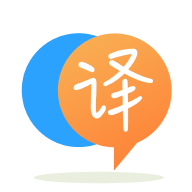
[英]Shapes are incompatible with Keras Functional Model and VGG16 model
[英]Keras 2 input model, incompatible Shapes
我正在尝试使用 keras 构建两个输入 model,每个输入都是一个字符串。
这是 model 的代码
vectorize_layer1 = TextVectorization(split="character", output_sequence_length=512,
max_tokens=MAX_STRING_SIZE)
vectorize_layer1.adapt(list(vocab))
# define two sets of inputs
inputA = Input(shape=(1,), dtype=tf.string)
inputB = Input(shape=(1,), dtype=tf.string)
# the first branch operates on the first input
x = vectorize_layer1(inputA)
x = Embedding(len(vectorize_layer1.get_vocabulary()), MAX_STRING_SIZE)(x)
x = Bidirectional(LSTM(MAX_STRING_SIZE, return_sequences=True, dropout=.2))(x)
x = LSTM(MAX_STRING_SIZE, activation="tanh", return_sequences=False, dropout=.2)(x)
x = Model(inputs=inputA, outputs=x)
# the second branch opreates on the second input
y = vectorize_layer1(inputB)
y = Embedding(len(vectorize_layer1.get_vocabulary()), MAX_STRING_SIZE)(y)
y = Bidirectional(LSTM(MAX_STRING_SIZE, return_sequences=True, dropout=.2))(y)
y = LSTM(MAX_STRING_SIZE, activation="tanh", return_sequences=False, dropout=.2)(y)
y = Model(inputs=inputB, outputs=y)
# combine the output of the two branches
combined = concatenate([x.output, y.output])
# apply a FC layer and then a prediction on the categories
z = Dense(2, activation="relu")(combined)
z = Dense(len(LABELS), activation="softmax")(z)
# our model will accept the inputs of the two branches an
model = Model(inputs=[x.input, y.input], outputs=z)
print(model.predict((np.array(["i love python"]), np.array(["test"]))))
#That prediction works fine!
model.summary()
plot_model(model, to_file="model.png", show_shapes=True, show_layer_names=True)
model.compile(optimizer='Adam',
loss=CategoricalCrossentropy(from_logits=False),
metrics=["categorical_accuracy"])
stopper = EarlyStopping(monitor='val_categorical_accuracy', patience=10)
checkpointer = ModelCheckpoint("model-best.tf", save_best_only=True)
model.fit(
training,
callbacks=[stopper, checkpointer],
steps_per_epoch=2048,
validation_data=validation,
batch_size=8,
epochs=epochs
)
model.save(output, save_format='tf')
我收到了 Shapes are incompatible 错误:
line 5119, in categorical_crossentropy
target.shape.assert_is_compatible_with(output.shape)
ValueError: Shapes (None, 1) and (None, 20) are incompatible
以下是训练/验证数据的示例:
((array(['foo'], dtype='<U6'), array(['bar'], dtype='<U26')), array([0., 0., 1., 0., 0., 0., 0., 0., 0., 0., 0., 0., 0., 0., 0., 0., 0., 0., 0., 0.]))
任何想法出了什么问题? 数据对我来说看起来不错,类别是 1-hot 编码的。
这是堆栈跟踪:
ValueError: in user code:
File "/Users/**/code/venv/lib/python3.10/site-packages/keras/engine/training.py", line 1051, in train_function *
return step_function(self, iterator)
File "/Users/**/code/venv/lib/python3.10/site-packages/keras/engine/training.py", line 1040, in step_function **
outputs = model.distribute_strategy.run(run_step, args=(data,))
File "/Users/**/code/venv/lib/python3.10/site-packages/keras/engine/training.py", line 1030, in run_step **
outputs = model.train_step(data)
File "/Users/**/code/venv/lib/python3.10/site-packages/keras/engine/training.py", line 890, in train_step
loss = self.compute_loss(x, y, y_pred, sample_weight)
File "/Users/**/code/venv/lib/python3.10/site-packages/keras/engine/training.py", line 948, in compute_loss
return self.compiled_loss(
File "/Users/**/code/venv/lib/python3.10/site-packages/keras/engine/compile_utils.py", line 201, in __call__
loss_value = loss_obj(y_t, y_p, sample_weight=sw)
File "/Users/**/code/venv/lib/python3.10/site-packages/keras/losses.py", line 139, in __call__
losses = call_fn(y_true, y_pred)
File "/Users/**/code/venv/lib/python3.10/site-packages/keras/losses.py", line 243, in call **
return ag_fn(y_true, y_pred, **self._fn_kwargs)
File "/Users/**/code/venv/lib/python3.10/site-packages/keras/losses.py", line 1787, in categorical_crossentropy
return backend.categorical_crossentropy(
File "/Users/**/code/venv/lib/python3.10/site-packages/keras/backend.py", line 5119, in categorical_crossentropy
target.shape.assert_is_compatible_with(output.shape)
ValueError: Shapes (None, 1) and (None, 20) are incompatible
我不确定这是问题所在,但它确实表明 categorical_crossentropy 可以 object 到 tensorflow 数据集中的形状张量 (n),正如您似乎拥有的那样,需要一个形状张量 (1,n)
无论如何,作为评论发布太长了,所以我只能将其作为“答案”发布
在下面的代码中,我正在创建一个像您这样的 tf 数据集,其中包含一行和 Y.shape (n)。 categorical_crossentropy 损失不会接受它,但如果 Y.shape 是 (1,6) 则确实有效
(恐怕我不知道如何在不设置 tf.config.run_functions_eagerly(True) 的情况下进行重塑工作,但这对您来说可能不是问题,因为您使用的是生成器)
这是我的最小 model 和数据集设置:
import tensorflow as tf
import numpy as np
tf.config.run_functions_eagerly(True) # tf.autograph objects to something about the reshape
dataset = tf.data.Dataset.from_tensor_slices(((np.array([[0.]]),np.array([[1.]])),np.array([[8., 3., 0., 8., 2., 1.]])))
# Even though the original array had shape (1,6), the dataset element has shape (6)
print(f"dataset y shape: {[row[1].shape for row in dataset]}")
# -- create and compile model, 2 inputs, one output
inp1 = tf.keras.Input(shape=(1,))
inp2 = tf.keras.Input(shape=(1,))
x = tf.concat([inp1, inp2], axis=1)
y = tf.keras.layers.Dense(6)(x)
model = tf.keras.models.Model(inputs=[inp1, inp2], outputs=y)
model.compile(optimizer='Adam', loss='categorical_crossentropy')
然后这失败了,与您的错误类似:
history = model.fit(dataset, epochs=1)
但这成功了,在将 y 从 (6) 重塑为 (1,6) 之后:
def reshaper(x, y):
return x, tf.expand_dims(y, 0)
dataset2 = dataset.map(reshaper)
print(f"dataset2 y shape: {[row[1].shape for row in dataset2]}")
history = model.fit(dataset2, epochs=1)
output 层中的隐藏单元应为 1。
z = Dense(1, activation="softmax")(z)
声明:本站的技术帖子网页,遵循CC BY-SA 4.0协议,如果您需要转载,请注明本站网址或者原文地址。任何问题请咨询:yoyou2525@163.com.