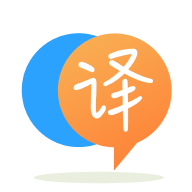
[英]How to use multi-gpu in Keras with shared weights applications model
[英]Keras multi-gpu model fails for a custom model
我有一個在 ImageNet 上訓練的簡單 CNN 模型。 我使用 keras.utils.multi_gpu_model 進行多 GPU 訓練。 它工作正常,但在嘗試訓練基於相同骨干網絡的 SSD 模型時遇到問題。 它在主干頂部有自定義損失和幾個自定義層:
model, predictor_sizes, input_encoder = build_model(input_shape=(args.img_height, args.img_width, 3),
n_classes=num_classes, mode='training')
optimizer = Adam(lr=0.001, beta_1=0.9, beta_2=0.999, epsilon=1e-08, decay=0.0)
loss = SSDMultiBoxLoss(neg_pos_ratio=3, alpha=1.0)
if args.num_gpus > 1:
model = multi_gpu_model(model, gpus=args.num_gpus)
model.compile(optimizer=optimizer, loss=loss.compute_loss)
model.summary()
在num_gpus==1
情況下,我有以下摘要:
__________________________________________________________________________________________________
Layer (type) Output Shape Param # Connected to
==================================================================================================
input_1 (InputLayer) (None, 512, 512, 3) 0
__________________________________________________________________________________________________
conv1_pad (Lambda) (None, 516, 516, 3) 0 input_1[0][0]
__________________________________________________________________________________________________
conv1 (Conv2D) (None, 256, 256, 16) 1216 conv1_pad[0][0]
__________________________________________________________________________________________________
conv1_bn (BatchNormalization) (None, 256, 256, 16) 64 conv1[0][0]
__________________________________________________________________________________________________
conv1_relu (Activation) (None, 256, 256, 16) 0 conv1_bn[0][0]
__________________________________________________________________________________________________
....
det_ctx6_2_mbox_loc_reshape[0][0]
__________________________________________________________________________________________________
mbox_priorbox (Concatenate) (None, None, 8) 0 det_ctx1_2_mbox_priorbox_reshape[
det_ctx2_2_mbox_priorbox_reshape[
det_ctx3_2_mbox_priorbox_reshape[
det_ctx4_2_mbox_priorbox_reshape[
det_ctx5_2_mbox_priorbox_reshape[
det_ctx6_2_mbox_priorbox_reshape[
__________________________________________________________________________________________________
mbox (Concatenate) (None, None, 33) 0 mbox_conf_softmax[0][0]
mbox_loc[0][0]
mbox_priorbox[0][0]
==================================================================================================
Total params: 1,890,510
Trainable params: 1,888,366
Non-trainable params: 2,144
但是,在多 GPU 情況下,我可以看到所有中間層都打包在model
:
__________________________________________________________________________________________________
Layer (type) Output Shape Param # Connected to
==================================================================================================
input_1 (InputLayer) (None, 512, 512, 3) 0
__________________________________________________________________________________________________
lambda (Lambda) (None, 512, 512, 3) 0 input_1[0][0]
__________________________________________________________________________________________________
lambda_1 (Lambda) (None, 512, 512, 3) 0 input_1[0][0]
__________________________________________________________________________________________________
model (Model) (None, None, 33) 1890510 lambda[0][0]
lambda_1[0][0]
__________________________________________________________________________________________________
mbox (Concatenate) (None, None, 33) 0 model[1][0]
model[2][0]
==================================================================================================
Total params: 1,890,510
Trainable params: 1,888,366
Non-trainable params: 2,144
訓練運行正常,但我無法加載以前預訓練的權重:
model.load_weights(args.weights, by_name=True)
因為錯誤:
ValueError: Layer #3 (named "model") expects 150 weight(s), but the saved weights have 68 element(s).
當然,預訓練模型只有主干的權重,而不是對象檢測模型的其余部分。
任何人都可以幫助我理解:
注意:我正在使用 tf.Keras,它現在是 Tensorflow 的一部分。
您可以在構建后立即加載權重,然后再轉換為多 GPU 對應物。 或者,您可以為單 GPU 和多 GPU 版本使用兩個對象,並使用第一個加載權重,然后使用第二個進行訓練。
在編譯您的多 GPU 模型時,嘗試將結果模型返回到一個新的變量,例如“model_multiGPU”,然后在使用您在 multi_gpu_model 函數中輸入的原始模型訓練負載權重后,這將解決問題。
聲明:本站的技術帖子網頁,遵循CC BY-SA 4.0協議,如果您需要轉載,請注明本站網址或者原文地址。任何問題請咨詢:yoyou2525@163.com.