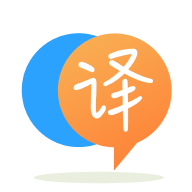
[英]Python pandas dataframe: Find last occurrence of value less-than-or-equal-to current row
[英]Python Pandas - Minutes since last occurrence in 2 million row dataframe
举个例子,我有以下数据帧:
Date indicator_1 indicator_2
2013-04-01 03:50:00 x w
2013-04-01 04:00:00 y u
2013-04-01 04:15:00 z v
2013-04-01 04:25:00 x w
2013-04-01 04:25:00 z u
2013-04-01 04:30:00 y u
2013-04-01 04:35:00 y w
2013-04-01 04:40:00 z w
2013-04-01 04:40:00 x u
2013-04-01 04:40:00 y v
2013-04-01 04:50:00 x w
我的目标是使用以下规则创建两列:
第一列应该给出自indicator_1列上最后一次出现'x'以来的分钟数。
第二列应该给出自指标_1上的“y”和“指标_2”列上的“u”最后一次出现以来的分钟数。
对于具有相同精确小时且其中一个时间对应于'x'(在第一种情况下)或对'y','u'(在第二种情况下)中的行,计算分钟数应该针对先前出现的变量做出。 因此,所需的输出应该是这样的:
Date desired_column_1 desired_column_2 indicator_1 indicator_2
2013-04-01 03:50:00 NaN NaN x w
2013-04-01 04:00:00 10.0 NaN y u
2013-04-01 04:15:00 25.0 15.0 z v
2013-04-01 04:25:00 35.0 25.0 x w
2013-04-01 04:25:00 35.0 25.0 z u
2013-04-01 04:30:00 5.0 30.0 y u
2013-04-01 04:35:00 10.0 5.0 y w
2013-04-01 04:40:00 15.0 10.0 z w
2013-04-01 04:40:00 15.0 10.0 x u
2013-04-01 04:40:00 15.0 10.0 y v
2013-04-01 04:50:00 10.0 20.0 x w
主要问题是整个数据帧有超过200万行,因此使用循环太耗时。 有没有办法实现这个问题的矢量化方法?
数据帧的python代码如下:
d = {'Date': ['2013-04-01 03:50:00','2013-04-01 04:00:00','2013-04-01
04:15:00','2013-04-01 04:25:00','2013-04-01 04:25:00',
'2013-04-01 04:30:00','2013-04-01 04:35:00','2013-04-01 04:40:00','2013-04-01 04:40:00','2013-04-01 04:40:00',
'2013-04-01 04:50:00'], 'indicator_1': ['x','y','z','x','z','y','y','z','x','y','x'],
'indicator_2': ['w','u','v','w','u','u','w','w','u','v','w'],
'desired_column_1': [np.nan, 10, 25, 35, 35,5,10,15,15,15,10],
'desired_column_2': [np.nan, np.nan, 15, 25, 25,30,5,10,10,10,20]}
df = pd.DataFrame(data=d)
首先确保列['Date']
是一个datetime对象,并获取一列来表示行与行之间的时间差
df.Date = pd.to_datetime(df.Date)
df['minD'] = (df.Date -df.Date.shift(1)).astype('timedelta64[m]')
接下来为您的条件创建分组键。 我们向下移动一行,因为我们正在寻找自上一个x以来的时间,这也包括下一个x值。 如果没有转移,我们就不会在我们的小组中包含下一个x。
mask2 = (df.indicator_1.str.cat(df.indicator_2) == 'yu').cumsum().shift(1)
mask1 = (df.indicator_1 == 'x').cumsum().shift(1)
现在通过mask和cumsum()
微小差异,但是我们需要过滤出布尔值的cumsum()
<1,因为条件还没有发生,因此应该有时间差的缺失值。
df['desired_column_1'] = df.groupby(mask1.where(mask1 > 0)).minD.cumsum()
df['desired_column_2'] = df.groupby(mask2.where(mask2 > 0)).minD.cumsum()
现在,您可以通过向前填充数据来替换这些列中的0值
df.desired_column_1 = df.desired_column_1.replace(0,method='ffill')
df.desired_column_2 = df.desired_column_2.replace(0,method='ffill')
这个
Date indicator_1 indicator_2 desired_column_1 \
0 2013-04-01 03:50:00 x w NaN
1 2013-04-01 04:00:00 y u 10.0
2 2013-04-01 04:15:00 z v 25.0
3 2013-04-01 04:25:00 x w 35.0
4 2013-04-01 04:25:00 z u 35.0
5 2013-04-01 04:30:00 y u 5.0
6 2013-04-01 04:35:00 y w 10.0
7 2013-04-01 04:40:00 z w 15.0
8 2013-04-01 04:40:00 x u 15.0
9 2013-04-01 04:40:00 y v 15.0
10 2013-04-01 04:50:00 x w 10.0
desired_column_2
0 NaN
1 NaN
2 15.0
3 25.0
4 25.0
5 30.0
6 5.0
7 10.0
8 10.0
df = df.loc[:, ['Date', 'indicator_1', 'indicator_2']]
idx = df.index
df['Date'] = df['Date'].apply(pd.to_datetime)
# Sort by column indicator_1 for using df.diff()
df.sort_values(['indicator_1'], inplace=True)
df['diffs1'] = df['Date'].diff()
# Shift 1 then compare with original to get the line that value changes (from x to y for init)
mask = df.indicator_1 != df.indicator_1.shift(1)
df['diffs1'][mask] = np.nan
df.reindex(idx)
# Same for case 2
df['indicator_3'] = df['indicator_1'] + df['indicator_2']
df.sort_values(['indicator_3'], inplace=True)
df['diffs2'] = df['Date'].diff()
mask = df.indicator_3 != df.indicator_3.shift(1)
df['diffs2'][mask] = np.nan
df.reindex(idx)
声明:本站的技术帖子网页,遵循CC BY-SA 4.0协议,如果您需要转载,请注明本站网址或者原文地址。任何问题请咨询:yoyou2525@163.com.