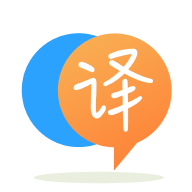
[英]How to get the percentage of each value in a row basis row total in python
[英]pandas: how to get the percentage for each row
当我使用 pandas value_count
方法时,我得到以下数据:
new_df['mark'].value_counts()
1 1349110
2 1606640
3 175629
4 790062
5 330978
我怎样才能得到这样的每一行的百分比?
1 1349110 31.7%
2 1606640 37.8%
3 175629 4.1%
4 790062 18.6%
5 330978 7.8%
我需要将每一行除以这些数据的总和。
np.random.seed([3,1415])
s = pd.Series(np.random.choice(list('ABCDEFGHIJ'), 1000, p=np.arange(1, 11) / 55.))
s.value_counts()
I 176
J 167
H 136
F 128
G 111
E 85
D 83
C 52
B 38
A 24
dtype: int64
百分比
s.value_counts(normalize=True)
I 0.176
J 0.167
H 0.136
F 0.128
G 0.111
E 0.085
D 0.083
C 0.052
B 0.038
A 0.024
dtype: float64
counts = s.value_counts()
percent = counts / counts.sum()
fmt = '{:.1%}'.format
pd.DataFrame({'counts': counts, 'per': percent.map(fmt)})
counts per
I 176 17.6%
J 167 16.7%
H 136 13.6%
F 128 12.8%
G 111 11.1%
E 85 8.5%
D 83 8.3%
C 52 5.2%
B 38 3.8%
A 24 2.4%
我认为你需要:
#if output is Series, convert it to DataFrame
df = df.rename('a').to_frame()
df['per'] = (df.a * 100 / df.a.sum()).round(1).astype(str) + '%'
print (df)
a per
1 1349110 31.7%
2 1606640 37.8%
3 175629 4.1%
4 790062 18.6%
5 330978 7.8%
时间:
使用sum
作为value_counts
两倍似乎更快:
In [184]: %timeit (jez(s))
10 loops, best of 3: 38.9 ms per loop
In [185]: %timeit (pir(s))
10 loops, best of 3: 76 ms per loop
计时代码:
np.random.seed([3,1415])
s = pd.Series(np.random.choice(list('ABCDEFGHIJ'), 1000, p=np.arange(1, 11) / 55.))
s = pd.concat([s]*1000)#.reset_index(drop=True)
def jez(s):
df = s.value_counts()
df = df.rename('a').to_frame()
df['per'] = (df.a * 100 / df.a.sum()).round(1).astype(str) + '%'
return df
def pir(s):
return pd.DataFrame({'a':s.value_counts(),
'per':s.value_counts(normalize=True).mul(100).round(1).astype(str) + '%'})
print (jez(s))
print (pir(s))
这是一个更pythonic的片段,我认为
def aspercent(column,decimals=2):
assert decimals >= 0
return (round(column*100,decimals).astype(str) + "%")
aspercent(df['mark'].value_counts(normalize=True),decimals=1)
这将输出:
1 1349110 31.7%
2 1606640 37.8%
3 175629 4.1%
4 790062 18.6%
5 330978 7.8%
这也允许调整小数位数
创建两个系列,第一个带有绝对值,第二个带有百分比,并将它们连接起来:
import pandas
d = {'mark': ['pos', 'pos', 'pos', 'pos', 'pos',
'neg', 'neg', 'neg', 'neg',
'neutral', 'neutral' ]}
df = pd.DataFrame(d)
absolute = df['mark'].value_counts(normalize=False)
absolute.name = 'value'
percentage = df['mark'].value_counts(normalize=True)
percentage.name = '%'
percentage = (percentage*100).round(2)
pd.concat([absolute, percentage], axis=1)
Output:
value %
pos 5 45.45
neg 4 36.36
neutral 2 18.18
声明:本站的技术帖子网页,遵循CC BY-SA 4.0协议,如果您需要转载,请注明本站网址或者原文地址。任何问题请咨询:yoyou2525@163.com.