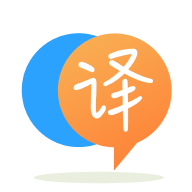
[英]Copernicus Dem xarray select nearest lat/lon with multi-dimension coordinates
[英]xarray select nearest lat/lon with multi-dimension coordinates
我有一個帶有不規則間隔緯度和經度坐標的 xarray 數據集。 我的目標是在最接近某個緯度/經度的點處找到變量的值。
由於x
和y
維度不是 lat/lon 值,因此在這種情況下似乎不能單獨使用ds.sel()
方法。 是否有以 xarray 為中心的方法通過參考多維緯度/經度維度來定位最接近所需緯度/經度的點? 例如,我想提取最接近lat=21.2
和lon=-122.68
的 SPEED 值。
下面是一個示例數據集...
lats = np.array([[21.138 , 21.14499, 21.15197, 21.15894, 21.16591],
[21.16287, 21.16986, 21.17684, 21.18382, 21.19079],
[21.18775, 21.19474, 21.20172, 21.2087 , 21.21568],
[21.21262, 21.21962, 21.22661, 21.23359, 21.24056],
[21.2375 , 21.2445 , 21.25149, 21.25848, 21.26545]])
lons = np.array([[-122.72 , -122.69333, -122.66666, -122.63999, -122.61331],
[-122.7275 , -122.70082, -122.67415, -122.64746, -122.62078],
[-122.735 , -122.70832, -122.68163, -122.65494, -122.62825],
[-122.7425 , -122.71582, -122.68912, -122.66243, -122.63573],
[-122.75001, -122.72332, -122.69662, -122.66992, -122.64321]])
speed = np.array([[10.934007, 10.941321, 10.991583, 11.063932, 11.159435],
[10.98778 , 10.975482, 10.990983, 11.042522, 11.131154],
[11.013505, 11.001573, 10.997754, 11.03566 , 11.123781],
[11.011163, 11.000227, 11.010223, 11.049 , 11.1449 ],
[11.015698, 11.026604, 11.030653, 11.076904, 11.201464]])
ds = xarray.Dataset({'SPEED':(('x', 'y'),speed)},
coords = {'latitude': (('x', 'y'), lats),
'longitude': (('x', 'y'), lons)},
attrs={'variable':'Wind Speed'})
ds
的值:
<xarray.Dataset>
Dimensions: (x: 5, y: 5)
Coordinates:
latitude (x, y) float64 21.14 21.14 21.15 21.16 ... 21.25 21.26 21.27
longitude (x, y) float64 -122.7 -122.7 -122.7 ... -122.7 -122.7 -122.6
Dimensions without coordinates: x, y
Data variables:
SPEED (x, y) float64 10.93 10.94 10.99 11.06 ... 11.03 11.03 11.08 11.2
Attributes:
variable: Wind Speed
同樣, ds.sel(latitude=21.2, longitude=-122.68)
不起作用,因為緯度和經度不是數據集維度。
在這里聚會有點晚了,但我已經多次回到這個問題。 如果您的 x 和 y 坐標位於地理空間坐標系中,則可以使用 cartopy 將 lat/lon 點轉換為該坐標系。 如果您查看 netcdf 中的元數據,構建 cartopy 投影通常很簡單。
import cartopy.crs as ccrs
# Example - your x and y coordinates are in a Lambert Conformal projection
data_crs = ccrs.LambertConformal(central_longitude=-100)
# Transform the point - src_crs is always Plate Carree for lat/lon grid
x, y = data_crs.transform_point(-122.68, 21.2, src_crs=ccrs.PlateCarree())
# Now you can select data
ds.sel(x=x, y=y)
我想出了一個不純粹使用 xarray 的方法。 我首先手動找到最近鄰居的索引,然后使用該索引訪問 xarray 維度。
# A 2D plot of the SPEED variable, assigning the coordinate values,
# and plot the verticies of each point
ds.SPEED.plot(x='longitude', y='latitude')
plt.scatter(ds.longitude, ds.latitude)
