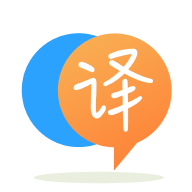
[英]How to use multi-gpu in Keras with shared weights applications model
[英]how to use the Multi GPU _ BERT
我使用以下 BERT 代码对多个 GPU 进行分析。
model = BertForSequenceClassification.from_pretrained(
"beomi/kcbert-large",
num_labels = len(df['label'].unique()),
output_attentions = False,
output_hidden_states = False,
)
model = torch.nn.DataParallel(model)
model.cuda()
用一个 GPU 分析时,分析没有问题。 (无模型 = torch.nn.DataParallel(model))
但是之后
model = torch.nn.DataParallel(model)
有错误
import random
import numpy as np
# This training code is based on the `run_glue.py` script here:
# https://github.com/huggingface/transformers/blob/5bfcd0485ece086ebcbed2d008813037968a9e58/examples/run_glue.py#L128
# Set the seed value all over the place to make this reproducible.
seed_val = 42
random.seed(seed_val)
np.random.seed(seed_val)
torch.manual_seed(seed_val)
torch.cuda.manual_seed_all(seed_val)
training_stats = []
total_t0 = time.time()
for epoch_i in range(0, epochs):
# ========================================
# Training
# ========================================
# Perform one full pass over the training set.
t0 = time.time()
total_train_loss = 0
total_train_accuracy = 0
for step, batch in enumerate(train_dataloader):
if step % 40 == 0 and not step == 0:
elapsed = format_time(time.time() - t0)
print(' Batch {:>5,} of {:>5,}. Elapsed: {:}.'.format(step, len(train_dataloader), elapsed))
b_input_ids = batch[0].to(device)
b_input_mask = batch[1].to(device)
b_labels = batch[2].to(device)
model.zero_grad()
loss, logits = model(b_input_ids,
token_type_ids=None,
attention_mask=b_input_mask,
labels=b_labels)
total_train_loss += loss.item()
logits = logits.detach().cpu().numpy()
label_ids = b_labels.to('cpu').numpy()
total_train_accuracy += flat_accuracy(logits, label_ids)
loss.backward()
torch.nn.utils.clip_grad_norm_(model.parameters(), 1.0)
optimizer.step()
scheduler.step()
avg_train_loss = total_train_loss / len(train_dataloader)
training_time = format_time(time.time() - t0)
avg_train_accuracy = total_train_accuracy / len(train_dataloader)
我遇到了以下问题:ValueError:只有一个元素张量可以转换为 Python 标量
-> total_train_loss += loss.item()
我不知道发生了什么错误,
请帮忙。 谢谢
DataParallel
将返回在您的每个 gpu 上计算的部分损失,因此您可以这样做
loss.backward(torch.Tensor([1, 1]))
或者
loss.sum().backward()
或者
loss.mean().backward()
loss.mean()
要求所有批次大小相等
它们中的任何一个都将帮助您获得缩放值。
声明:本站的技术帖子网页,遵循CC BY-SA 4.0协议,如果您需要转载,请注明本站网址或者原文地址。任何问题请咨询:yoyou2525@163.com.