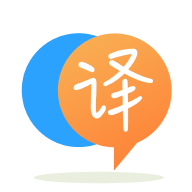
[英]Difference between elements of subsequent pairs in numpy arrays (not numpy.diff)
[英]Vectorizing an operation between all pairs of elements in two numpy arrays
给定两个数组,其中每一行代表一个圆(x,y,r):
data = {}
data[1] = np.array([[455.108, 97.0478, 0.0122453333],
[403.775, 170.558, 0.0138770952],
[255.383, 363.815, 0.0179857619]])
data[2] = np.array([[455.103, 97.0473, 0.012041],
[210.19, 326.958, 0.0156912857],
[455.106, 97.049, 0.0150472381]])
我想抽出所有不相交的圆圈。 这可以通过以下方式完成:
close_data = {}
for row1 in data[1]: #loop over first array
for row2 in data[2]: #loop over second array
condition = ((abs(row1[0]-row2[0]) + abs(row1[1]-row2[1])) < (row1[2]+row2[2]))
if condition: #circles overlap if true
if tuple(row1) not in close_data.keys():
close_data[tuple(row1)] = [row1, row2] #pull out close data points
else:
close_data[tuple(row1)].append(row2)
for k, v in close_data.iteritems():
print k, v
#desired outcome
#(455.108, 97.047799999999995, 0.012245333299999999)
#[array([ 4.55108000e+02, 9.70478000e+01, 1.22453333e-02]),
# array([ 4.55103000e+02, 9.70473000e+01, 1.2040000e-02]),
# array([ 4.55106000e+02, 9.70490000e+01, 1.50472381e-02])]
但是,对于大型数据集,数组上的多个循环效率很低。 是否可以对计算进行向量化,以便获得使用numpy的优势?
实际上,最困难的是获取信息的表示形式。 哦,我插入了几个方块。 如果您确实不想要欧几里德距离,则必须改回来。
import numpy as np
data = {}
data[1] = np.array([[455.108, 97.0478, 0.0122453333],
[403.775, 170.558, 0.0138770952],
[255.383, 363.815, 0.0179857619]])
data[2] = np.array([[455.103, 97.0473, 0.012041],
[210.19, 326.958, 0.0156912857],
[455.106, 97.049, 0.0150472381]])
d1 = data[1][:, None, :]
d2 = data[2][None, :, :]
dists2 = ((d1[..., :2] - d2[..., :2])**2).sum(axis = -1)
radss2 = (d1[..., 2] + d2[..., 2])**2
inds1, inds2 = np.where(dists2 <= radss2)
# translate to your representation:
bnds = np.r_[np.searchsorted(inds1, np.arange(3)), len(inds1)]
rows = [data[2][inds2[bnds[i]:bnds[i+1]]] for i in range(3)]
out = dict([(tuple (data[1][i]), rows[i]) for i in range(3) if rows[i].size > 0])
这是一种纯粹的numpythonic方式( a
是data[1]
和b
是data[2]
):
In [80]: p = np.arange(3) # for creating the indices of combinations using np.tile and np.repeat
In [81]: a = a[np.repeat(p, 3)] # creates the first column of combination array
In [82]: b = b[np.tile(p, 3)] # creates the second column of combination array
In [83]: abs(a[:, :2] - b[:, :2]).sum(1) < a[:, 2] + b[:, 2]
Out[83]: array([ True, False, True, True, False, True, True, False, True], dtype=bool)
声明:本站的技术帖子网页,遵循CC BY-SA 4.0协议,如果您需要转载,请注明本站网址或者原文地址。任何问题请咨询:yoyou2525@163.com.