# I want to find the speed at a certain lat/lon point.
lat = 21.22
lon = -122.68
# First, find the index of the grid point nearest a specific lat/lon.
abslat = np.abs(ds.latitude-lat)
abslon = np.abs(ds.longitude-lon)
c = np.maximum(abslon, abslat)
([xloc], [yloc]) = np.where(c == np.min(c))
# Now I can use that index location to get the values at the x/y diminsion
point_ds = ds.sel(x=xloc, y=yloc)
# Plot requested lat/lon point blue
plt.scatter(lon, lat, color='b')
plt.text(lon, lat, 'requested')
# Plot nearest point in the array red
plt.scatter(point_ds.longitude, point_ds.latitude, color='r')
plt.text(point_ds.longitude, point_ds.latitude, 'nearest')
plt.title('speed at nearest point: %s' % point_ds.SPEED.data)
另一個潛在的解決方案(同樣,不是 xarray)是使用 scipy 的 KDTree,或者更好的是 scikit-learn 的 BallTree( https://scikit-learn.org/stable/modules/generated/sklearn.neighbors.BallTree.ZFC35FDC70D5FC69D269883A822CA ) https://github.com/xarray-contrib/xoak 。
我認為您需要以不同的方式創建數據集,以確保latitude
和longitude
具有可解釋的維度(請參閱xarray 的基本數據結構一文)。
例如:
import numpy as np
import pandas as pd
import xarray
import matplotlib.pyplot as plt
from scipy.interpolate import griddata
lats = np.array([21.138, 21.14499, 21.15197, 21.15894, 21.16591,
21.16287, 21.16986, 21.17684, 21.18382, 21.19079,
21.18775, 21.19474, 21.20172, 21.2087, 21.21568,
21.21262, 21.21962, 21.22661, 21.23359, 21.24056,
21.2375, 21.2445, 21.25149, 21.25848, 21.26545])
lons = np.array([-122.72, -122.69333, -122.66666, -122.63999, -122.61331,
-122.7275, -122.70082, -122.67415, -122.64746, -122.62078,
-122.735, -122.70832, -122.68163, -122.65494, -122.62825,
-122.7425, -122.71582, -122.68912, -122.66243, -122.63573,
-122.75001, -122.72332, -122.69662, -122.66992, -122.64321])
speed = np.array([10.934007, 10.941321, 10.991583, 11.063932, 11.159435,
10.98778, 10.975482, 10.990983, 11.042522, 11.131154,
11.013505, 11.001573, 10.997754, 11.03566, 11.123781,
11.011163, 11.000227, 11.010223, 11.049, 11.1449,
11.015698, 11.026604, 11.030653, 11.076904, 11.201464])
fig, (ax1, ax2) = plt.subplots(ncols=2, figsize=(12, 5))
idx = pd.MultiIndex.from_arrays(arrays=[lons, lats], names=["lon", "lat"])
s = pd.Series(data=speed, index=idx)
da = xarray.DataArray.from_series(s)
print(da)
da.plot(ax=ax1)
print('-'*80)
print(da.sel(lat=21.2, lon=-122.68, method='nearest'))
# define grid.
num_points = 100
lats_i = np.linspace(np.min(lats), np.max(lats), num_points)
lons_i = np.linspace(np.min(lons), np.max(lons), num_points)
# grid the data.
speed_i = griddata((lats, lons), speed,
(lats_i[None, :], lons_i[:, None]), method='cubic')
# contour the gridded data
ax2.contour(lats_i, lons_i, speed_i, 15, linewidths=0.5, colors='k')
contour = ax2.contourf(lats_i, lons_i, speed_i, 15, cmap=plt.cm.jet)
plt.colorbar(contour, ax=ax2)
