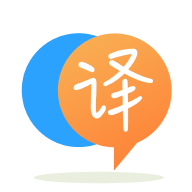
[英]Apply function row wise on pandas data frame on columns with numerical values
[英]Apply function to sets of columns in pandas, 'looping' over entire data frame column-wise
这是一个测试示例,以显示我想要实现的目标。 这是一个玩具数据框:
df = pd.DataFrame(np.random.randn(10,7),index=range(1,11),columns=headers)
这使
Time A_x A_y A_z B_x B_y B_z
1 -0.075509 -0.123527 -0.547239 -0.453707 -0.969796 0.248761 1.369613
2 -0.206369 -0.112098 -1.122609 0.218538 -0.878985 0.566872 -1.048862
3 -0.194552 0.818276 -1.563931 0.097377 1.641384 -0.766217 -1.482096
4 0.502731 0.766515 -0.650482 -0.087203 -0.089075 0.443969 0.354747
5 1.411380 -2.419204 -0.882383 0.005204 -0.204358 -0.999242 -0.395236
6 1.036695 1.115630 0.081825 -1.038442 0.515798 -0.060016 2.669702
7 0.392943 0.226386 0.039879 0.732611 -0.073447 1.164285 1.034357
8 -1.253264 0.389148 0.158289 0.440282 -1.195860 0.872064 0.906377
9 -0.133580 -0.308314 -0.839347 -0.517989 0.652120 0.477232 -0.391767
10 0.623841 0.473552 0.059428 0.726088 -0.593291 -3.186297 -0.846863
我想要做的只是在这种情况下,为每个索引计算每个标题(A和B)的向量长度,并除以Time
列。 因此,该函数需要是np.sqrt(A_x^2 + A_y^2 + A_z^2)
,当然对于B也是如此。 即我想要计算每一行的速度,但三列有助于一个速度结果。
我已经尝试使用df.groupby
和df.filter
来循环遍历列,但我无法让它工作,因为我完全不确定如何将相同的函数有效地应用于数据框的块,所有这些都在一个去(显然一个是避免在行上循环)。 我试过了
df = df.apply(lambda x: np.sqrt(x.dot(x)), axis=1)
这当然有效,但是只有当输入数据框具有正确的列数(3)时,如果更长,那么在整个行上计算点积,而不是在我想要的三列的块中(因为这是转弯对应于标签坐标,这是三维的)。
所以这就是我最终试图用上面的例子得到的(下面的数组只是填充了随机数,而不是我想要计算的实际速度 - 只是为了显示我想要实现的形状):
Velocity_A Velocity_B
1 -0.975633 -2.669544
2 0.766405 -0.264904
3 0.425481 -0.429894
4 -0.437316 0.954006
5 1.073352 -1.475964
6 -0.647534 0.937035
7 0.082517 0.438112
8 -0.387111 -1.417930
9 -0.111011 1.068530
10 0.451979 -0.053333
我的实际数据是50,000 x 36(因此有12个带有x,y,z坐标的标签),我想一次性计算速度以避免迭代(如果可能的话)。 还有一个相同长度的时间列(50,000x1)。
你怎么做到这一点?
谢谢,阿斯特丽德
一个可能的开始。
过滤掉与特定向量对应的列名。 例如
In [20]: filter(lambda x: x.startswith("A_"),df.columns)
Out[20]: ['A_x', 'A_y', 'A_z']
从DataFrame中选择这些列
In [22]: df[filter(lambda x: x.startswith("A_"),df.columns)]
Out[22]:
A_x A_y A_z
1 -0.123527 -0.547239 -0.453707
2 -0.112098 -1.122609 0.218538
3 0.818276 -1.563931 0.097377
4 0.766515 -0.650482 -0.087203
5 -2.419204 -0.882383 0.005204
6 1.115630 0.081825 -1.038442
7 0.226386 0.039879 0.732611
8 0.389148 0.158289 0.440282
9 -0.308314 -0.839347 -0.517989
10 0.473552 0.059428 0.726088
因此,使用此技术,您可以获得3列的块。 例如。
column_initials = ["A","B"]
for column_initial in column_initials:
df["Velocity_"+column_initial]=df[filter(lambda x: x.startswith(column_initial+"_"),df.columns)].apply(lambda x: np.sqrt(x.dot(x)), axis=1)/df.Time
In [32]: df[['Velocity_A','Velocity_B']]
Out[32]:
Velocity_A Velocity_B
1 -9.555311 -22.467965
2 -5.568487 -7.177625
3 -9.086257 -12.030091
4 2.007230 1.144208
5 1.824531 0.775006
6 1.472305 2.623467
7 1.954044 3.967796
8 -0.485576 -1.384815
9 -7.736036 -6.722931
10 1.392823 5.369757
我没有得到与你相同的答案。 但是,我借用你的df.apply(lambda x: np.sqrt(x.dot(x)), axis=1)
并假设它是正确的。
希望这可以帮助。
你的计算比Panda-ish更多NumPy-ish,我的意思是如果你认为你的DataFrame只是一个大数组,计算可以用一些简洁的方式表达,而解决方案(至少我提出的那个)更复杂当你尝试使用melt,groupby等来争论DataFrame时
整个计算可以基本上用一行表示:
np.sqrt((arr**2).reshape(arr.shape[0],-1,3).sum(axis=-1))/times[:,None]
所以这是NumPy方式:
import numpy as np
import pandas as pd
import io
content = '''
Time A_x A_y A_z B_x B_y B_z
-0.075509 -0.123527 -0.547239 -0.453707 -0.969796 0.248761 1.369613
-0.206369 -0.112098 -1.122609 0.218538 -0.878985 0.566872 -1.048862
-0.194552 0.818276 -1.563931 0.097377 1.641384 -0.766217 -1.482096
