thr.copyTo(con);
// Read stored sample and label for training
Mat sample;
Mat response,tmp;
FileStorage Data("TrainingData.yml",FileStorage::READ); // Read traing data to a Mat
Data["data"] >> sample;
Data.release();
FileStorage Label("LabelData.yml",FileStorage::READ); // Read label data to a Mat
Label["label"] >> response;
Label.release();
KNearest knn;
knn.train(sample,response); // Train with sample and responses
cout<<"Training compleated.....!!"<<endl;
vector< vector <Point> > contours; // Vector for storing contour
vector< Vec4i > hierarchy;
//Create input sample by contour finding and cropping
findContours( con, contours, hierarchy,CV_RETR_CCOMP, CV_CHAIN_APPROX_SIMPLE );
Mat dst(src.rows,src.cols,CV_8UC3,Scalar::all(0));
for( int i = 0; i< contours.size(); i=hierarchy[i][0] ) // iterate through each contour for first hierarchy level .
{
Rect r= boundingRect(contours[i]);
Mat ROI = thr(r);
Mat tmp1, tmp2;
resize(ROI,tmp1, Size(10,10), 0,0,INTER_LINEAR );
tmp1.convertTo(tmp2,CV_32FC1);
float p=knn.find_nearest(tmp2.reshape(1,1), 1);
char name[4];
sprintf(name,"%d",(int)p);
putText( dst,name,Point(r.x,r.y+r.height) ,0,1, Scalar(0, 255, 0), 2, 8 );
}
imshow("src",src);
imshow("dst",dst);
imwrite("dest.jpg",dst);
waitKey();
結果第一行中的點被檢測為 8,我們還沒有針對點進行訓練。 此外,我正在考慮將第一層級中的每個輪廓作為樣本輸入,用戶可以通過計算面積來避免它。
如果您對機器學習的最新技術感興趣,您應該研究深度學習。 您應該擁有支持GPU的CUDA,或者在Amazon Web Services上使用GPU。
Google Udacity使用Tensor Flow提供了一個很好的教程。 本教程將教您如何在手寫數字上訓練自己的分類器。 使用Convolutional Networks,我在測試集上獲得了超過97%的准確率。
我在生成訓練數據時遇到了一些問題,因為有時很難識別最后一個選擇的字母,所以我將圖像旋轉了 1.5 度。 現在按順序選擇每個字符,訓練后測試仍然顯示 100% 的准確率。 這是代碼:
import numpy as np
import cv2
def rotate_image(image, angle):
image_center = tuple(np.array(image.shape[1::-1]) / 2)
rot_mat = cv2.getRotationMatrix2D(image_center, angle, 1.0)
result = cv2.warpAffine(image, rot_mat, image.shape[1::-1], flags=cv2.INTER_LINEAR)
return result
img = cv2.imread('training_image.png')
cv2.imshow('orig image', img)
whiteBorder = [255,255,255]
# extend the image border
image1 = cv2.copyMakeBorder(img, 80, 80, 80, 80, cv2.BORDER_CONSTANT, None, whiteBorder)
# rotate the image 1.5 degrees clockwise for ease of data entry
image_rot = rotate_image(image1, -1.5)
#crop_img = image_rot[y:y+h, x:x+w]
cropped = image_rot[70:350, 70:710]
cv2.imwrite('rotated.png', cropped)
cv2.imshow('rotated image', cropped)
cv2.waitKey(0)
對於示例數據,我對腳本進行了一些更改,如下所示:
import sys
import numpy as np
import cv2
def sort_contours(contours, x_axis_sort='LEFT_TO_RIGHT', y_axis_sort='TOP_TO_BOTTOM'):
# initialize the reverse flag
x_reverse = False
y_reverse = False
if x_axis_sort == 'RIGHT_TO_LEFT':
x_reverse = True
if y_axis_sort == 'BOTTOM_TO_TOP':
y_reverse = True
boundingBoxes = [cv2.boundingRect(c) for c in contours]
# sorting on x-axis
sortedByX = zip(*sorted(zip(contours, boundingBoxes),
key=lambda b:b[1][0], reverse=x_reverse))
# sorting on y-axis
(contours, boundingBoxes) = zip(*sorted(zip(*sortedByX),
key=lambda b:b[1][1], reverse=y_reverse))
# return the list of sorted contours and bounding boxes
return (contours, boundingBoxes)
im = cv2.imread('rotated.png')
im3 = im.copy()
gray = cv2.cvtColor(im,cv2.COLOR_BGR2GRAY)
blur = cv2.GaussianBlur(gray,(5,5),0)
thresh = cv2.adaptiveThreshold(blur,255,1,1,11,2)
contours,hierarchy = cv2.findContours(thresh,cv2.RETR_LIST,cv2.CHAIN_APPROX_SIMPLE)
contours, boundingBoxes = sort_contours(contours, x_axis_sort='LEFT_TO_RIGHT', y_axis_sort='TOP_TO_BOTTOM')
samples = np.empty((0,100))
responses = []
keys = [i for i in range(48,58)]
for cnt in contours:
if cv2.contourArea(cnt)>50:
[x,y,w,h] = cv2.boundingRect(cnt)
if h>28 and h < 40:
cv2.rectangle(im,(x,y),(x+w,y+h),(0,0,255),2)
roi = thresh[y:y+h,x:x+w]
roismall = cv2.resize(roi,(10,10))
cv2.imshow('norm',im)
key = cv2.waitKey(0)
if key == 27: # (escape to quit)
sys.exit()
elif key in keys:
responses.append(int(chr(key)))
sample = roismall.reshape((1,100))
samples = np.append(samples,sample,0)
responses = np.array(responses,np.ubyte)
responses = responses.reshape((responses.size,1))
print("training complete")
np.savetxt('generalsamples.data',samples,fmt='%i')
np.savetxt('generalresponses.data',responses,fmt='%i')
聲明:本站的技術帖子網頁,遵循CC BY-SA 4.0協議,如果您需要轉載,請注明本站網址或者原文地址。任何問題請咨詢:yoyou2525@163.com.