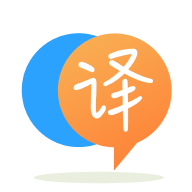
[英]Data Augmentation using ImageDataGenerator in keras-python
[英]Data augmentation with ImageDataGenerator for videos (4D tensors) in Keras
我在 Keras 中有一個ImageDataGenerator
,我想在訓練期間將其應用於短視頻剪輯中的每一幀,這些短視頻剪輯表示為 4D numpy arrays,寬度為,num_frames 3
對於由每個具有形狀(寬度、高度、3)的圖像組成的標准數據集,我通常會執行以下操作:
aug = tf.keras.preprocessing.image.ImageDataGenerator(
rotation_range=15,
zoom_range=0.15)
model.fit_generator(
aug.flow(X_train, y_train),
epochs=100)
如何將這些相同的數據增強應用於表示圖像序列的 4D numpy arrays 數據集?
我想到了。 我創建了一個自定義 class,它繼承自 tensorflow.keras.utils.Sequence,它使用 Z10EAZ440D34CD35AC02 為每個圖像執行增強。
class CustomDataset(tf.keras.utils.Sequence):
def __init__(self, batch_size, *args, **kwargs):
self.batch_size = batch_size
self.X_train = args[0]
self.Y_train = args[1]
def __len__(self):
# returns the number of batches
return int(self.X_train.shape[0] / self.batch_size)
def __getitem__(self, index):
# returns one batch
X = []
y = []
for i in range(self.batch_size):
r = random.randint(0, self.X_train.shape[0] - 1)
next_x = self.X_train[r]
next_y = self.Y_train[r]
augmented_next_x = []
###
### Augmentation parameters for this clip.
###
rotation_amt = random.randint(-45, 45)
for j in range(self.X_train.shape[1]):
transformed_img = ndimage.rotate(next_x[j], rotation_amt, reshape=False)
transformed_img[transformed_img == 0] = 255
augmented_next_x.append(transformed_img)
X.append(augmented_next_x)
y.append(next_y)
X = np.array(X).astype('uint8')
y = np.array(y)
encoder = LabelBinarizer()
y = encoder.fit_transform(y)
return X, y
def on_epoch_end(self):
# option method to run some logic at the end of each epoch: e.g. reshuffling
pass
然后我將它傳遞給fit_generator
方法:
training_data_augmentation = CustomDataset(BS, X_train_L, y_train_L)
model.fit_generator(
training_data_augmentation,
epochs=300)
聲明:本站的技術帖子網頁,遵循CC BY-SA 4.0協議,如果您需要轉載,請注明本站網址或者原文地址。任何問題請咨詢:yoyou2525@163.com.