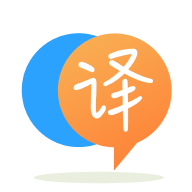
[英]How do I convert my 2D numpy array to a pandas dataframe with given categories?
[英]how do you convert a dataframe into 2d numpy array
I am trying to figure out a way to make a numpy array out of a dataframe so that i can use it as training data for tensorflow this is a function that takes candles for a stock price and makes a dataframe with pandas, now the dataframe values都是浮点数所以数据类型是 float32 如果我错了,请纠正我如何将没有第一行的 output 转换为 numpy 数组以供张量流使用
def some_function(candles):
date_time = []
open_lst = []
high_lst = []
low_lst = []
close_lst = []
volume_lst = []
for item in candles:
#print (item)
t_time = float(item[0])/1000
#print (t_time)
#dt_obj = datetime.fromtimestamp(t_time)
date_time.append(t_time)
#date_time.append(dt_obj)
open_lst.append(float(item[1]))
high_lst.append(float(item[2]))
low_lst.append(float(item[3]))
close_lst.append(float(item[4]))
volume_lst.append(float(item[5]))
## creating data frame
coin_data_frame = {
'date_time' : date_time,
'open' : open_lst,
'high' : high_lst,
'low' : low_lst,
'close' : close_lst,
'volume': volume_lst,
}
df = pd.DataFrame(coin_data_frame , columns = [ 'date_time' , 'open' , 'high' , 'low' , 'close','volume' ])
#print (df.head(5))
### the last 3,5 hours
self.df = df
df['close'] = df[['close']].shift(-15)
df.set_index("date_time", inplace=True)
# graph_df(df.head(10))
print (df.tail(40))
output:
open high low close volume
date_time
1.592598e+09 0.001719 0.001720 0.001718 0.001720 342.21
1.592598e+09 0.001719 0.001719 0.001718 0.001720 1217.08
1.592599e+09 0.001719 0.001719 0.001718 0.001718 237.83
1.592599e+09 0.001719 0.001719 0.001718 0.001718 228.67
1.592599e+09 0.001719 0.001722 0.001718 0.001718 1690.65
1.592600e+09 0.001721 0.001721 0.001719 0.001717 1251.64
1.592600e+09 0.001719 0.001722 0.001719 0.001717 1625.74
1.592600e+09 0.001721 0.001722 0.001720 0.001717 446.60
1.592600e+09 0.001721 0.001721 0.001719 0.001716 372.68
1.592601e+09 0.001720 0.001721 0.001719 0.001718 330.26
1.592601e+09 0.001721 0.001722 0.001721 0.001718 475.65
1.592601e+09 0.001721 0.001722 0.001720 0.001718 406.49
1.592602e+09 0.001721 0.001721 0.001719 0.001719 1013.71
1.592602e+09 0.001720 0.001721 0.001720 0.001720 602.16
1.592602e+09 0.001721 0.001721 0.001720 0.001720 138.23
1.592602e+09 0.001720 0.001721 0.001720 NaN 441.67
1.592603e+09 0.001720 0.001721 0.001719 NaN 100.16
1.592603e+09 0.001721 0.001721 0.001718 NaN 8551.14
1.592603e+09 0.001718 0.001718 0.001716 NaN 28164.34
1.592604e+09 0.001718 0.001719 0.001717 NaN 27695.52
1.592604e+09 0.001718 0.001719 0.001715 NaN 17872.19
1.592604e+09 0.001717 0.001717 0.001715 NaN 8310.23
1.592605e+09 0.001717 0.001717 0.001715 NaN 754.65
1.592605e+09 0.001717 0.001717 0.001716 NaN 695.99
1.592605e+09 0.001716 0.001718 0.001716 NaN 921.44
1.592606e+09 0.001718 0.001719 0.001717 NaN 1474.45
1.592606e+09 0.001718 0.001720 0.001717 NaN 3991.33
1.592606e+09 0.001718 0.001720 0.001717 NaN 457.34
1.592606e+09 0.001719 0.001720 0.001718 NaN 1165.05
1.592607e+09 0.001720 0.001720 0.001718 NaN 1786.93
只需执行df.to_numpy()
为您提供所需的 numpy 数组。 (对于 pandas>=0.24。对于较低版本,等效的是df.values
现在已弃用)
只需确保您事先已将“目标”dataframe 列保存到y
向量中,然后调用df.drop()
将其从 dataframe 中删除,然后再转换为 Z2EA9510C37F7F89E21CB,因此它不会意外馈入您的网络。
此外,这将不包括结果数组中的df.index
列( data_time
)。 我想这是您的预期行为。
声明:本站的技术帖子网页,遵循CC BY-SA 4.0协议,如果您需要转载,请注明本站网址或者原文地址。任何问题请咨询:yoyou2525@163.com.