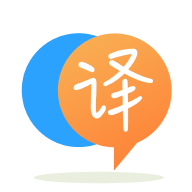
[英]How to calculate dimensions of the dense and output layer in convolutional neural network?
[英]Visualize output of each layer in theano Convolutional MLP
我正在閱讀卷積神經網絡教程 。 我希望在訓練模型后可視化每層的輸出。 例如,在函數“evaluate_lenet5”中,我想將一個實例(它是一個圖像)傳遞給網絡,並查看每個圖層的輸出以及為輸入設置訓練神經網絡的類。 我認為在每個圖層的圖像和權重向量上做點積可能很容易,但它根本不起作用。
我有每個圖層的對象:
# Reshape matrix of rasterized images of shape (batch_size, 28 * 28)
# to a 4D tensor, compatible with our LeNetConvPoolLayer
# (28, 28) is the size of MNIST images.
layer0_input = x.reshape((batch_size, 1, 28, 28))
# Construct the first convolutional pooling layer:
# filtering reduces the image size to (28-5+1 , 28-5+1) = (24, 24)
# maxpooling reduces this further to (24/2, 24/2) = (12, 12)
# 4D output tensor is thus of shape (batch_size, nkerns[0], 12, 12)
layer0 = LeNetConvPoolLayer(
rng,
input=layer0_input,
image_shape=(batch_size, 1, 28, 28),
filter_shape=(nkerns[0], 1, 5, 5),
poolsize=(2, 2)
)
# Construct the second convolutional pooling layer
# filtering reduces the image size to (12-5+1, 12-5+1) = (8, 8)
# maxpooling reduces this further to (8/2, 8/2) = (4, 4)
# 4D output tensor is thus of shape (batch_size, nkerns[1], 4, 4)
layer1 = LeNetConvPoolLayer(
rng,
input=layer0.output,
image_shape=(batch_size, nkerns[0], 12, 12),
filter_shape=(nkerns[1], nkerns[0], 5, 5),
poolsize=(2, 2)
)
# the HiddenLayer being fully-connected, it operates on 2D matrices of
# shape (batch_size, num_pixels) (i.e matrix of rasterized images).
# This will generate a matrix of shape (batch_size, nkerns[1] * 4 * 4),
# or (500, 50 * 4 * 4) = (500, 800) with the default values.
layer2_input = layer1.output.flatten(2)
# construct a fully-connected sigmoidal layer
layer2 = HiddenLayer(
rng,
input=layer2_input,
n_in=nkerns[1] * 4 * 4,
n_out=500,
activation=T.tanh
)
# classify the values of the fully-connected sigmoidal layer
layer3 = LogisticRegression(input=layer2.output, n_in=500, n_out=10)
因此,您可以建議一種方法來在訓練神經網絡后逐步可視化處理圖像的樣本嗎?
這不是那么難。 如果你從theano深度學習教程中使用相同的LeNetConvPoolLayer類定義,那么你只需要編譯一個函數,其中x
作為輸入, [LayerObject].output
作為輸出(其中LayerObject可以是任何層對象,如layer0 , layer1等你想要想象的那個層。
vis_layer1 = function([x],[layer1.output])
傳遞一個(或許多)樣本(確切地說,在訓練時你如何輸入輸入張量),你將獲得為你的函數編譯的特定層的輸出。
注意:這樣你會得到完全相同的形狀模型的計算采用了輸出。 然而,你可以重塑它,只要你想通過整形等的輸出變量layer1.output.flatten(n)
聲明:本站的技術帖子網頁,遵循CC BY-SA 4.0協議,如果您需要轉載,請注明本站網址或者原文地址。任何問題請咨詢:yoyou2525@163.com.