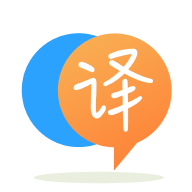
[英]Sums of Column A values for contigious same-value boolean values of separate column
[英]different aggregated sums of the same column based on boolean values in another column
我有一個 dataframe 記錄每個樂高套裝盒中包含的不同樂高積木。 對於每個套裝盒,總是有許多不同的常規件,但有時盒子還包含一些額外的備用件。 所以 dataframe 有一個 boolean 列來區分這種情況。
現在我想總結數據集,所以我只得到每個樂高集(groupby set_id)的一行,其中一個新列是該集框中的總數量(“數量”的總和)。
我的問題是,我還想要兩個額外的列來計算這些部分中有多少是“常規的”,有多少是“備用的”,基於 True/False 列。
有沒有辦法通過創建一個額外的 dataframe 和一個.agg() 調用來計算這三個總和列?
而不是創建 3 個數據框和合並列,這是我目前的方法:
import pandas as pd
import random
random.seed(1)
# creating sample data:
nrows=15
df = pd.DataFrame([], columns=["set_id","part_id","quantity","is_spare"])
df["set_id"]=["ABC"[random.randint(0,2)] for r in range(0,nrows)]
df["part_id"] = [random.randint(1000,8000) for n in range(0,nrows)]
df["quantity"] = [random.randint(1,10) for n in range(0,nrows)]
df["is_spare"]=[random.random()>0.75 for r in range(0,nrows)]
print(df)
# grouping into a new dfsummary dataframe: HOW TO DO IT IN JUST ONE STEP ?
# aggregate sum of ALL pieces:
dfsummary = df.groupby("set_id", as_index=False) \
.agg(num_pieces=("quantity","sum"))
# aggregate sum of "normal" pieces:
dfsummary2 = df.loc[df["is_spare"]==False].groupby("set_id", as_index=False) \
.agg(normal_pieces=("quantity","sum"))
# aggregate sum of "spare" pieces:
dfsummary3 = df.loc[df["is_spare"]==True].groupby("set_id", as_index=False) \
.agg(spare_pieces=("quantity","sum"))
# Putting all aggregate columns together:
dfsummary = dfsummary \
.merge(dfsummary2,on="set_id",how="left") \
.merge(dfsummary3,on="set_id",how="left")
print(dfsummary)
set_id part_id quantity is_spare
0 A 4545 1 False
1 C 5976 1 False
2 A 7244 9 False
3 B 7284 1 False
4 A 1017 7 False
5 B 6700 4 True
6 B 4648 7 False
7 B 3181 1 False
8 C 6910 9 False
9 B 7568 4 True
10 A 2874 8 True
11 A 5842 8 False
12 B 1837 9 False
13 A 3600 4 False
14 B 1250 6 False
set_id num_pieces normal_pieces spare_pieces
0 A 37 29 8.0
1 B 32 24 8.0
2 C 10 10 NaN
我看到了這個Stackoverflow question ,但我的情況有些不同,因為 sum() 函數只會應用於目標列的某些行,具體取決於其他列的 True/False 值。
您可以在一行中完成。 訣竅是創建一個臨時列,其中的數量對於spare_pieces
為負,對於正常件為normal_pieces
:
out = df.assign(qty=df['is_spare'].replace({True: -1, False: 1}) * df['quantity']) \
.groupby('set_id')['qty'] \
.agg(num_pieces=lambda x: sum(abs(x)),
normal_pieces=lambda x: sum(x[x > 0]),
sparse_pieces=lambda x: abs(sum(x[x < 0]))) \
.reset_index()
Output:
>>> out
set_id num_pieces normal_pieces sparse_pieces
0 A 37 29 8
1 B 32 24 8
2 C 10 10 0
>>> df['is_spare'].replace({True: -1, False: 1}) * df['quantity'])
0 1 # normal_pieces
1 1
2 9
3 1
4 7
5 -4 # spare_pieces
6 7
7 1
8 9
9 -4
10 -8
11 8
12 9
13 4
14 6
dtype: int64
一種選擇是進行 groupby 和 unstack:
(df
.groupby(['set_id', 'is_spare'])
.quantity
.sum()
.unstack('is_spare')
.rename(columns={False:'normal_pieces', True:'spare_pieces'})
.assign(num_pieces = lambda df: df.sum(axis = 'columns'))
.rename_axis(columns=None)
.reset_index()
)
set_id normal_pieces spare_pieces num_pieces
0 A 29.0 8.0 37.0
1 B 24.0 8.0 32.0
2 C 10.0 NaN 10.0
聲明:本站的技術帖子網頁,遵循CC BY-SA 4.0協議,如果您需要轉載,請注明本站網址或者原文地址。任何問題請咨詢:yoyou2525@163.com.