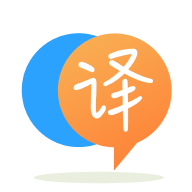
[英]Fail to convert saved EfficientDet model saved_model.pb to tflite format
[英]Error trying to convert from saved model to tflite format
在尝试将保存的模型转换为 tflite 文件时,出现以下错误:
F tensorflow/contrib/lite/toco/tflite/export.cc:363] Some of the
operators in the model are not supported by the standard TensorFlow Lite
runtime. If you have a custom implementation for them you can disable this
error with --allow_custom_ops, or by setting allow_custom_ops=True when
calling tf.contrib.lite.toco_convert(). **Here is a list of operators for
which you will need custom implementations: AsString,
ParseExample**.\nAborted (core dumped)\n'
None
我正在使用 DNN 预制估算器。
from __future__ import absolute_import
from __future__ import division
from __future__ import print_function
import numpy as np
import tensorflow as tf
IRIS_TRAINING = "iris_training.csv"
IRIS_TEST = "iris_test.csv"
INPUT_TENSOR_NAME = 'inputs'
def main():
training_set = tf.contrib.learn.datasets.base.load_csv_with_header(
filename=IRIS_TRAINING,
target_dtype=np.int,
features_dtype=np.float32)
feature_columns = [tf.feature_column.numeric_column(INPUT_TENSOR_NAME, shape=[4])]
# Build 3 layer DNN with 10, 20, 10 units respectively.
classifier = tf.estimator.DNNClassifier(feature_columns=feature_columns,
hidden_units=[10, 20, 10],
n_classes=3,
model_dir="/tmp/iris_model")
# Define the training inputs
train_input_fn = tf.estimator.inputs.numpy_input_fn(
x={INPUT_TENSOR_NAME: np.array(training_set.data)},
y=np.array(training_set.target),
num_epochs=None,
shuffle=True)
# Train model.
classifier.train(input_fn=train_input_fn, steps=2000)
inputs = {'x': tf.placeholder(tf.float32, [4])}
tf.estimator.export.ServingInputReceiver(inputs, inputs)
saved_model=classifier.export_savedmodel(export_dir_base="/tmp/iris_model", serving_input_receiver_fn=serving_input_receiver_fn)
print(saved_model)
converter = tf.contrib.lite.TocoConverter.from_saved_model(saved_model)
tflite_model = converter.convert()
def serving_input_receiver_fn():
feature_spec = {INPUT_TENSOR_NAME: tf.FixedLenFeature(dtype=tf.float32, shape=[4])}
return tf.estimator.export.build_parsing_serving_input_receiver_fn(feature_spec)()
if __name__ == "__main__":
main()
虹膜文件可以从以下链接下载:
IRIS_TRAINING 文件:“ http://download.tensorflow.org/data/iris_training.csv ”
IRIS_TEST 文件:“ http://download.tensorflow.org/data/iris_test.csv ”
ParseExample用于tf.estimator.export.build_parsing_serving_input_receiver_fn
方法。
如果你想避免它,你应该使用tf.estimator.export.build_raw_serving_input_receiver_fn
。
请记住,当您想对结果 SavedModel 进行预测时,您应该设置signature_def_key="predict"
。
所以它看起来像这样predict_fn = predictor.from_saved_model(export_dir='tmp/...', signature_def_key="predict")
声明:本站的技术帖子网页,遵循CC BY-SA 4.0协议,如果您需要转载,请注明本站网址或者原文地址。任何问题请咨询:yoyou2525@163.com.