# plot data points.
for i, (lat, lon) in enumerate(zip(lats, lons)):
label = f'{speed[i]:0.2f}'
ax2.annotate(label, (lat, lon))
ax2.scatter(lats, lons, marker='o', c='b', s=5)
ax2.set_title(f'griddata test {num_points} points')
plt.subplots_adjust(wspace=0.2)
plt.show()
結果
<xarray.DataArray (lat: 25, lon: 25)>
array([[ nan, nan, nan, nan, nan, 10.934007,
nan, nan, nan, nan, nan, nan,
nan, nan, nan, nan, nan, nan,
nan, nan, nan, nan, nan, nan,
nan],
[ nan, nan, nan, nan, nan, nan,
nan, nan, nan, nan, 10.941321, nan,
nan, nan, nan, nan, nan, nan,
nan, nan, nan, nan, nan, nan,
nan],
[ nan, nan, nan, nan, nan, nan,
nan, nan, nan, nan, nan, nan,
nan, nan, nan, 10.991583, nan, nan,
nan, nan, nan, nan, nan, nan,
nan],
[ nan, nan, nan, nan, nan, nan,
nan, nan, nan, nan, nan, nan,
nan, nan, nan, nan, nan, nan,
nan, nan, 11.063932, nan, nan, nan,
nan],
[ nan, nan, nan, 10.98778 , nan, nan,
nan, nan, nan, nan, nan, nan,
nan, nan, nan, nan, nan, nan,
nan, nan, nan, nan, nan, nan,
nan],
[ nan, nan, nan, nan, nan, nan,
nan, nan, nan, nan, nan, nan,
nan, nan, nan, nan, nan, nan,
nan, nan, nan, nan, nan, nan,
11.159435],
[ nan, nan, nan, nan, nan, nan,
nan, nan, 10.975482, nan, nan, nan,
nan, nan, nan, nan, nan, nan,
nan, nan, nan, nan, nan, nan,
nan],
[ nan, nan, nan, nan, nan, nan,
nan, nan, nan, nan, nan, nan,
nan, 10.990983, nan, nan, nan, nan,
nan, nan, nan, nan, nan, nan,
nan],
[ nan, nan, nan, nan, nan, nan,
nan, nan, nan, nan, nan, nan,
nan, nan, nan, nan, nan, nan,
11.042522, nan, nan, nan, nan, nan,
nan],
[ nan, nan, 11.013505, nan, nan, nan,
nan, nan, nan, nan, nan, nan,
nan, nan, nan, nan, nan, nan,
nan, nan, nan, nan, nan, nan,
nan],
[ nan, nan, nan, nan, nan, nan,
nan, nan, nan, nan, nan, nan,
nan, nan, nan, nan, nan, nan,
nan, nan, nan, nan, nan, 11.131154,
nan],
[ nan, nan, nan, nan, nan, nan,
nan, 11.001573, nan, nan, nan, nan,
nan, nan, nan, nan, nan, nan,
nan, nan, nan, nan, nan, nan,
nan],
[ nan, nan, nan, nan, nan, nan,
nan, nan, nan, nan, nan, nan,
10.997754, nan, nan, nan, nan, nan,
nan, nan, nan, nan, nan, nan,
nan],
[ nan, nan, nan, nan, nan, nan,
nan, nan, nan, nan, nan, nan,
nan, nan, nan, nan, nan, 11.03566 ,
nan, nan, nan, nan, nan, nan,
nan],
[ nan, 11.011163, nan, nan, nan, nan,
nan, nan, nan, nan, nan, nan,
nan, nan, nan, nan, nan, nan,
nan, nan, nan, nan, nan, nan,
nan],
[ nan, nan, nan, nan, nan, nan,
nan, nan, nan, nan, nan, nan,
nan, nan, nan, nan, nan, nan,
nan, nan, nan, nan, 11.123781, nan,
nan],
[ nan, nan, nan, nan, nan, nan,
11.000227, nan, nan, nan, nan, nan,
nan, nan, nan, nan, nan, nan,
nan, nan, nan, nan, nan, nan,
nan],
[ nan, nan, nan, nan, nan, nan,
nan, nan, nan, nan, nan, 11.010223,
nan, nan, nan, nan, nan, nan,
nan, nan, nan, nan, nan, nan,
nan],
[ nan, nan, nan, nan, nan, nan,
nan, nan, nan, nan, nan, nan,
nan, nan, nan, nan, 11.049 , nan,
nan, nan, nan, nan, nan, nan,
nan],
[11.015698, nan, nan, nan, nan, nan,
nan, nan, nan, nan, nan, nan,
nan, nan, nan, nan, nan, nan,
nan, nan, nan, nan, nan, nan,
nan],
[ nan, nan, nan, nan, nan, nan,
nan, nan, nan, nan, nan, nan,
nan, nan, nan, nan, nan, nan,
nan, nan, nan, 11.1449 , nan, nan,
nan],
[ nan, nan, nan, nan, 11.026604, nan,
nan, nan, nan, nan, nan, nan,
nan, nan, nan, nan, nan, nan,
nan, nan, nan, nan, nan, nan,
nan],
[ nan, nan, nan, nan, nan, nan,
