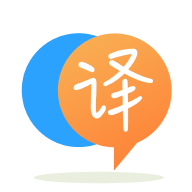
[英]Wav2Vec pytorch element 0 of tensors does not require grad and does not have a grad_fn
[英]element 0 of tensors does not require grad and does not have a grad_fn
我正在嘗試將強化學習機制應用於分類任務。 我知道這是無用的事情,因為深度學習在任務中的表現可能超過 rl。 無論如何,我正在做的研究目的。
如果代理正確為正 1 或不為負 -1,我會獎勵代理,並使用predicted_action(predicted_class)
和獎勵計算損失 FUNC。
但我收到一個錯誤:
張量的元素 0 不需要 grad 並且沒有 grad_fn
# creating model
class Net(nn.Module):
def __init__(self):
super(Net, self).__init__()
self.pipe = nn.Sequential(nn.Linear(9, 120),
nn.ReLU(),
nn.Linear(120, 64),
nn.ReLU(),
nn.Linear(64,2),
nn.Softmax()
)
def forward(self, x):
return self.pipe(x)
def env_step(action, label, size):
total_reward = []
for i in range(size):
reward = 0
if action[i] == label[i]:
total_reward.append(reward+1)
continue
else:
total_reward.append(reward-1)
continue
return total_reward
if __name__=='__main__':
epoch_size = 100
net = Net()
criterion = nn.MSELoss()
optimizer = optim.Adam(params=net.parameters(), lr=0.01)
total_loss = deque(maxlen = 50)
for epoch in range(epoch_size):
batch_index = 0
for i in range(13):
# batch sample
batch_xs = torch.FloatTensor(train_state[batch_index: batch_index+50]) # make tensor
batch_ys = torch.from_numpy(train_label[batch_index: batch_index+50]).type('torch.LongTensor') # make tensor
# action_prob; e.g classification prob
actions_prob = net(batch_xs)
#print(actions_prob)
action = torch.argmax(actions_prob, dim=1).unsqueeze(1)
#print(action)
reward = np.array(env_step(action, batch_ys, 50))
#print(reward)
reward = torch.from_numpy(reward).unsqueeze(1).type('torch.FloatTensor')
#print(reward)
action = action.type('torch.FloatTensor')
optimizer.zero_grad()
loss = criterion(action, reward)
loss.backward()
optimizer.step()
batch_index += 50
action
是由 argmax 函數產生的,它是不可微的。 相反,您希望在所采取的行動的獎勵和負責任的概率之間承擔損失。
通常,為強化學習中的策略選擇的“損失”是所謂的評分函數:
這是在日志中行動負責概率看起來的產品a
采取倍獎勵獲得。
聲明:本站的技術帖子網頁,遵循CC BY-SA 4.0協議,如果您需要轉載,請注明本站網址或者原文地址。任何問題請咨詢:yoyou2525@163.com.