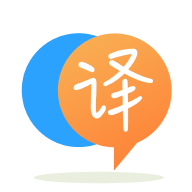
[英]How do I fix an InvalidArgument error when trying to train a TensorFlow model?
[英]How do I fix a “Number of features error” when trying to test against my random forrest model?
我有一个训练有素的模型。 我想找出新数据属于哪个类。 我做了一些试验,但我遇到了一些问题。
with open('text_classifier', 'rb') as training_model:
model = pickle.load(training_model)
y_pred2 = model.predict(X_test)
此代码有效
但...
new_test_data=["spor toto süper lig 30. hafta medipol bu akşam ev göztepe
ile saat 20.30'da başla mücadele suat arslanboğa arslanboğa yardımcı
serka ok ve ismail şencan"]
tfidfconverter = TfidfVectorizer()
new_test_data = tfidfconverter.fit_transform(new_test_data).toarray()
model.predict(new_test_data)
我收到这样的错误
Number of features of the model must match the input. Model n_features is 9671 and input n_features is 25
我正在训练的代码块
data = load_files(r"...\docs",encoding="utf-8")
X, y = data.data, data.target
tfidfconverter = TfidfVectorizer(min_df=3, max_df=0.7)
X = tfidfconverter.fit_transform(X).toarray()
X_train, X_test, y_train, y_test = train_test_split(X, y, test_size=0.2,
random_state=0)
classifier = RandomForestClassifier(n_estimators=1000, random_state=0)
classifier.fit(X_train, y_train)
y_pred2 = classifier.predict(X_test)
我相信在训练模型时,您需要在数据中指定要实际用作特征的参数。 看起来您的训练模型使用行条目作为特征而不是每一列。 这可以通过读入数据,然后将其转换为 CSV,然后再次读入来解决。 但是,如果您已经知道数据的结构,则不需要此步骤。 基本上,您只需要知道数据列的名称。 此方法需要 Pandas 模块。 这是一些代码...
data = load_files(r"...\docs",encoding="utf-8")
data.to_csv('train_data.csv', encoding = 'utf-8', index = False)
然后从 CSV 读回训练数据......
train_data = pd.read_csv('train_data.csv')
现在,当您调用 train_test_split 方法时,您应该指定要用作数据中的特征的内容。 这通常是数据表中的列,因为这些是收集来分析的指标。 我定义了函数来拆分数据并构建指定特征的模型,因为我认为它更容易理解,但您也可以直接调用函数。
def split_dataset(dataset, train_percentage, feature_headers, target_header):
train_x, test_x, train_y, test_y = train_test_split(dataset[feature_headers],
dataset[target_header], train_size = train_percentage)
return train_x, test_x, train_y, test_y
def random_forest_classifier(features, target):
model = RandomForestClassifier(n_estimators = 500, oob_score = True, n_jobs
=-1,random_state = 1, min_impurity_decrease = .01)
model.fit(features, target)
return model
现在您已准备好使用您的数据调用函数。
train_x, test_x, train_y, test_y = split_dataset(train_data, 0.80,
train_data.columns[0:24], train_data.columns[-1])
trained_model = random_forest_classifier(train_x,train_y)
您现在应该能够使用 25 个特征针对您的训练模型进行预测。
声明:本站的技术帖子网页,遵循CC BY-SA 4.0协议,如果您需要转载,请注明本站网址或者原文地址。任何问题请咨询:yoyou2525@163.com.