nan, nan, nan, 11.030653, nan, nan,
nan, nan, nan, nan, nan, nan,
nan, nan, nan, nan, nan, nan,
nan],
[ nan, nan, nan, nan, nan, nan,
nan, nan, nan, nan, nan, nan,
nan, nan, 11.076904, nan, nan, nan,
nan, nan, nan, nan, nan, nan,
nan],
[ nan, nan, nan, nan, nan, nan,
nan, nan, nan, nan, nan, nan,
nan, nan, nan, nan, nan, nan,
nan, 11.201464, nan, nan, nan, nan,
nan]])
Coordinates:
* lat (lat) float64 21.14 21.14 21.15 21.16 ... 21.24 21.25 21.26 21.27
* lon (lon) float64 -122.8 -122.7 -122.7 -122.7 ... -122.6 -122.6 -122.6
--------------------------------------------------------------------------------
<xarray.DataArray ()>
array(10.997754)
Coordinates:
lat float64 21.2
lon float64 -122.7
我喜歡@blaylockbk 給出的答案,但我無法理解計算到數據點的最短距離的方式。 下面我提供了一個替代方案,它只是利用畢達哥拉斯加上一種網格數據集ds
的方法。 為了不將數據集中的 (x, y) 與 x, y 大地坐標混淆,我將它們重命名為 (i, j)。
import numpy as np
import xarray
import matplotlib.pyplot as plt
from scipy.interpolate import griddata
lats = np.array([[21.138, 21.14499, 21.15197, 21.15894, 21.16591],
[21.16287, 21.16986, 21.17684, 21.18382, 21.19079],
[21.18775, 21.19474, 21.20172, 21.2087, 21.21568],
[21.21262, 21.21962, 21.22661, 21.23359, 21.24056],
[21.2375, 21.2445, 21.25149, 21.25848, 21.26545]])
lons = np.array([[-122.72, -122.69333, -122.66666, -122.63999, -122.61331],
[-122.7275, -122.70082, -122.67415, -122.64746, -122.62078],
[-122.735, -122.70832, -122.68163, -122.65494, -122.62825],
[-122.7425, -122.71582, -122.68912, -122.66243, -122.63573],
[-122.75001, -122.72332, -122.69662, -122.66992, -122.64321]])
speed = np.array([[10.934007, 10.941321, 10.991583, 11.063932, 11.159435],
[10.98778, 10.975482, 10.990983, 11.042522, 11.131154],
[11.013505, 11.001573, 10.997754, 11.03566, 11.123781],
[11.011163, 11.000227, 11.010223, 11.049, 11.1449],
[11.015698, 11.026604, 11.030653, 11.076904, 11.201464]])
ds = xarray.Dataset({'SPEED': (('i', 'j'), speed)},
coords={'latitude': (('i', 'j'), lats),
'longitude': (('i', 'j'), lons)},
attrs={'variable': 'Wind Speed'})
lat_min = float(np.min(ds.latitude))
lat_max = float(np.max(ds.latitude))
lon_min = float(np.min(ds.longitude))
lon_max = float(np.max(ds.longitude))
margin = 0.02
fig, ((ax1, ax2)) = plt.subplots(nrows=1, ncols=2, figsize=(12, 5))
ax1.set_xlim(lat_min - margin, lat_max + margin)
ax1.set_ylim(lon_min - margin, lon_max + margin)
ax1.axis('equal')
ds.SPEED.plot(ax=ax1, x='latitude', y='longitude', cmap=plt.cm.jet)
ax1.scatter(ds.latitude, ds.longitude, color='black')
# find nearest_point for a requested lat/ lon
lat_requested = 21.22
lon_requested = -122.68
d_lat = ds.latitude - lat_requested
d_lon = ds.longitude - lon_requested
r2_requested = d_lat**2 + d_lon**2
i_j_loc = np.where(r2_requested == np.min(r2_requested))
nearest_point = ds.sel(i=i_j_loc[0], j=i_j_loc[1])
# Plot nearest point in the array red# Plot nearest point in the array red
ax1.scatter(lat_requested, lon_requested, color='green')
ax1.text(lat_requested, lon_requested, 'requested')
ax1.scatter(nearest_point.latitude, nearest_point.longitude, color='red')
ax1.text(nearest_point.latitude, nearest_point.longitude, 'nearest')
ax1.set_title(f'speed at nearest point: {float(nearest_point.SPEED.data):.2f}')
# define grid from the dataset
num_points = 100
lats_i = np.linspace(lat_min, lat_max, num_points)
lons_i = np.linspace(lon_min, lon_max, num_points)