0.502731 0.766515 -0.650482 -0.087203 -0.089075 0.443969 0.354747
1.411380 -2.419204 -0.882383 0.005204 -0.204358 -0.999242 -0.395236
1.036695 1.115630 0.081825 -1.038442 0.515798 -0.060016 2.669702
0.392943 0.226386 0.039879 0.732611 -0.073447 1.164285 1.034357
-1.253264 0.389148 0.158289 0.440282 -1.195860 0.872064 0.906377
-0.133580 -0.308314 -0.839347 -0.517989 0.652120 0.477232 -0.391767
0.623841 0.473552 0.059428 0.726088 -0.593291 -3.186297 -0.846863'''
df = pd.read_table(io.BytesIO(content), sep='\s+', header=True)
arr = df.values
times = arr[:,0]
arr = arr[:,1:]
result = np.sqrt((arr**2).reshape(arr.shape[0],-1,3).sum(axis=-1))/times[:,None]
result = pd.DataFrame(result, columns=['Velocity_%s'%(x,) for x in list('AB')])
print(result)
产量
Velocity_A Velocity_B
0 -9.555311 -22.467965
1 -5.568487 -7.177625
2 -9.086257 -12.030091
3 2.007230 1.144208
4 1.824531 0.775006
5 1.472305 2.623467
6 1.954044 3.967796
7 -0.485576 -1.384815
8 -7.736036 -6.722931
9 1.392823 5.369757
由于您的实际DataFrame具有形状(50000,36),因此选择快速方法可能很重要。 这是一个基准:
import numpy as np
import pandas as pd
import string
N = 12
col_ids = string.letters[:N]
df = pd.DataFrame(
np.random.randn(50000, 3*N+1),
columns=['Time']+['{}_{}'.format(letter, coord) for letter in col_ids
for coord in list('xyz')])
def using_numpy(df):
arr = df.values
times = arr[:,0]
arr = arr[:,1:]
result = np.sqrt((arr**2).reshape(arr.shape[0],-1,3).sum(axis=-1))/times[:,None]
result = pd.DataFrame(result, columns=['Velocity_%s'%(x,) for x in col_ids])
return result
def using_loop(df):
results = pd.DataFrame(index=df.index) # the result container
for id in col_ids:
results['Velocity_'+id] = np.sqrt((df.filter(regex=id+'_')**2).sum(axis=1))/df.Time
return results
使用IPython :
In [43]: %timeit using_numpy(df)
10 loops, best of 3: 34.7 ms per loop
In [44]: %timeit using_loop(df)
10 loops, best of 3: 82 ms per loop
我至少会对标记标识符进行循环,但不要担心,这是一个非常快速的循环,只是确定过滤模式以获得正确的列:
df = pd.DataFrame(np.random.randn(10,7), index=range(1,11), columns='Time A_x A_y A_z B_x B_y B_z'.split())
col_ids = ['A', 'B'] # I guess you can create that one easily
results = pd.DataFrame(index=df.index) # the result container
for id in col_ids:
results['Velocity_'+id] = np.sqrt((df.filter(regex=id+'_')**2).sum(axis=1))/df.Time
一条班轮......分成许多行以便于阅读:
import numpy as np
import pandas as pd
np.random.seed(0)
df = pd.DataFrame(
np.random.randn(10,7),
index=range(1,11),
columns='Time A_x A_y A_z B_x B_y B_z'.split()
)
result = df\
.loc[:, df.columns.values!='Time']\
.T\
.groupby(lambda x: x[0])\
.apply(lambda x: np.sqrt((x ** 2).sum()))\
.T\
.apply(lambda x: x / df['Time'])
print result
A B
1 1.404626 1.310639
2 -2.954644 -10.874091
3 3.479836 6.105961
4 3.885530 2.244544
5 0.995012 1.434228
6 11.278208 11.454466
7 -1.209242 -1.281165
8 -5.175911 -5.905070
9 11.889318 16.758958
10 -0.978014 -0.590767
注意:我有点沮丧,我需要抛出两个转置。 我只是不能得到groupby
并apply
与axis=1
很好地玩。 如果有人能告诉我如何做到这一点,我将非常感激。 这里的技巧是知道当你调用groupby(lambda x: f(x))
x
是每行索引的值。 因此groupby(lambda x: x[0])
按行索引的第一个字母分组。 换位后,这是A
或B
好的,没有更多的转座:
result = df\
.loc[:, df.columns!='Time']\
.groupby(lambda x: x[0], axis=1)\
.apply(lambda x: np.sqrt((x**2).sum(1)))\
.apply(lambda x: x / df['Time'])
print result
A B
1 1.404626 1.310639
2 -2.954644 -10.874091
3 3.479836 6.105961
4 3.885530 2.244544
5 0.995012 1.434228
6 11.278208 11.454466
7 -1.209242 -1.281165
8 -5.175911 -5.905070
9 11.889318 16.758958
10 -0.978014 -0.590767
声明:本站的技术帖子网页,遵循CC BY-SA 4.0协议,如果您需要转载,请注明本站网址或者原文地址。任何问题请咨询:yoyou2525@163.com.