# grid and contour the data.
speed_i = griddata((ds.latitude.values.flatten(), ds.longitude.values.flatten()),
ds.SPEED.values.flatten(),
(lats_i[None, :], lons_i[:, None]), method='cubic')
ax2.set_xlim(lat_min - margin, lat_max + margin)
ax2.set_ylim(lon_min - margin, lon_max + margin)
ax2.axis('equal')
ax2.set_title(f'griddata test {num_points} points')
ax2.contour(lats_i, lons_i, speed_i, 15, linewidths=0.5, colors='k')
contour = ax2.contourf(lats_i, lons_i, speed_i, 15, cmap=plt.cm.jet)
plt.colorbar(contour, ax=ax2)
# plot data points and labels
ax2.scatter(ds.latitude, ds.longitude, marker='o', c='b', s=5)
for i, (lat, lon) in enumerate(zip(ds.latitude.values.flatten(),
ds.longitude.values.flatten())):
text_label = f'{ds.SPEED.values.flatten()[i]:0.2f}'
ax2.text(lat, lon, text_label)
# Plot nearest point in the array red
ax2.scatter(lat_requested, lon_requested, color='green')
ax2.text(lat_requested, lon_requested, 'requested')
ax2.scatter(nearest_point.latitude, nearest_point.longitude, color='red')
plt.subplots_adjust(wspace=0.2)
plt.show()
如另一個答案中所述,要基於此數據格式的投影進行查找,不幸的是,您必須將投影信息添加回數據中。
import cartopy.crs as ccrs
# Projection may vary
projection = ccrs.LambertConformal(central_longitude=-97.5,
central_latitude=38.5,
standard_parallels=[38.5])
transform = np.vectorize(lambda x, y: projection.transform_point(x, y, ccrs.PlateCarree()))
# The grid should be aligned such that the projection x and y are the same
# at every y and x index respectively
grid_y = ds.isel(x=0)
grid_x = ds.isel(y=0)
_, proj_y = transform(grid_y.longitude, grid_y.latitude)
proj_x, _ = transform(grid_x.longitude, grid_x.latitude)
# ds.sel only works on the dimensions, so we can't just add
# proj_x and proj_y as additional coordinate variables
ds["x"] = proj_x
ds["y"] = proj_y
desired_x, desired_y = transform(-122.68, 21.2)
nearest_point = ds.sel(x=desired_x, y=desired_y, method="nearest")
print(nearest_point.SPEED)
Output:
<xarray.DataArray 'SPEED' ()>
array(10.934007)
Coordinates:
latitude float64 21.14
longitude float64 -122.7
x float64 -2.701e+06
y float64 -1.581e+06
只是一個評論和一些運行時:
對於 5000 × 5000 個數據點,每個查詢占用的時間和空間與 2500 萬個成正比。 以下,我認為相當於你的代碼,在我的舊 2.7 GHz iMac 上每次查詢大約需要 1 秒:
import sys
import numpy as np
from scipy.spatial.distance import cdist
n = 5000
nask = 1
dim = 2
# to change these params, run this.py a=1 b=None 'c = expr' ... in sh or ipython --
for arg in sys.argv[1:]:
exec( arg )
rng = np.random.default_rng( seed=0 )
X = rng.uniform( -100, 100, size=(n*n, dim) ) # data, n^2 × 2
ask = rng.uniform( -100, 100, size=(nask, dim) ) # query points
dist = cdist( X, ask, "chebyshev" ) # -> n^2 × nask
# 1d index -> 2d index, e.g. 60003 -> row 12, col 3
jminflat = dist[:,0].argmin()
jmin = np.unravel_index( jminflat, (n,n) )
print( "cdist N %g dim %d ask %s: dist %.2g to X[%s] = %s " % (
n*n, dim, ask[0], dist[jminflat], jmin, X[jminflat] ))
# cdist N 25000000 dim 2 ask [-4.6 94]: dist 0.0079 to X[(4070, 2530)] = [-4.6 94]
作為比較, scipy KDTree需要約 30 秒來為 25M 2d 點構建樹,然后每個查詢需要毫秒。 優點:輸入點可以任意散布,找到 5 或 10 個最近鄰進行插值不會比 1 長多少。
另見: scipy cdist
gis.stack 上的重新項目匹配和 interp 之間的差異
最近鄰搜索...
聲明:本站的技術帖子網頁,遵循CC BY-SA 4.0協議,如果您需要轉載,請注明本站網址或者原文地址。任何問題請咨詢:yoyou2525@163.com.